# Copyright 2021 NVIDIA Corporation. All Rights Reserved.
#
# Licensed under the Apache License, Version 2.0 (the "License");
# you may not use this file except in compliance with the License.
# You may obtain a copy of the License at
#
# http://www.apache.org/licenses/LICENSE-2.0
#
# Unless required by applicable law or agreed to in writing, software
# distributed under the License is distributed on an "AS IS" BASIS,
# WITHOUT WARRANTIES OR CONDITIONS OF ANY KIND, either express or implied.
# See the License for the specific language governing permissions and
# limitations under the License.
# ==============================================================================
# Each user is responsible for checking the content of datasets and the
# applicable licenses and determining if suitable for the intended use.
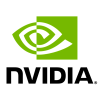
SOK Train DLRM Demo
Overview
This notebook demonstrates how to train a DLRM model with SparseOperationKit (SOK) and then make inference with HierarchicalParameterServer(HPS). It is recommended to run sparse_operation_kit_demo.ipynb and hierarchical_parameter_server_demo.ipynb before diving into this notebook.
For more details about SOK, please refer to SOK Documentation. For more details about HPS APIs, please refer to HPS APIs. For more details about HPS, please refer to HugeCTR Hierarchical Parameter Server (HPS).
Installation
Get SOK from NGC
Both SOK and HPS Python modules are preinstalled in the 24.06 and later Merlin HugeCTR Container: nvcr.io/nvidia/merlin/merlin-hugectr:24.06
.
You can check the existence of the required libraries by running the following Python code after launching this container.
$ python3 -c "import sparse_operation_kit as sok"
$ python3 -c "import hierarchical_parameter_server as hps"
Configurations
First of all we specify the required configurations, e.g., the arguments needed for generating the dataset, the model parameters and the paths to save the model. We will use DLRM model which has one embedding table, bottom MLP layers, interaction layer and top MLP layers. Please note that the input to the embedding layer will be a sparse key tensor.
import sys
import os
os.environ['TF_CPP_MIN_LOG_LEVEL'] = '3'
import numpy as np
import tensorflow as tf
import horovod.tensorflow as hvd
import sparse_operation_kit as sok
import struct
args = dict()
args["gpu_num"] = 2 # the number of available GPUs
args["iter_num"] = 10 # the number of training iteration
args["slot_num"] = 26 # the number of feature fields in this embedding layer
args["embed_vec_sizes"] = [16]*args["slot_num"] # the dimension of embedding vectors
args["dense_dim"] = 13 # the dimension of dense features
args["global_batch_size"] = 1024 # the globally batchsize for all GPUs
args["local_batch_size"] = int(args["global_batch_size"]/args["gpu_num"]) # the locally batchsize for all GPUs
args["table_names"] = ["table"+str(i) for i in range(args["slot_num"])] # embedding table names
args["max_vocabulary_sizes"] = np.random.randint(1000, 1200, size=args["slot_num"]).tolist()
args["max_nnz"] = np.random.randint(1, 100, size=args["slot_num"])
args["combiner"] = ["mean"]*args["slot_num"]
args["sok_backend_type"] = "hybrid" # selcet sok backend type , hybrid means use HKV, hbm means use DET
args["ps_config_file"] = "dlrm.json"
args["dense_model_path"] = "dlrm_dense.model"
args["sparse_model_path"] = "dlrm_sparse.model"
args["sok_embedding_table_path"] = "sok_dlrm_sparse.model"
args["saved_path"] = "dlrm_tf_saved_model"
args["np_key_type"] = np.int64
args["np_vector_type"] = np.float32
args["tf_key_type"] = tf.int64
args["tf_vector_type"] = tf.float32
