# Copyright 2021 NVIDIA Corporation. All Rights Reserved.
#
# Licensed under the Apache License, Version 2.0 (the "License");
# you may not use this file except in compliance with the License.
# You may obtain a copy of the License at
#
# http://www.apache.org/licenses/LICENSE-2.0
#
# Unless required by applicable law or agreed to in writing, software
# distributed under the License is distributed on an "AS IS" BASIS,
# WITHOUT WARRANTIES OR CONDITIONS OF ANY KIND, either express or implied.
# See the License for the specific language governing permissions and
# limitations under the License.
# ==============================================================================
# Each user is responsible for checking the content of datasets and the
# applicable licenses and determining if suitable for the intended use.
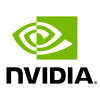
HugeCTR Embedding Collection
About this Notebook
This notebook shows how to use an embedding collection in a DLRM model with the Criteo dataset for training and evaluation.
It shows two key feature usage in embedding collection:
How to configure table place strategy.
How to use dynamic hash table.
Concepts and API Reference
The following key classes are used in this notebook:
hugectr.EmbeddingTableConfig
hugectr.EmbeddingCollectionConfig
For the concepts and API reference information about the classes and file, see the Overview of Using the HugeCTR Embedding Collection in the HugeCTR Layer Classes and Methods information.
Setup
To setup the environment, refer to HugeCTR Example Notebooks and follow the instructions there before running the following.
Use an Embedding Collection with a DLRM Model
Data Preparation
To download and prepare the dataset we will be doing the following steps. At the end of this cell, we provide the shell commands you can run on the terminal to get the data ready for this notebook.
Note: If you already have the data downloaded, then skip to the preprocessing step (2). If preprocessing is also done, skip to creating the softlink between the processed data to the notebooks/
directory (3).
Download the Criteo dataset
To preprocess the downloaded Kaggle Criteo dataset, we’ll make the following operations:
Reduce the amounts of data to speed up the preprocessing
Fill missing values
Remove the feature values whose occurrences are very rare, etc.
Preprocessing by Pandas:
Meanings of the command line arguments:
The 1st argument represents the dataset postfix. It is
1
here sinceday_1
is used.The 2nd argument
wdl_data
is where the preprocessed data is stored.The 3rd argument
pandas
is the processing script going to use, here we choosepandas
.The 4th argument
1
embodies that the normalization is applied to dense features.The 5th argument
1
means that the feature crossing is applied.The 6th argument
100
means the number of data files in each file list.
For more details about the data preprocessing, please refer to the “Preprocess the Criteo Dataset” section of the README in the samples/criteo directory of the repository on GitHub.
Create a soft link of the dataset folder to the path of this notebook
Run the following commands on the terminal to prepare the data for this notebook
export project_root=/home/hugectr # set this to the directory where hugectr is downloaded
cd ${project_root}/tools
# Step 1
wget https://storage.googleapis.com/criteo-cail-datasets/day_0.gz
#Step 2
bash preprocess.sh 0 deepfm_data_nvt nvt 1 0 0
#Step 3
ln -s ${project_root}/tools/deepfm_data_nvt ${project_root}/notebooks/deepfm_data_nvt
Prepare the Training Script
This notebook was developed with on single DGX-1 to run the DLRM model in this notebook. The GPU info in DGX-1 is as follows. It consists of 8 V100-SXM2 GPUs.
! nvidia-smi
Thu Jun 23 00:14:56 2022
+-----------------------------------------------------------------------------+
| NVIDIA-SMI 460.32.03 Driver Version: 460.32.03 CUDA Version: 11.6 |
|-------------------------------+----------------------+----------------------+
| GPU Name Persistence-M| Bus-Id Disp.A | Volatile Uncorr. ECC |
| Fan Temp Perf Pwr:Usage/Cap| Memory-Usage | GPU-Util Compute M. |
| | | MIG M. |
|===============================+======================+======================|
| 0 Tesla V100-SXM2... On | 00000000:06:00.0 Off | 0 |
| N/A 33C P0 42W / 300W | 0MiB / 16160MiB | 0% Default |
| | | N/A |
+-------------------------------+----------------------+----------------------+
| 1 Tesla V100-SXM2... On | 00000000:07:00.0 Off | 0 |
| N/A 35C P0 45W / 300W | 0MiB / 16160MiB | 0% Default |
| | | N/A |
+-------------------------------+----------------------+----------------------+
| 2 Tesla V100-SXM2... On | 00000000:0A:00.0 Off | 0 |
| N/A 36C P0 44W / 300W | 0MiB / 16160MiB | 0% Default |
| | | N/A |
+-------------------------------+----------------------+----------------------+
| 3 Tesla V100-SXM2... On | 00000000:0B:00.0 Off | 0 |
| N/A 33C P0 42W / 300W | 0MiB / 16160MiB | 0% Default |
| | | N/A |
+-------------------------------+----------------------+----------------------+
| 4 Tesla V100-SXM2... On | 00000000:85:00.0 Off | 0 |
| N/A 36C P0 44W / 300W | 0MiB / 16160MiB | 0% Default |
| | | N/A |
+-------------------------------+----------------------+----------------------+
| 5 Tesla V100-SXM2... On | 00000000:86:00.0 Off | 0 |
| N/A 35C P0 42W / 300W | 0MiB / 16160MiB | 0% Default |
| | | N/A |
+-------------------------------+----------------------+----------------------+
| 6 Tesla V100-SXM2... On | 00000000:89:00.0 Off | 0 |
| N/A 36C P0 44W / 300W | 0MiB / 16160MiB | 0% Default |
| | | N/A |
+-------------------------------+----------------------+----------------------+
| 7 Tesla V100-SXM2... On | 00000000:8A:00.0 Off | 0 |
| N/A 34C P0 41W / 300W | 0MiB / 16160MiB | 0% Default |
| | | N/A |
+-------------------------------+----------------------+----------------------+
+-----------------------------------------------------------------------------+
| Processes: |
| GPU GI CI PID Type Process name GPU Memory |
| ID ID Usage |
|=============================================================================|
| No running processes found |
+-----------------------------------------------------------------------------+
The training script, dlrm_train.py
, uses the the embedding collection API.
The script accepts argument that specifies the table placement strategy and use_dynamic_hash_table so we can run the script several times and evaluate different table placement strategy & use_dynamic_hash_table:
%%writefile dlrm_train.py
"""
Copyright (c) 2023, NVIDIA CORPORATION.
Licensed under the Apache License, Version 2.0 (the "License");
you may not use this file except in compliance with the License.
You may obtain a copy of the License at
http://www.apache.org/licenses/LICENSE-2.0
Unless required by applicable law or agreed to in writing, software
distributed under the License is distributed on an "AS IS" BASIS,
WITHOUT WARRANTIES OR CONDITIONS OF ANY KIND, either express or implied.
See the License for the specific language governing permissions and
limitations under the License.
"""
import hugectr
import argparse
from mpi4py import MPI
parser = argparse.ArgumentParser(description="HugeCTR Embedding Collection DLRM model training script.")