hvd.init()
gpus = tf.config.experimental.list_physical_devices("GPU")
for gpu in gpus:
tf.config.experimental.set_memory_growth(gpu, True)
if gpus:
tf.config.experimental.set_visible_devices(gpus[hvd.local_rank()], "GPU")
sok.init()
def generate_random_samples(batch_size,iters, vocabulary_range_per_slot, max_nnz, dense_dim):
num_samples = batch_size*iters
def generate_ragged_tensor_samples(embedding_table_sizes,batch_size, lookup_num, hotness, iters):
if len(hotness) != lookup_num:
raise ValueError("Length of hotness list must be equal to lookup_num")
total_indices = []
for i in range(lookup_num):
offsets = np.random.randint(1, hotness[i] + 1, iters * batch_size)
offsets = tf.convert_to_tensor(offsets, dtype=tf.int64)
values = np.random.randint(0, embedding_table_sizes[i], tf.reduce_sum(offsets))
values = tf.convert_to_tensor(values, dtype=tf.int64)
total_indices.append(tf.RaggedTensor.from_row_lengths(values, offsets))
return total_indices
sparse_keys = generate_ragged_tensor_samples(vocabulary_range_per_slot,batch_size,len(vocabulary_range_per_slot),max_nnz,iters)
dense_features = np.random.random((num_samples, dense_dim)).astype(np.float32)
labels = np.random.randint(low=0, high=2, size=(num_samples, 1))
return sparse_keys, dense_features, labels
def tf_dataset(sparse_keys, dense_features, labels, batchsize):
total_data = []
total_data.extend(sparse_keys)
total_data.append(dense_features)
total_data.append(labels)
dataset = tf.data.Dataset.from_tensor_slices(tuple(total_data))
dataset = dataset.batch(batchsize, drop_remainder=True)
return dataset
Build model with SOK embedding layers
We define the model graph for training with SOK embedding variables, i.e., sok.DynamicVariable
and lookup sparse values use sok.lookup_sparse
,e can then train the model and save the trained weights of the embedding table into file system. As for the dense layers, they are saved as a separate model graph, which can be loaded directly during inference.
class MLP(tf.keras.layers.Layer):
def __init__(self,
arch,
activation='relu',
out_activation=None,
**kwargs):
super(MLP, self).__init__(**kwargs)
self.layers = []
index = 0
for units in arch[:-1]:
self.layers.append(tf.keras.layers.Dense(units, activation=activation, name="{}_{}".format(kwargs['name'], index)))
index+=1
self.layers.append(tf.keras.layers.Dense(arch[-1], activation=out_activation, name="{}_{}".format(kwargs['name'], index)))
def call(self, inputs, training=True):
x = self.layers[0](inputs)
for layer in self.layers[1:]:
x = layer(x)
return x
class SecondOrderFeatureInteraction(tf.keras.layers.Layer):
def __init__(self, self_interaction=False):
super(SecondOrderFeatureInteraction, self).__init__()
self.self_interaction = self_interaction
def call(self, inputs):
batch_size = tf.shape(inputs)[0]
num_feas = tf.shape(inputs)[1]
dot_products = tf.matmul(inputs, inputs, transpose_b=True)
ones = tf.ones_like(dot_products)
mask = tf.linalg.band_part(ones, 0, -1)
out_dim = num_feas * (num_feas + 1) // 2
if not self.self_interaction:
mask = mask - tf.linalg.band_part(ones, 0, 0)
out_dim = num_feas * (num_feas - 1) // 2
flat_interactions = tf.reshape(tf.boolean_mask(dot_products, mask), (batch_size, out_dim))
return flat_interactions
class SokEmbLayer(tf.keras.layers.Layer):
def __init__(self,embedding_dims,embedding_table_sizes,var_type,combiners,table_names,name):
super(SokEmbLayer, self).__init__(name=name)
self.table_num = len(embedding_dims)
self.combiners = combiners
self.initializers = ["uniform"]*self.table_num
self.sok_vars = [sok.DynamicVariable(
dimension=embedding_dims[i],
var_type=var_type,
initializer=self.initializers[i],