parser.add_argument(
"--shard_plan",
help="shard strategy",
type=str,
choices=["round_robin", "uniform", "hybrid"],
)
parser.add_argument(
"--use_dynamic_hash_table",
action="store_true",
)
args = parser.parse_args()
def generate_shard_plan(slot_size_array, num_gpus):
if args.shard_plan == "round_robin":
shard_strategy = [("mp", [str(i) for i in range(len(slot_size_array))])]
shard_matrix = [[] for _ in range(num_gpus)]
for i, table_id in enumerate(range(len(slot_size_array))):
target_gpu = i % num_gpus
shard_matrix[target_gpu].append(str(table_id))
elif args.shard_plan == "uniform":
shard_strategy = [("mp", [str(i) for i in range(len(slot_size_array))])]
shard_matrix = [[] for _ in range(num_gpus)]
for table_id in range(len(slot_size_array)):
for gpu_id in range(num_gpus):
shard_matrix[gpu_id].append(str(table_id))
elif args.shard_plan == "hybrid":
mp_table = [i for i in range(len(slot_size_array)) if slot_size_array[i] > 6000]
dp_table = [i for i in range(len(slot_size_array)) if slot_size_array[i] <= 6000]
shard_matrix = [[] for _ in range(num_gpus)]
shard_strategy = [("mp", [str(i) for i in mp_table]), ("dp", [str(i) for i in dp_table])]
for table_id in dp_table:
for gpu_id in range(num_gpus):
shard_matrix[gpu_id].append(str(table_id))
for i, table_id in enumerate(mp_table):
target_gpu = i % num_gpus
shard_matrix[target_gpu].append(str(table_id))
else:
raise Exception(args.shard_plan + " is not supported")
return shard_matrix, shard_strategy
solver = hugectr.CreateSolver(
max_eval_batches=70,
batchsize_eval=65536,
batchsize=65536,
lr=0.5,
warmup_steps=300,
vvgpu=[[0, 1, 2, 3, 4, 5, 6, 7]],
repeat_dataset=True,
i64_input_key=True,
metrics_spec={hugectr.MetricsType.AverageLoss: 0.0},
use_embedding_collection=True,
)
slot_size_array = [
203931,
18598,
14092,
7012,
18977,
4,
6385,
1245,
49,
186213,
71328,
67288,
11,
2168,
7338,
61,
4,
932,
15,
204515,
141526,
199433,
60919,
9137,
71,
34,
]
reader = hugectr.DataReaderParams(
data_reader_type=hugectr.DataReaderType_t.Parquet,
source=["./criteo_data/train/_file_list.txt"],
eval_source="./criteo_data/val/_file_list.txt",
check_type=hugectr.Check_t.Non,
)
optimizer = hugectr.CreateOptimizer(
optimizer_type=hugectr.Optimizer_t.SGD, update_type=hugectr.Update_t.Local, atomic_update=True
)
model = hugectr.Model(solver, reader, optimizer)
num_embedding = 26
model.add(
hugectr.Input(
label_dim=1,
label_name="label",
dense_dim=13,
dense_name="dense",
data_reader_sparse_param_array=[
hugectr.DataReaderSparseParam("data{}".format(i), 1, False, 1)
for i in range(num_embedding)
],
)
)
# create embedding table
embedding_table_list = []
for i in range(num_embedding):
embedding_table_list.append(
hugectr.EmbeddingTableConfig(
name=str(i), max_vocabulary_size=-1 if args.use_dynamic_hash_table else slot_size_array[i], ev_size=128
)
)
# create ebc config
ebc_config = hugectr.EmbeddingCollectionConfig(use_exclusive_keys=True)
emb_vec_list = []
for i in range(num_embedding):
ebc_config.embedding_lookup(
table_config=embedding_table_list[i],
bottom_name="data{}".format(i),
top_name="emb_vec{}".format(i),
combiner="sum",
)
shard_matrix, shard_strategy = generate_shard_plan(slot_size_array, 8)
ebc_config.shard(shard_matrix=shard_matrix, shard_strategy=shard_strategy)
model.add(ebc_config)
# need concat
model.add(
hugectr.DenseLayer(
layer_type=hugectr.Layer_t.Concat,
bottom_names=["emb_vec{}".format(i) for i in range(num_embedding)],
top_names=["sparse_embedding1"],
)
)
model.add(
hugectr.DenseLayer(
layer_type=hugectr.Layer_t.InnerProduct,
bottom_names=["dense"],
top_names=["fc1"],
num_output=512,
)
)
model.add(
hugectr.DenseLayer(layer_type=hugectr.Layer_t.ReLU, bottom_names=["fc1"], top_names=["relu1"])
)
model.add(
hugectr.DenseLayer(
layer_type=hugectr.Layer_t.InnerProduct,
bottom_names=["relu1"],
top_names=["fc2"],
num_output=256,
)
)
model.add(
hugectr.DenseLayer(layer_type=hugectr.Layer_t.ReLU, bottom_names=["fc2"], top_names=["relu2"])
)
model.add(
hugectr.DenseLayer(
layer_type=hugectr.Layer_t.InnerProduct,
bottom_names=["relu2"],
top_names=["fc3"],
num_output=128,
)
)
model.add(
hugectr.DenseLayer(layer_type=hugectr.Layer_t.ReLU, bottom_names=["fc3"], top_names=["relu3"])
)
model.add(
hugectr.DenseLayer(
layer_type=hugectr.Layer_t.Interaction, # interaction only support 3-D input
bottom_names=["relu3", "sparse_embedding1"],
top_names=["interaction1"],
)
)
model.add(
hugectr.DenseLayer(
layer_type=hugectr.Layer_t.InnerProduct,
bottom_names=["interaction1"],
top_names=["fc4"],
num_output=1024,
)
)
model.add(
hugectr.DenseLayer(layer_type=hugectr.Layer_t.ReLU, bottom_names=["fc4"], top_names=["relu4"])
)
model.add(
hugectr.DenseLayer(
layer_type=hugectr.Layer_t.InnerProduct,
bottom_names=["relu4"],
top_names=["fc5"],
num_output=1024,
)
)
model.add(
hugectr.DenseLayer(layer_type=hugectr.Layer_t.ReLU, bottom_names=["fc5"], top_names=["relu5"])
)
model.add(
hugectr.DenseLayer(
layer_type=hugectr.Layer_t.InnerProduct,
bottom_names=["relu5"],
top_names=["fc6"],
num_output=512,
)
)
model.add(
hugectr.DenseLayer(layer_type=hugectr.Layer_t.ReLU, bottom_names=["fc6"], top_names=["relu6"])
)
model.add(
hugectr.DenseLayer(
layer_type=hugectr.Layer_t.InnerProduct,
bottom_names=["relu6"],
top_names=["fc7"],
num_output=256,
)
)
model.add(
hugectr.DenseLayer(layer_type=hugectr.Layer_t.ReLU, bottom_names=["fc7"], top_names=["relu7"])
)
model.add(
hugectr.DenseLayer(
layer_type=hugectr.Layer_t.InnerProduct,
bottom_names=["relu7"],
top_names=["fc8"],
num_output=1,
)
)
model.add(
hugectr.DenseLayer(
layer_type=hugectr.Layer_t.BinaryCrossEntropyLoss,
bottom_names=["fc8", "label"],
top_names=["loss"],
)
)
model.compile()
model.summary()
model.fit(max_iter=1000, display=100, eval_interval=100, snapshot=10000000, snapshot_prefix="dlrm")
Overwriting dlrm_train.py
Embedding Table Placement Strategy: Round Robin
In this Embedding Table Placement Strategy, we place each table on single GPU in a round robin way.
!python3 dlrm_train.py --shard_plan round_robin
HugeCTR Version: 23.2
====================================================Model Init=====================================================
[HCTR][10:25:19.539][WARNING][RK0][main]: The model name is not specified when creating the solver.
[HCTR][10:25:19.539][INFO][RK0][main]: Global seed is 3508545476
[HCTR][10:25:19.637][INFO][RK0][main]: Device to NUMA mapping:
GPU 0 -> node 0
GPU 1 -> node 0
GPU 2 -> node 0
GPU 3 -> node 0
GPU 4 -> node 1
GPU 5 -> node 1
GPU 6 -> node 1
GPU 7 -> node 1
[HCTR][10:25:30.608][WARNING][RK0][main]: Peer-to-peer access cannot be fully enabled.