init_capacity=embedding_table_sizes[i],
max_capacity=embedding_table_sizes[i],
name = table_names[i]
)
for i in range(self.table_num)
]
self.reshape_layer_list = []
for i in range(self.table_num):
self.reshape_layer_list.append(tf.keras.layers.Reshape((1, args["embed_vec_sizes"][i]), name = "sok_reshape"+str(i)))
self.concat1 = tf.keras.layers.Concatenate(axis=1, name = "sok_concat1")
def call(self, inputs):
embeddings = sok.lookup_sparse(self.sok_vars, inputs, combiners=self.combiners)
ret_embeddings = []
for i in range(args["slot_num"]):
ret_embeddings.append(self.reshape_layer_list[i](embeddings[i]))
ret_embeddings = self.concat1(ret_embeddings)
return ret_embeddings
class DLRM(tf.keras.models.Model):
def __init__(self,
combiners,
embedding_table_sizes,
embed_vec_dims,
sok_backend_type,
slot_num,
dense_dim,
arch_bot,
arch_top,
self_interaction,
table_names,
**kwargs):
super(DLRM, self).__init__(**kwargs)
self.combiners = combiners
self.embed_vec_dims = embed_vec_dims
self.sok_backend_type = sok_backend_type
self.embedding_table_sizes = embedding_table_sizes
self.slot_num = len(combiners)
self.dense_dim = dense_dim
self.embedding_model = SokEmbLayer(embedding_dims=self.embed_vec_dims,
embedding_table_sizes = self.embedding_table_sizes,
var_type = self.sok_backend_type,combiners=combiners,table_names = table_names,name="sok_embedding")
self.bot_nn = MLP(arch_bot, name = "bottom", out_activation='relu')
self.top_nn = MLP(arch_top, name = "top", out_activation='sigmoid')
self.interaction_op = SecondOrderFeatureInteraction(self_interaction)
if self_interaction:
self.interaction_out_dim = (self.slot_num+1) * (self.slot_num+2) // 2
else:
self.interaction_out_dim = self.slot_num * (self.slot_num+1) // 2
self.reshape_layer1 = tf.keras.layers.Reshape((1, arch_bot[-1]), name = "dense_reshape1")
self.concat1 = tf.keras.layers.Concatenate(axis=1, name = "dense_concat1")
self.concat2 = tf.keras.layers.Concatenate(axis=1, name = "dense_concat2")
def call(self, inputs, training=True):
input_sparse = inputs[0]
input_dense = inputs[1]
embedding_vectors = self.embedding_model(input_sparse)
dense_x = self.bot_nn(input_dense)
concat_features = self.concat1([embedding_vectors, self.reshape_layer1(dense_x)])
Z = self.interaction_op(embedding_vectors)
z = self.concat2([dense_x, Z])
logit = self.top_nn(z)
return logit, embedding_vectors
def summary(self):
sparse_inputs = []
for i in range(self.slot_num):
sparse_inputs.append(tf.keras.Input(shape=(args["max_nnz"][i], ), sparse=True, dtype=args["tf_key_type"]))
dense_input = tf.keras.Input(shape=(self.dense_dim, ), dtype=tf.float32)
inputs = [sparse_inputs,dense_input]
model = tf.keras.models.Model(inputs=inputs, outputs=self.call(inputs))
return model.summary()
def get_embedding_model(self):
return self.embedding_model
def get_embedding_variables(self):
return self.embedding_model.trainable_variables
def get_dense_variables(self):
tmp_var = self.trainable_variables
sparse_vars , dense_vars = sok.filter_variables(tmp_var)
return dense_vars
def embedding_load(self,path,opt):
embedding_vars = self.get_embedding_variables()
sok.load(path, embedding_vars, opt)
def embedding_dump(self,path,opt):
embedding_vars = self.get_embedding_variables()
sok.dump(path, embedding_vars, opt)
Train with SOK models
Define a Trainer class to wrap the training of SOK. When training SOK, the following points need to be noted: 1.Two gradient tapes need to be defined because the dense variables may need to be wrapped with Horovod’s hvd.DistributedGradientTape. 2. SOK variables need to be updated using SOK’s optimizer, while the dense variables need to be updated using TensorFlow’s optimizer.