[HCTR][10:25:30.608][DEBUG][RK0][main]: [device 0] allocating 0.0000 GB, available 30.4714
[HCTR][10:25:30.608][DEBUG][RK0][main]: [device 1] allocating 0.0000 GB, available 30.4441
[HCTR][10:25:30.609][DEBUG][RK0][main]: [device 2] allocating 0.0000 GB, available 30.5378
[HCTR][10:25:30.609][DEBUG][RK0][main]: [device 3] allocating 0.0000 GB, available 30.5339
[HCTR][10:25:30.609][DEBUG][RK0][main]: [device 4] allocating 0.0000 GB, available 30.4636
[HCTR][10:25:30.609][DEBUG][RK0][main]: [device 5] allocating 0.0000 GB, available 30.4480
[HCTR][10:25:30.609][DEBUG][RK0][main]: [device 6] allocating 0.0000 GB, available 30.4949
[HCTR][10:25:30.609][DEBUG][RK0][main]: [device 7] allocating 0.0000 GB, available 30.5183
[HCTR][10:25:30.609][INFO][RK0][main]: Start all2all warmup
[HCTR][10:25:30.772][INFO][RK0][main]: End all2all warmup
[HCTR][10:25:30.783][INFO][RK0][main]: Using All-reduce algorithm: NCCL
[HCTR][10:25:30.789][INFO][RK0][main]: Device 0: Tesla V100-SXM2-32GB
[HCTR][10:25:30.790][INFO][RK0][main]: Device 1: Tesla V100-SXM2-32GB
[HCTR][10:25:30.790][INFO][RK0][main]: Device 2: Tesla V100-SXM2-32GB
[HCTR][10:25:30.791][INFO][RK0][main]: Device 3: Tesla V100-SXM2-32GB
[HCTR][10:25:30.792][INFO][RK0][main]: Device 4: Tesla V100-SXM2-32GB
[HCTR][10:25:30.792][INFO][RK0][main]: Device 5: Tesla V100-SXM2-32GB
[HCTR][10:25:30.793][INFO][RK0][main]: Device 6: Tesla V100-SXM2-32GB
[HCTR][10:25:30.793][INFO][RK0][main]: Device 7: Tesla V100-SXM2-32GB
[HCTR][10:25:30.919][INFO][RK0][main]: eval source ./deepfm_data_nvt/val/_file_list.txt max_row_group_size 133678
[HCTR][10:25:31.022][INFO][RK0][main]: train source ./deepfm_data_nvt/train/_file_list.txt max_row_group_size 134102
[HCTR][10:25:31.027][INFO][RK0][main]: num of DataReader workers for train: 8
[HCTR][10:25:31.027][INFO][RK0][main]: num of DataReader workers for eval: 8
[HCTR][10:25:31.029][DEBUG][RK0][main]: [device 0] allocating 0.0804 GB, available 30.0457
[HCTR][10:25:31.030][DEBUG][RK0][main]: [device 1] allocating 0.0804 GB, available 30.0183
[HCTR][10:25:31.032][DEBUG][RK0][main]: [device 2] allocating 0.0804 GB, available 30.1121
[HCTR][10:25:31.033][DEBUG][RK0][main]: [device 3] allocating 0.0804 GB, available 30.1082
[HCTR][10:25:31.035][DEBUG][RK0][main]: [device 4] allocating 0.0804 GB, available 30.0378
[HCTR][10:25:31.037][DEBUG][RK0][main]: [device 5] allocating 0.0804 GB, available 30.0222
[HCTR][10:25:31.038][DEBUG][RK0][main]: [device 6] allocating 0.0804 GB, available 30.0691
[HCTR][10:25:31.039][DEBUG][RK0][main]: [device 7] allocating 0.0804 GB, available 30.0925
[HCTR][10:25:31.041][DEBUG][RK0][main]: [device 0] allocating 0.0804 GB, available 29.9636
[HCTR][10:25:31.043][DEBUG][RK0][main]: [device 1] allocating 0.0804 GB, available 29.9363
[HCTR][10:25:31.044][DEBUG][RK0][main]: [device 2] allocating 0.0804 GB, available 30.0300
[HCTR][10:25:31.046][DEBUG][RK0][main]: [device 3] allocating 0.0804 GB, available 30.0261
[HCTR][10:25:31.047][DEBUG][RK0][main]: [device 4] allocating 0.0804 GB, available 29.9558
[HCTR][10:25:31.049][DEBUG][RK0][main]: [device 5] allocating 0.0804 GB, available 29.9402
[HCTR][10:25:31.050][DEBUG][RK0][main]: [device 6] allocating 0.0804 GB, available 29.9871
[HCTR][10:25:31.052][DEBUG][RK0][main]: [device 7] allocating 0.0804 GB, available 30.0105
[HCTR][10:25:31.114][DEBUG][RK0][main]: [device 0] allocating 0.0000 GB, available 29.6863
[HCTR][10:25:31.224][DEBUG][RK0][main]: [device 1] allocating 0.0000 GB, available 29.6589
[HCTR][10:25:31.330][DEBUG][RK0][main]: [device 2] allocating 0.0000 GB, available 29.7527
[HCTR][10:25:31.474][DEBUG][RK0][main]: [device 3] allocating 0.0000 GB, available 29.7488
[HCTR][10:25:31.564][DEBUG][RK0][main]: [device 4] allocating 0.0000 GB, available 29.6785
[HCTR][10:25:31.646][DEBUG][RK0][main]: [device 5] allocating 0.0000 GB, available 29.6628
[HCTR][10:25:31.755][DEBUG][RK0][main]: [device 6] allocating 0.0000 GB, available 29.7097
[HCTR][10:25:31.836][DEBUG][RK0][main]: [device 7] allocating 0.0000 GB, available 29.7332
[HCTR][10:25:32.040][DEBUG][RK0][main]: [device 0] allocating 0.0000 GB, available 29.4089
[HCTR][10:25:32.175][DEBUG][RK0][main]: [device 1] allocating 0.0000 GB, available 29.3816
[HCTR][10:25:32.319][DEBUG][RK0][main]: [device 2] allocating 0.0000 GB, available 29.4753
[HCTR][10:25:32.467][DEBUG][RK0][main]: [device 3] allocating 0.0000 GB, available 29.4714
[HCTR][10:25:32.617][DEBUG][RK0][main]: [device 4] allocating 0.0000 GB, available 29.4011
[HCTR][10:25:32.768][DEBUG][RK0][main]: [device 5] allocating 0.0000 GB, available 29.3855
[HCTR][10:25:32.921][DEBUG][RK0][main]: [device 6] allocating 0.0000 GB, available 29.4324
[HCTR][10:25:33.063][DEBUG][RK0][main]: [device 7] allocating 0.0000 GB, available 29.4558
[HCTR][10:25:33.221][INFO][RK0][main]: Vocabulary size: 0
[HCTR][10:25:33.406][DEBUG][RK0][main]: [device 0] allocating 0.1016 GB, available 28.7546
[HCTR][10:25:33.408][DEBUG][RK0][main]: [device 1] allocating 0.1016 GB, available 28.7253
[HCTR][10:25:33.409][DEBUG][RK0][main]: [device 2] allocating 0.1016 GB, available 28.9402
[HCTR][10:25:33.410][DEBUG][RK0][main]: [device 3] allocating 0.1016 GB, available 28.8425
[HCTR][10:25:33.412][DEBUG][RK0][main]: [device 4] allocating 0.1016 GB, available 28.8308
[HCTR][10:25:33.413][DEBUG][RK0][main]: [device 5] allocating 0.1016 GB, available 28.7957
[HCTR][10:25:33.414][DEBUG][RK0][main]: [device 6] allocating 0.1016 GB, available 28.9031
[HCTR][10:25:33.415][DEBUG][RK0][main]: [device 7] allocating 0.1016 GB, available 28.9578
[HCTR][10:25:33.417][DEBUG][RK0][main]: [device 0] allocating 0.1016 GB, available 28.6531
[HCTR][10:25:33.418][DEBUG][RK0][main]: [device 1] allocating 0.1016 GB, available 28.6238
[HCTR][10:25:33.420][DEBUG][RK0][main]: [device 2] allocating 0.1016 GB, available 28.8386
[HCTR][10:25:33.421][DEBUG][RK0][main]: [device 3] allocating 0.1016 GB, available 28.7410
[HCTR][10:25:33.422][DEBUG][RK0][main]: [device 4] allocating 0.1016 GB, available 28.7292
[HCTR][10:25:33.424][DEBUG][RK0][main]: [device 5] allocating 0.1016 GB, available 28.6941
[HCTR][10:25:33.425][DEBUG][RK0][main]: [device 6] allocating 0.1016 GB, available 28.8015
[HCTR][10:25:33.426][DEBUG][RK0][main]: [device 7] allocating 0.1016 GB, available 28.8562
[HCTR][10:25:33.558][INFO][RK0][main]: Graph analysis to resolve tensor dependency
===================================================Model Compile===================================================
[HCTR][10:25:33.564][DEBUG][RK0][main]: [device 0] allocating 1.4051 GB, available 27.1921
[HCTR][10:25:33.567][DEBUG][RK0][main]: [device 1] allocating 1.4051 GB, available 27.1628
[HCTR][10:25:33.570][DEBUG][RK0][main]: [device 2] allocating 1.4051 GB, available 27.3777
[HCTR][10:25:33.573][DEBUG][RK0][main]: [device 3] allocating 1.4051 GB, available 27.2800
[HCTR][10:25:33.576][DEBUG][RK0][main]: [device 4] allocating 1.4051 GB, available 27.2683
[HCTR][10:25:33.579][DEBUG][RK0][main]: [device 5] allocating 1.4051 GB, available 27.2332
[HCTR][10:25:33.582][DEBUG][RK0][main]: [device 6] allocating 1.4051 GB, available 27.3406
[HCTR][10:25:33.585][DEBUG][RK0][main]: [device 7] allocating 1.4051 GB, available 27.3953
[HCTR][10:25:33.587][DEBUG][RK0][main]: [device 0] allocating 0.0088 GB, available 27.1824
[HCTR][10:25:33.588][DEBUG][RK0][main]: [device 1] allocating 0.0088 GB, available 27.1531
[HCTR][10:25:33.589][DEBUG][RK0][main]: [device 2] allocating 0.0088 GB, available 27.3679
[HCTR][10:25:33.590][DEBUG][RK0][main]: [device 3] allocating 0.0088 GB, available 27.2703
[HCTR][10:25:33.591][DEBUG][RK0][main]: [device 4] allocating 0.0088 GB, available 27.2585
[HCTR][10:25:33.592][DEBUG][RK0][main]: [device 5] allocating 0.0088 GB, available 27.2234
[HCTR][10:25:33.593][DEBUG][RK0][main]: [device 6] allocating 0.0088 GB, available 27.3308
[HCTR][10:25:33.595][DEBUG][RK0][main]: [device 7] allocating 0.0088 GB, available 27.3855
===================================================Model Summary===================================================
[HCTR][10:26:11.457][INFO][RK0][main]: Model structure on each GPU
Label Dense Sparse
label dense data0,data1,data2,data3,data4,data5,data6,data7,data8,data9,data10,data11,data12,data13,data14,data15,data16,data17,data18,data19,data20,data21,data22,data23,data24,data25
(8192,1) (8192,13)
——————————————————————————————————————————————————————————————————————————————————————————————————————————————————
Layer Type Input Name Output Name Output Shape
——————————————————————————————————————————————————————————————————————————————————————————————————————————————————
EmbeddingCollection0 data0 emb_vec0 (8192,1,128)
data1 emb_vec1 (8192,1,128)
data2 emb_vec2 (8192,1,128)
data3 emb_vec3 (8192,1,128)
data4 emb_vec4 (8192,1,128)
data5 emb_vec5 (8192,1,128)
data6 emb_vec6 (8192,1,128)
data7 emb_vec7 (8192,1,128)
data8 emb_vec8 (8192,1,128)
data9 emb_vec9 (8192,1,128)
data10 emb_vec10 (8192,1,128)
data11 emb_vec11 (8192,1,128)
data12 emb_vec12 (8192,1,128)
data13 emb_vec13 (8192,1,128)
data14 emb_vec14 (8192,1,128)
data15 emb_vec15 (8192,1,128)
data16 emb_vec16 (8192,1,128)
data17 emb_vec17 (8192,1,128)
data18 emb_vec18 (8192,1,128)
data19 emb_vec19 (8192,1,128)
data20 emb_vec20 (8192,1,128)
data21 emb_vec21 (8192,1,128)
data22 emb_vec22 (8192,1,128)
data23 emb_vec23 (8192,1,128)
data24 emb_vec24 (8192,1,128)
data25 emb_vec25 (8192,1,128)
------------------------------------------------------------------------------------------------------------------
Concat emb_vec0 sparse_embedding1 (8192,26,128)
emb_vec1
emb_vec2
emb_vec3
emb_vec4
emb_vec5
emb_vec6
emb_vec7
emb_vec8
emb_vec9
emb_vec10
emb_vec11
emb_vec12
emb_vec13
emb_vec14
emb_vec15
emb_vec16
emb_vec17
emb_vec18
emb_vec19
emb_vec20
emb_vec21
emb_vec22
emb_vec23
emb_vec24
emb_vec25
------------------------------------------------------------------------------------------------------------------
InnerProduct dense fc1 (8192,512)
------------------------------------------------------------------------------------------------------------------
ReLU fc1 relu1 (8192,512)
------------------------------------------------------------------------------------------------------------------
InnerProduct relu1 fc2 (8192,256)
------------------------------------------------------------------------------------------------------------------
ReLU fc2 relu2 (8192,256)
------------------------------------------------------------------------------------------------------------------
InnerProduct relu2 fc3 (8192,128)
------------------------------------------------------------------------------------------------------------------
ReLU fc3 relu3 (8192,128)
------------------------------------------------------------------------------------------------------------------
Interaction relu3 interaction1 (8192,480)
sparse_embedding1
------------------------------------------------------------------------------------------------------------------
InnerProduct interaction1 fc4 (8192,1024)
------------------------------------------------------------------------------------------------------------------
ReLU fc4 relu4 (8192,1024)
------------------------------------------------------------------------------------------------------------------
InnerProduct relu4 fc5 (8192,1024)
------------------------------------------------------------------------------------------------------------------
ReLU fc5 relu5 (8192,1024)
------------------------------------------------------------------------------------------------------------------
InnerProduct relu5 fc6 (8192,512)
------------------------------------------------------------------------------------------------------------------
ReLU fc6 relu6 (8192,512)
------------------------------------------------------------------------------------------------------------------
InnerProduct relu6 fc7 (8192,256)
------------------------------------------------------------------------------------------------------------------
ReLU fc7 relu7 (8192,256)
------------------------------------------------------------------------------------------------------------------
InnerProduct relu7 fc8 (8192,1)
------------------------------------------------------------------------------------------------------------------
BinaryCrossEntropyLoss fc8 loss
label
------------------------------------------------------------------------------------------------------------------
=====================================================Model Fit=====================================================
[HCTR][10:26:11.457][INFO][RK0][main]: Use non-epoch mode with number of iterations: 1000
[HCTR][10:26:11.457][INFO][RK0][main]: Training batchsize: 65536, evaluation batchsize: 65536
[HCTR][10:26:11.457][INFO][RK0][main]: Evaluation interval: 100, snapshot interval: 10000000
[HCTR][10:26:11.457][INFO][RK0][main]: Dense network trainable: True
[HCTR][10:26:11.457][INFO][RK0][main]: Use mixed precision: False, scaler: 1.000000, use cuda graph: True
[HCTR][10:26:11.457][INFO][RK0][main]: lr: 0.500000, warmup_steps: 300, end_lr: 0.000000
[HCTR][10:26:11.457][INFO][RK0][main]: decay_start: 0, decay_steps: 1, decay_power: 2.000000
[HCTR][10:26:11.457][INFO][RK0][main]: Training source file: ./deepfm_data_nvt/train/_file_list.txt
[HCTR][10:26:11.458][INFO][RK0][main]: Evaluation source file: ./deepfm_data_nvt/val/_file_list.txt
[HCTR][10:26:15.652][INFO][RK0][main]: Evaluation, AverageLoss: 0.14373
[HCTR][10:26:15.652][INFO][RK0][main]: Eval Time for 70 iters: 1.24478s
[HCTR][10:26:15.697][INFO][RK0][main]: Iter: 100 Time(100 iters): 4.23782s Loss: 0.142604 lr:0.168333
[HCTR][10:26:19.865][INFO][RK0][main]: Evaluation, AverageLoss: 0.142137
[HCTR][10:26:19.865][INFO][RK0][main]: Eval Time for 70 iters: 1.25698s
[HCTR][10:26:19.899][INFO][RK0][main]: Iter: 200 Time(100 iters): 4.19912s Loss: 0.142685 lr:0.335
[HCTR][10:26:24.035][INFO][RK0][main]: Evaluation, AverageLoss: 0.1404
[HCTR][10:26:24.035][INFO][RK0][main]: Eval Time for 70 iters: 1.24589s
[HCTR][10:26:24.080][INFO][RK0][main]: Iter: 300 Time(100 iters): 4.18021s Loss: 0.143021 lr:0.5
[HCTR][10:26:28.211][INFO][RK0][main]: Evaluation, AverageLoss: 0.139695
[HCTR][10:26:28.211][INFO][RK0][main]: Eval Time for 70 iters: 1.25073s
[HCTR][10:26:28.245][INFO][RK0][main]: Iter: 400 Time(100 iters): 4.16407s Loss: 0.141111 lr:0.5
[HCTR][10:26:32.375][INFO][RK0][main]: Evaluation, AverageLoss: 0.13893
[HCTR][10:26:32.375][INFO][RK0][main]: Eval Time for 70 iters: 1.24958s
[HCTR][10:26:32.419][INFO][RK0][main]: Iter: 500 Time(100 iters): 4.17112s Loss: 0.141069 lr:0.5
[HCTR][10:26:36.558][INFO][RK0][main]: Evaluation, AverageLoss: 0.138218
[HCTR][10:26:36.558][INFO][RK0][main]: Eval Time for 70 iters: 1.25123s
[HCTR][10:26:36.606][INFO][RK0][main]: Iter: 600 Time(100 iters): 4.18422s Loss: 0.135439 lr:0.5
[HCTR][10:26:40.759][INFO][RK0][main]: Evaluation, AverageLoss: 0.137244
[HCTR][10:26:40.759][INFO][RK0][main]: Eval Time for 70 iters: 1.25471s
[HCTR][10:26:40.803][INFO][RK0][main]: Iter: 700 Time(100 iters): 4.19334s Loss: 0.139792 lr:0.5
[HCTR][10:26:44.933][INFO][RK0][main]: Evaluation, AverageLoss: 0.136812
[HCTR][10:26:44.933][INFO][RK0][main]: Eval Time for 70 iters: 1.2416s
[HCTR][10:26:44.979][INFO][RK0][main]: Iter: 800 Time(100 iters): 4.17574s Loss: 0.140519 lr:0.5
[HCTR][10:26:49.115][INFO][RK0][main]: Evaluation, AverageLoss: 0.135968
[HCTR][10:26:49.116][INFO][RK0][main]: Eval Time for 70 iters: 1.25386s
[HCTR][10:26:49.163][INFO][RK0][main]: Iter: 900 Time(100 iters): 4.18238s Loss: 0.134846 lr:0.5
[HCTR][10:26:53.291][INFO][RK0][main]: Evaluation, AverageLoss: 0.134873
[HCTR][10:26:53.292][INFO][RK0][main]: Eval Time for 70 iters: 1.23619s
[HCTR][10:26:53.292][INFO][RK0][main]: Finish 1000 iterations with batchsize: 65536 in 41.83s.