class Trainer:
def __init__(self,args):
self.args = args
self.dlrm = DLRM(combiners = args["combiner"],
embedding_table_sizes = args["max_vocabulary_sizes"],
embed_vec_dims = args["embed_vec_sizes"],
sok_backend_type = args["sok_backend_type"],
slot_num = args["slot_num"],
dense_dim = args["dense_dim"],
arch_bot = [256, 128, args["embed_vec_sizes"][0]],
arch_top = [256, 128, 1],
self_interaction = False,
table_names = args["table_names"])
# initialize optimizer
optimizer = tf.optimizers.Adam(learning_rate=1.0)
self.embedding_opt = sok.OptimizerWrapper(optimizer)
self.dense_opt = tf.optimizers.Adam(learning_rate=1.0)
self.loss_fn = tf.keras.losses.BinaryCrossentropy()
def train(self):
embedding_vars = self.dlrm.get_embedding_variables()
dense_vars = self.dlrm.get_dense_variables()
@tf.function
def _train_step(inputs, labels):
with tf.GradientTape() as tape, tf.GradientTape() as emb_tape:
logit, embedding_vector = self.dlrm(inputs, training=True)
loss = self.loss_fn(labels, logit)
tape = hvd.DistributedGradientTape(tape)
dense_grads = tape.gradient(loss, dense_vars)
embedding_grads = emb_tape.gradient(loss, embedding_vars)
self.embedding_opt.apply_gradients(zip(embedding_grads, embedding_vars))
self.dense_opt.apply_gradients(zip(dense_grads, dense_vars))
return logit, embedding_vector, loss
sparse_keys, dense_features, labels = generate_random_samples(self.args["local_batch_size"], self.args["iter_num"], self.args["max_vocabulary_sizes"], self.args["max_nnz"], self.args["dense_dim"])
dataset = tf_dataset(sparse_keys, dense_features, labels, self.args["local_batch_size"])
for i, input_tuple in enumerate(dataset):
sparse_keys = input_tuple[:-2]
dense_features = input_tuple[-2]
labels = input_tuple[-1]
inputs = [sparse_keys, dense_features]
logit, embedding_vector, loss = _train_step(inputs, labels)
print("-"*20, "Step {}, loss: {}".format(i, loss), "-"*20)
self.dlrm.summary()
def dump_model(self):
self.dlrm.embedding_dump(args["sok_embedding_table_path"],self.embedding_opt)
dense_model = tf.keras.Model([self.dlrm.get_layer("sok_embedding").output,
self.dlrm.get_layer("bottom").input],
self.dlrm.get_layer("top").output)
dense_model.summary()
dense_model.save(args["dense_model_path"])
trainer = Trainer(args)
trainer.train()
trainer.dump_model()
!mkdir -p dlrm_sparse.model
embedding_saver.dump_to_file(trained_model.embedding_layer.embedding_variable, args["embedding_table_path"])
!mv dlrm_sparse.model/EmbeddingVariable_keys.file dlrm_sparse.model/key
!mv dlrm_sparse.model/EmbeddingVariable_values.file dlrm_sparse.model/emb_vector
!ls -l dlrm_sparse.model
2022-07-29 07:17:01.079021: I sparse_operation_kit/kit_cc/kit_cc_infra/src/parameters/raw_manager.cc:192] Saving EmbeddingVariable to dlrm_sparse.model..
2022-07-29 07:17:01.079021: I sparse_operation_kit/kit_cc_impl/embedding/common/src/dumping_functions.cc:60] Worker: 0, GPU: 0 key-index count = 260000
2022-07-29 07:17:01.079021: I sparse_operation_kit/kit_cc_impl/embedding/common/src/dumping_functions.cc:147] Worker: 0, GPU: 0: dumping parameters from hashtable..
2022-07-29 07:17:01.079021: I sparse_operation_kit/kit_cc/kit_cc_infra/src/parameters/raw_manager.cc:200] Saved EmbeddingVariable to dlrm_sparse.model.
total 18360
-rw-r--r-- 1 nobody nogroup 16640000 Jul 29 07:17 emb_vector
-rw-r--r-- 1 nobody nogroup 2080000 Jul 29 07:17 key