Embedding Table Placement Strategy: Uniform
In this Embedding Table Placement Strategy, we place each table on all 8 GPUs.
!python3 dlrm_train.py --shard_plan uniform
HugeCTR Version: 23.3
====================================================Model Init=====================================================
[HCTR][06:33:37.284][WARNING][RK0][main]: The model name is not specified when creating the solver.
[HCTR][06:33:37.284][INFO][RK0][main]: Global seed is 3445591887
[HCTR][06:33:37.408][INFO][RK0][main]: Device to NUMA mapping:
GPU 0 -> node 0
GPU 1 -> node 0
GPU 2 -> node 0
GPU 3 -> node 0
GPU 4 -> node 1
GPU 5 -> node 1
GPU 6 -> node 1
GPU 7 -> node 1
[HCTR][06:33:56.383][WARNING][RK0][main]: Peer-to-peer access cannot be fully enabled.
[HCTR][06:33:56.384][DEBUG][RK0][main]: [device 0] allocating 0.0000 GB, available 30.4714
[HCTR][06:33:56.385][DEBUG][RK0][main]: [device 1] allocating 0.0000 GB, available 30.4441
[HCTR][06:33:56.385][DEBUG][RK0][main]: [device 2] allocating 0.0000 GB, available 30.5378
[HCTR][06:33:56.385][DEBUG][RK0][main]: [device 3] allocating 0.0000 GB, available 30.5339
[HCTR][06:33:56.385][DEBUG][RK0][main]: [device 4] allocating 0.0000 GB, available 30.4636
[HCTR][06:33:56.386][DEBUG][RK0][main]: [device 5] allocating 0.0000 GB, available 30.4480
[HCTR][06:33:56.386][DEBUG][RK0][main]: [device 6] allocating 0.0000 GB, available 30.4949
[HCTR][06:33:56.386][DEBUG][RK0][main]: [device 7] allocating 0.0000 GB, available 30.5183
[HCTR][06:33:56.386][INFO][RK0][main]: Start all2all warmup
[HCTR][06:33:56.628][INFO][RK0][main]: End all2all warmup
[HCTR][06:33:56.643][INFO][RK0][main]: Using All-reduce algorithm: NCCL
[HCTR][06:33:56.650][INFO][RK0][main]: Device 0: Tesla V100-SXM2-32GB
[HCTR][06:33:56.650][INFO][RK0][main]: Device 1: Tesla V100-SXM2-32GB
[HCTR][06:33:56.651][INFO][RK0][main]: Device 2: Tesla V100-SXM2-32GB
[HCTR][06:33:56.652][INFO][RK0][main]: Device 3: Tesla V100-SXM2-32GB
[HCTR][06:33:56.652][INFO][RK0][main]: Device 4: Tesla V100-SXM2-32GB
[HCTR][06:33:56.653][INFO][RK0][main]: Device 5: Tesla V100-SXM2-32GB
[HCTR][06:33:56.654][INFO][RK0][main]: Device 6: Tesla V100-SXM2-32GB
[HCTR][06:33:56.654][INFO][RK0][main]: Device 7: Tesla V100-SXM2-32GB
[HCTR][06:33:56.785][INFO][RK0][main]: eval source ./deepfm_data_nvt/val/_file_list.txt max_row_group_size 133678
[HCTR][06:33:56.939][INFO][RK0][main]: train source ./deepfm_data_nvt/train/_file_list.txt max_row_group_size 134102
[HCTR][06:33:56.946][INFO][RK0][main]: num of DataReader workers for train: 8
[HCTR][06:33:56.946][INFO][RK0][main]: num of DataReader workers for eval: 8
[HCTR][06:33:56.997][DEBUG][RK0][main]: [device 0] allocating 0.0258 GB, available 30.0417
[HCTR][06:33:57.001][DEBUG][RK0][main]: [device 1] allocating 0.0258 GB, available 30.0144
[HCTR][06:33:57.006][DEBUG][RK0][main]: [device 2] allocating 0.0258 GB, available 30.1082
[HCTR][06:33:57.011][DEBUG][RK0][main]: [device 3] allocating 0.0258 GB, available 30.1042
[HCTR][06:33:57.015][DEBUG][RK0][main]: [device 4] allocating 0.0258 GB, available 30.0339
[HCTR][06:33:57.020][DEBUG][RK0][main]: [device 5] allocating 0.0258 GB, available 30.0183
[HCTR][06:33:57.024][DEBUG][RK0][main]: [device 6] allocating 0.0258 GB, available 30.0652
[HCTR][06:33:57.029][DEBUG][RK0][main]: [device 7] allocating 0.0258 GB, available 30.0886
[HCTR][06:33:57.071][DEBUG][RK0][main]: [device 0] allocating 0.0258 GB, available 29.9558
[HCTR][06:33:57.075][DEBUG][RK0][main]: [device 1] allocating 0.0258 GB, available 29.9285
[HCTR][06:33:57.080][DEBUG][RK0][main]: [device 2] allocating 0.0258 GB, available 30.0222
[HCTR][06:33:57.084][DEBUG][RK0][main]: [device 3] allocating 0.0258 GB, available 30.0183
[HCTR][06:33:57.088][DEBUG][RK0][main]: [device 4] allocating 0.0258 GB, available 29.9480
[HCTR][06:33:57.092][DEBUG][RK0][main]: [device 5] allocating 0.0258 GB, available 29.9324
[HCTR][06:33:57.097][DEBUG][RK0][main]: [device 6] allocating 0.0258 GB, available 29.9792
[HCTR][06:33:57.101][DEBUG][RK0][main]: [device 7] allocating 0.0258 GB, available 30.0027
[HCTR][06:33:59.332][INFO][RK0][main]: Vocabulary size: 0
[HCTR][06:33:59.745][DEBUG][RK0][main]: [device 0] allocating 0.1016 GB, available 25.9753
[HCTR][06:33:59.746][DEBUG][RK0][main]: [device 1] allocating 0.1016 GB, available 25.9480
[HCTR][06:33:59.748][DEBUG][RK0][main]: [device 2] allocating 0.1016 GB, available 26.0417
[HCTR][06:33:59.749][DEBUG][RK0][main]: [device 3] allocating 0.1016 GB, available 26.0378
[HCTR][06:33:59.751][DEBUG][RK0][main]: [device 4] allocating 0.1016 GB, available 25.9675
[HCTR][06:33:59.752][DEBUG][RK0][main]: [device 5] allocating 0.1016 GB, available 25.9519
[HCTR][06:33:59.754][DEBUG][RK0][main]: [device 6] allocating 0.1016 GB, available 25.9988
[HCTR][06:33:59.756][DEBUG][RK0][main]: [device 7] allocating 0.1016 GB, available 26.0222
[HCTR][06:33:59.757][DEBUG][RK0][main]: [device 0] allocating 0.1016 GB, available 25.8738
[HCTR][06:33:59.759][DEBUG][RK0][main]: [device 1] allocating 0.1016 GB, available 25.8464
[HCTR][06:33:59.760][DEBUG][RK0][main]: [device 2] allocating 0.1016 GB, available 25.9402
[HCTR][06:33:59.762][DEBUG][RK0][main]: [device 3] allocating 0.1016 GB, available 25.9363
[HCTR][06:33:59.763][DEBUG][RK0][main]: [device 4] allocating 0.1016 GB, available 25.8660
[HCTR][06:33:59.765][DEBUG][RK0][main]: [device 5] allocating 0.1016 GB, available 25.8503
[HCTR][06:33:59.767][DEBUG][RK0][main]: [device 6] allocating 0.1016 GB, available 25.8972
[HCTR][06:33:59.768][DEBUG][RK0][main]: [device 7] allocating 0.1016 GB, available 25.9207
[HCTR][06:33:59.911][INFO][RK0][main]: Graph analysis to resolve tensor dependency
===================================================Model Compile===================================================
[HCTR][06:33:59.917][DEBUG][RK0][main]: [device 0] allocating 1.4051 GB, available 24.4128
[HCTR][06:33:59.921][DEBUG][RK0][main]: [device 1] allocating 1.4051 GB, available 24.3855
[HCTR][06:33:59.924][DEBUG][RK0][main]: [device 2] allocating 1.4051 GB, available 24.4792
[HCTR][06:33:59.927][DEBUG][RK0][main]: [device 3] allocating 1.4051 GB, available 24.4753
[HCTR][06:33:59.930][DEBUG][RK0][main]: [device 4] allocating 1.4051 GB, available 24.4050
[HCTR][06:33:59.934][DEBUG][RK0][main]: [device 5] allocating 1.4051 GB, available 24.3894
[HCTR][06:33:59.937][DEBUG][RK0][main]: [device 6] allocating 1.4051 GB, available 24.4363
[HCTR][06:33:59.940][DEBUG][RK0][main]: [device 7] allocating 1.4051 GB, available 24.4597
[HCTR][06:33:59.941][DEBUG][RK0][main]: [device 0] allocating 0.0088 GB, available 24.4031
[HCTR][06:33:59.942][DEBUG][RK0][main]: [device 1] allocating 0.0088 GB, available 24.3757
[HCTR][06:33:59.944][DEBUG][RK0][main]: [device 2] allocating 0.0088 GB, available 24.4695
[HCTR][06:33:59.945][DEBUG][RK0][main]: [device 3] allocating 0.0088 GB, available 24.4656
[HCTR][06:33:59.946][DEBUG][RK0][main]: [device 4] allocating 0.0088 GB, available 24.3953
[HCTR][06:33:59.947][DEBUG][RK0][main]: [device 5] allocating 0.0088 GB, available 24.3796
[HCTR][06:33:59.948][DEBUG][RK0][main]: [device 6] allocating 0.0088 GB, available 24.4265
[HCTR][06:33:59.950][DEBUG][RK0][main]: [device 7] allocating 0.0088 GB, available 24.4500
===================================================Model Summary===================================================
[HCTR][06:34:37.841][INFO][RK0][main]: Model structure on each GPU
Label Dense Sparse
label dense data0,data1,data2,data3,data4,data5,data6,data7,data8,data9,data10,data11,data12,data13,data14,data15,data16,data17,data18,data19,data20,data21,data22,data23,data24,data25
(8192,1) (8192,13)
——————————————————————————————————————————————————————————————————————————————————————————————————————————————————
Layer Type Input Name Output Name Output Shape
——————————————————————————————————————————————————————————————————————————————————————————————————————————————————
EmbeddingCollection0 data0 emb_vec0 (8192,1,128)
data1 emb_vec1 (8192,1,128)
data2 emb_vec2 (8192,1,128)
data3 emb_vec3 (8192,1,128)
data4 emb_vec4 (8192,1,128)
data5 emb_vec5 (8192,1,128)
data6 emb_vec6 (8192,1,128)
data7 emb_vec7 (8192,1,128)
data8 emb_vec8 (8192,1,128)
data9 emb_vec9 (8192,1,128)
data10 emb_vec10 (8192,1,128)
data11 emb_vec11 (8192,1,128)
data12 emb_vec12 (8192,1,128)
data13 emb_vec13 (8192,1,128)
data14 emb_vec14 (8192,1,128)
data15 emb_vec15 (8192,1,128)
data16 emb_vec16 (8192,1,128)
data17 emb_vec17 (8192,1,128)
data18 emb_vec18 (8192,1,128)
data19 emb_vec19 (8192,1,128)
data20 emb_vec20 (8192,1,128)
data21 emb_vec21 (8192,1,128)
data22 emb_vec22 (8192,1,128)
data23 emb_vec23 (8192,1,128)
data24 emb_vec24 (8192,1,128)
data25 emb_vec25 (8192,1,128)
------------------------------------------------------------------------------------------------------------------
Concat emb_vec0 sparse_embedding1 (8192,26,128)
emb_vec1
emb_vec2
emb_vec3
emb_vec4
emb_vec5
emb_vec6
emb_vec7
emb_vec8
emb_vec9
emb_vec10
emb_vec11
emb_vec12
emb_vec13
emb_vec14
emb_vec15
emb_vec16
emb_vec17
emb_vec18
emb_vec19
emb_vec20
emb_vec21
emb_vec22
emb_vec23
emb_vec24
emb_vec25
------------------------------------------------------------------------------------------------------------------
InnerProduct dense fc1 (8192,512)
------------------------------------------------------------------------------------------------------------------
ReLU fc1 relu1 (8192,512)
------------------------------------------------------------------------------------------------------------------
InnerProduct relu1 fc2 (8192,256)
------------------------------------------------------------------------------------------------------------------
ReLU fc2 relu2 (8192,256)
------------------------------------------------------------------------------------------------------------------
InnerProduct relu2 fc3 (8192,128)
------------------------------------------------------------------------------------------------------------------
ReLU fc3 relu3 (8192,128)
------------------------------------------------------------------------------------------------------------------
Interaction relu3 interaction1 (8192,480)
sparse_embedding1
------------------------------------------------------------------------------------------------------------------
InnerProduct interaction1 fc4 (8192,1024)
------------------------------------------------------------------------------------------------------------------
ReLU fc4 relu4 (8192,1024)
------------------------------------------------------------------------------------------------------------------
InnerProduct relu4 fc5 (8192,1024)
------------------------------------------------------------------------------------------------------------------
ReLU fc5 relu5 (8192,1024)
------------------------------------------------------------------------------------------------------------------
InnerProduct relu5 fc6 (8192,512)
------------------------------------------------------------------------------------------------------------------
ReLU fc6 relu6 (8192,512)
------------------------------------------------------------------------------------------------------------------
InnerProduct relu6 fc7 (8192,256)
------------------------------------------------------------------------------------------------------------------
ReLU fc7 relu7 (8192,256)
------------------------------------------------------------------------------------------------------------------
InnerProduct relu7 fc8 (8192,1)
------------------------------------------------------------------------------------------------------------------
BinaryCrossEntropyLoss fc8 loss
label
------------------------------------------------------------------------------------------------------------------
=====================================================Model Fit=====================================================
[HCTR][06:34:37.842][INFO][RK0][main]: Use non-epoch mode with number of iterations: 1000
[HCTR][06:34:37.842][INFO][RK0][main]: Training batchsize: 65536, evaluation batchsize: 65536
[HCTR][06:34:37.842][INFO][RK0][main]: Evaluation interval: 100, snapshot interval: 10000000
[HCTR][06:34:37.842][INFO][RK0][main]: Dense network trainable: True
[HCTR][06:34:37.842][INFO][RK0][main]: Use mixed precision: False, scaler: 1.000000, use cuda graph: True
[HCTR][06:34:37.842][INFO][RK0][main]: lr: 0.500000, warmup_steps: 300, end_lr: 0.000000
[HCTR][06:34:37.842][INFO][RK0][main]: decay_start: 0, decay_steps: 1, decay_power: 2.000000
[HCTR][06:34:37.842][INFO][RK0][main]: Training source file: ./deepfm_data_nvt/train/_file_list.txt
[HCTR][06:34:37.842][INFO][RK0][main]: Evaluation source file: ./deepfm_data_nvt/val/_file_list.txt
[HCTR][06:34:46.251][INFO][RK0][main]: Evaluation, AverageLoss: 0.143524
[HCTR][06:34:46.251][INFO][RK0][main]: Eval Time for 70 iters: 2.34586s
[HCTR][06:34:46.345][INFO][RK0][main]: Iter: 100 Time(100 iters): 8.48449s Loss: 0.142247 lr:0.168333
[HCTR][06:34:54.657][INFO][RK0][main]: Evaluation, AverageLoss: 0.141641
[HCTR][06:34:54.657][INFO][RK0][main]: Eval Time for 70 iters: 2.33134s
[HCTR][06:34:54.751][INFO][RK0][main]: Iter: 200 Time(100 iters): 8.40384s Loss: 0.142243 lr:0.335
[HCTR][06:35:03.069][INFO][RK0][main]: Evaluation, AverageLoss: 0.139913
[HCTR][06:35:03.069][INFO][RK0][main]: Eval Time for 70 iters: 2.33118s
[HCTR][06:35:03.161][INFO][RK0][main]: Iter: 300 Time(100 iters): 8.40793s Loss: 0.142713 lr:0.5
[HCTR][06:35:11.479][INFO][RK0][main]: Evaluation, AverageLoss: 0.138901
[HCTR][06:35:11.479][INFO][RK0][main]: Eval Time for 70 iters: 2.34956s
[HCTR][06:35:11.568][INFO][RK0][main]: Iter: 400 Time(100 iters): 8.40618s Loss: 0.140238 lr:0.5
[HCTR][06:35:19.883][INFO][RK0][main]: Evaluation, AverageLoss: 0.138208
[HCTR][06:35:19.883][INFO][RK0][main]: Eval Time for 70 iters: 2.34071s
[HCTR][06:35:19.974][INFO][RK0][main]: Iter: 500 Time(100 iters): 8.38745s Loss: 0.140117 lr:0.5
[HCTR][06:35:28.326][INFO][RK0][main]: Evaluation, AverageLoss: 0.137638
[HCTR][06:35:28.326][INFO][RK0][main]: Eval Time for 70 iters: 2.34076s
[HCTR][06:35:28.415][INFO][RK0][main]: Iter: 600 Time(100 iters): 8.42352s Loss: 0.135055 lr:0.5
[HCTR][06:35:36.727][INFO][RK0][main]: Evaluation, AverageLoss: 0.137268
[HCTR][06:35:36.728][INFO][RK0][main]: Eval Time for 70 iters: 2.33588s
[HCTR][06:35:36.819][INFO][RK0][main]: Iter: 700 Time(100 iters): 8.38619s Loss: 0.139783 lr:0.5
[HCTR][06:35:45.193][INFO][RK0][main]: Evaluation, AverageLoss: 0.136816
[HCTR][06:35:45.193][INFO][RK0][main]: Eval Time for 70 iters: 2.3762s
[HCTR][06:35:45.253][INFO][RK0][main]: Iter: 800 Time(100 iters): 8.43341s Loss: 0.140772 lr:0.5
[HCTR][06:35:53.581][INFO][RK0][main]: Evaluation, AverageLoss: 0.136368
[HCTR][06:35:53.581][INFO][RK0][main]: Eval Time for 70 iters: 2.3521s
[HCTR][06:35:53.673][INFO][RK0][main]: Iter: 900 Time(100 iters): 8.41807s Loss: 0.135264 lr:0.5
[HCTR][06:36:01.985][INFO][RK0][main]: Evaluation, AverageLoss: 0.135726
[HCTR][06:36:01.985][INFO][RK0][main]: Eval Time for 70 iters: 2.34242s
[HCTR][06:36:01.985][INFO][RK0][main]: Finish 1000 iterations with batchsize: 65536 in 84.14s.
Embedding Table Placement Strategy: Hybrid
In this Embedding Table Placement Strategy, we place small table (size < 6000) in a data parallel way and large table(size >= 6000) in a round robin way
!python3 dlrm_train.py --shard_plan hybrid
HugeCTR Version: 23.2
====================================================Model Init=====================================================
[HCTR][10:35:14.415][WARNING][RK0][main]: The model name is not specified when creating the solver.
[HCTR][10:35:14.415][INFO][RK0][main]: Global seed is 198655838
[HCTR][10:35:14.517][INFO][RK0][main]: Device to NUMA mapping:
GPU 0 -> node 0
GPU 1 -> node 0
GPU 2 -> node 0
GPU 3 -> node 0
GPU 4 -> node 1
GPU 5 -> node 1
GPU 6 -> node 1
GPU 7 -> node 1
[HCTR][10:35:25.730][WARNING][RK0][main]: Peer-to-peer access cannot be fully enabled.
[HCTR][10:35:25.731][DEBUG][RK0][main]: [device 0] allocating 0.0000 GB, available 30.4714
[HCTR][10:35:25.731][DEBUG][RK0][main]: [device 1] allocating 0.0000 GB, available 30.4441
[HCTR][10:35:25.731][DEBUG][RK0][main]: [device 2] allocating 0.0000 GB, available 30.5378
[HCTR][10:35:25.731][DEBUG][RK0][main]: [device 3] allocating 0.0000 GB, available 30.5339
[HCTR][10:35:25.731][DEBUG][RK0][main]: [device 4] allocating 0.0000 GB, available 30.4636
[HCTR][10:35:25.731][DEBUG][RK0][main]: [device 5] allocating 0.0000 GB, available 30.4480
[HCTR][10:35:25.731][DEBUG][RK0][main]: [device 6] allocating 0.0000 GB, available 30.4949
[HCTR][10:35:25.731][DEBUG][RK0][main]: [device 7] allocating 0.0000 GB, available 30.5183
[HCTR][10:35:25.732][INFO][RK0][main]: Start all2all warmup
[HCTR][10:35:25.896][INFO][RK0][main]: End all2all warmup
[HCTR][10:35:25.907][INFO][RK0][main]: Using All-reduce algorithm: NCCL
[HCTR][10:35:25.913][INFO][RK0][main]: Device 0: Tesla V100-SXM2-32GB
[HCTR][10:35:25.914][INFO][RK0][main]: Device 1: Tesla V100-SXM2-32GB
[HCTR][10:35:25.914][INFO][RK0][main]: Device 2: Tesla V100-SXM2-32GB
[HCTR][10:35:25.915][INFO][RK0][main]: Device 3: Tesla V100-SXM2-32GB
[HCTR][10:35:25.916][INFO][RK0][main]: Device 4: Tesla V100-SXM2-32GB
[HCTR][10:35:25.916][INFO][RK0][main]: Device 5: Tesla V100-SXM2-32GB
[HCTR][10:35:25.917][INFO][RK0][main]: Device 6: Tesla V100-SXM2-32GB
[HCTR][10:35:25.917][INFO][RK0][main]: Device 7: Tesla V100-SXM2-32GB
[HCTR][10:35:25.969][INFO][RK0][main]: eval source ./deepfm_data_nvt/val/_file_list.txt max_row_group_size 133678
[HCTR][10:35:26.002][INFO][RK0][main]: train source ./deepfm_data_nvt/train/_file_list.txt max_row_group_size 134102
[HCTR][10:35:26.004][INFO][RK0][main]: num of DataReader workers for train: 8
[HCTR][10:35:26.004][INFO][RK0][main]: num of DataReader workers for eval: 8
[HCTR][10:35:26.005][DEBUG][RK0][main]: [device 0] allocating 0.0804 GB, available 30.0457
[HCTR][10:35:26.007][DEBUG][RK0][main]: [device 1] allocating 0.0804 GB, available 30.0183
[HCTR][10:35:26.008][DEBUG][RK0][main]: [device 2] allocating 0.0804 GB, available 30.1121
[HCTR][10:35:26.009][DEBUG][RK0][main]: [device 3] allocating 0.0804 GB, available 30.1082
[HCTR][10:35:26.010][DEBUG][RK0][main]: [device 4] allocating 0.0804 GB, available 30.0378
[HCTR][10:35:26.012][DEBUG][RK0][main]: [device 5] allocating 0.0804 GB, available 30.0222
[HCTR][10:35:26.013][DEBUG][RK0][main]: [device 6] allocating 0.0804 GB, available 30.0691
[HCTR][10:35:26.014][DEBUG][RK0][main]: [device 7] allocating 0.0804 GB, available 30.0925
[HCTR][10:35:26.016][DEBUG][RK0][main]: [device 0] allocating 0.0804 GB, available 29.9636
[HCTR][10:35:26.017][DEBUG][RK0][main]: [device 1] allocating 0.0804 GB, available 29.9363
[HCTR][10:35:26.018][DEBUG][RK0][main]: [device 2] allocating 0.0804 GB, available 30.0300
[HCTR][10:35:26.020][DEBUG][RK0][main]: [device 3] allocating 0.0804 GB, available 30.0261
[HCTR][10:35:26.021][DEBUG][RK0][main]: [device 4] allocating 0.0804 GB, available 29.9558
[HCTR][10:35:26.022][DEBUG][RK0][main]: [device 5] allocating 0.0804 GB, available 29.9402
[HCTR][10:35:26.023][DEBUG][RK0][main]: [device 6] allocating 0.0804 GB, available 29.9871
[HCTR][10:35:26.025][DEBUG][RK0][main]: [device 7] allocating 0.0804 GB, available 30.0105
[HCTR][10:35:26.081][DEBUG][RK0][main]: [device 0] allocating 0.0000 GB, available 29.6863
[HCTR][10:35:26.121][DEBUG][RK0][main]: [device 1] allocating 0.0000 GB, available 29.6589
[HCTR][10:35:26.423][DEBUG][RK0][main]: [device 2] allocating 0.0000 GB, available 29.7527
[HCTR][10:35:26.505][DEBUG][RK0][main]: [device 3] allocating 0.0000 GB, available 29.7488
[HCTR][10:35:27.056][DEBUG][RK0][main]: [device 4] allocating 0.0000 GB, available 29.6785
[HCTR][10:35:27.145][DEBUG][RK0][main]: [device 5] allocating 0.0000 GB, available 29.6628
[HCTR][10:35:27.235][DEBUG][RK0][main]: [device 6] allocating 0.0000 GB, available 29.7097
[HCTR][10:35:27.559][DEBUG][RK0][main]: [device 7] allocating 0.0000 GB, available 29.7332
[HCTR][10:35:27.747][DEBUG][RK0][main]: [device 0] allocating 0.0000 GB, available 29.4089
[HCTR][10:35:29.286][DEBUG][RK0][main]: [device 1] allocating 0.0000 GB, available 29.3816
[HCTR][10:35:30.351][DEBUG][RK0][main]: [device 2] allocating 0.0000 GB, available 29.4753
[HCTR][10:35:31.224][DEBUG][RK0][main]: [device 3] allocating 0.0000 GB, available 29.4714
[HCTR][10:35:31.749][DEBUG][RK0][main]: [device 4] allocating 0.0000 GB, available 29.4011
[HCTR][10:35:32.275][DEBUG][RK0][main]: [device 5] allocating 0.0000 GB, available 29.3855
[HCTR][10:35:33.299][DEBUG][RK0][main]: [device 6] allocating 0.0000 GB, available 29.4324
[HCTR][10:35:34.091][DEBUG][RK0][main]: [device 7] allocating 0.0000 GB, available 29.4558
[HCTR][10:35:34.133][INFO][RK0][main]: Vocabulary size: 0
[HCTR][10:35:34.361][DEBUG][RK0][main]: [device 0] allocating 0.1016 GB, available 28.9285
[HCTR][10:35:34.363][DEBUG][RK0][main]: [device 1] allocating 0.1016 GB, available 29.0203
[HCTR][10:35:34.364][DEBUG][RK0][main]: [device 2] allocating 0.1016 GB, available 29.0203
[HCTR][10:35:34.365][DEBUG][RK0][main]: [device 3] allocating 0.1016 GB, available 29.0515
[HCTR][10:35:34.367][DEBUG][RK0][main]: [device 4] allocating 0.1016 GB, available 28.9460
[HCTR][10:35:34.368][DEBUG][RK0][main]: [device 5] allocating 0.1016 GB, available 29.0046
[HCTR][10:35:34.369][DEBUG][RK0][main]: [device 6] allocating 0.1016 GB, available 28.9890
[HCTR][10:35:34.371][DEBUG][RK0][main]: [device 7] allocating 0.1016 GB, available 29.1355
[HCTR][10:35:34.372][DEBUG][RK0][main]: [device 0] allocating 0.1016 GB, available 28.8269
[HCTR][10:35:34.373][DEBUG][RK0][main]: [device 1] allocating 0.1016 GB, available 28.9187
[HCTR][10:35:34.375][DEBUG][RK0][main]: [device 2] allocating 0.1016 GB, available 28.9187
[HCTR][10:35:34.376][DEBUG][RK0][main]: [device 3] allocating 0.1016 GB, available 28.9500
[HCTR][10:35:34.377][DEBUG][RK0][main]: [device 4] allocating 0.1016 GB, available 28.8445
[HCTR][10:35:34.379][DEBUG][RK0][main]: [device 5] allocating 0.1016 GB, available 28.9031
[HCTR][10:35:34.380][DEBUG][RK0][main]: [device 6] allocating 0.1016 GB, available 28.8875
[HCTR][10:35:34.381][DEBUG][RK0][main]: [device 7] allocating 0.1016 GB, available 29.0339
[HCTR][10:35:34.516][INFO][RK0][main]: Graph analysis to resolve tensor dependency
===================================================Model Compile===================================================
[HCTR][10:35:34.522][DEBUG][RK0][main]: [device 0] allocating 1.4051 GB, available 27.3660
[HCTR][10:35:34.525][DEBUG][RK0][main]: [device 1] allocating 1.4051 GB, available 27.4578
[HCTR][10:35:34.528][DEBUG][RK0][main]: [device 2] allocating 1.4051 GB, available 27.4578
[HCTR][10:35:34.531][DEBUG][RK0][main]: [device 3] allocating 1.4051 GB, available 27.4890
[HCTR][10:35:34.534][DEBUG][RK0][main]: [device 4] allocating 1.4051 GB, available 27.3835
[HCTR][10:35:34.538][DEBUG][RK0][main]: [device 5] allocating 1.4051 GB, available 27.4421
[HCTR][10:35:34.541][DEBUG][RK0][main]: [device 6] allocating 1.4051 GB, available 27.4265
[HCTR][10:35:34.544][DEBUG][RK0][main]: [device 7] allocating 1.4051 GB, available 27.5730
[HCTR][10:35:34.545][DEBUG][RK0][main]: [device 0] allocating 0.0088 GB, available 27.3562
[HCTR][10:35:34.546][DEBUG][RK0][main]: [device 1] allocating 0.0088 GB, available 27.4480
[HCTR][10:35:34.547][DEBUG][RK0][main]: [device 2] allocating 0.0088 GB, available 27.4480
[HCTR][10:35:34.548][DEBUG][RK0][main]: [device 3] allocating 0.0088 GB, available 27.4792
[HCTR][10:35:34.550][DEBUG][RK0][main]: [device 4] allocating 0.0088 GB, available 27.3738
[HCTR][10:35:34.551][DEBUG][RK0][main]: [device 5] allocating 0.0088 GB, available 27.4324
[HCTR][10:35:34.552][DEBUG][RK0][main]: [device 6] allocating 0.0088 GB, available 27.4167
[HCTR][10:35:34.553][DEBUG][RK0][main]: [device 7] allocating 0.0088 GB, available 27.5632
===================================================Model Summary===================================================
[HCTR][10:36:12.594][INFO][RK0][main]: Model structure on each GPU
Label Dense Sparse
label dense data0,data1,data2,data3,data4,data5,data6,data7,data8,data9,data10,data11,data12,data13,data14,data15,data16,data17,data18,data19,data20,data21,data22,data23,data24,data25
(8192,1) (8192,13)
——————————————————————————————————————————————————————————————————————————————————————————————————————————————————
Layer Type Input Name Output Name Output Shape
——————————————————————————————————————————————————————————————————————————————————————————————————————————————————
EmbeddingCollection0 data0 emb_vec0 (8192,1,128)
data1 emb_vec1 (8192,1,128)
data2 emb_vec2 (8192,1,128)
data3 emb_vec3 (8192,1,128)
data4 emb_vec4 (8192,1,128)
data5 emb_vec5 (8192,1,128)
data6 emb_vec6 (8192,1,128)
data7 emb_vec7 (8192,1,128)
data8 emb_vec8 (8192,1,128)
data9 emb_vec9 (8192,1,128)
data10 emb_vec10 (8192,1,128)
data11 emb_vec11 (8192,1,128)
data12 emb_vec12 (8192,1,128)
data13 emb_vec13 (8192,1,128)
data14 emb_vec14 (8192,1,128)
data15 emb_vec15 (8192,1,128)
data16 emb_vec16 (8192,1,128)
data17 emb_vec17 (8192,1,128)
data18 emb_vec18 (8192,1,128)
data19 emb_vec19 (8192,1,128)
data20 emb_vec20 (8192,1,128)
data21 emb_vec21 (8192,1,128)
data22 emb_vec22 (8192,1,128)
data23 emb_vec23 (8192,1,128)
data24 emb_vec24 (8192,1,128)
data25 emb_vec25 (8192,1,128)
------------------------------------------------------------------------------------------------------------------
Concat emb_vec0 sparse_embedding1 (8192,26,128)
emb_vec1
emb_vec2
emb_vec3
emb_vec4
emb_vec5
emb_vec6
emb_vec7
emb_vec8
emb_vec9
emb_vec10
emb_vec11
emb_vec12
emb_vec13
emb_vec14
emb_vec15
emb_vec16
emb_vec17
emb_vec18
emb_vec19
emb_vec20
emb_vec21
emb_vec22
emb_vec23
emb_vec24
emb_vec25
------------------------------------------------------------------------------------------------------------------
InnerProduct dense fc1 (8192,512)
------------------------------------------------------------------------------------------------------------------
ReLU fc1 relu1 (8192,512)
------------------------------------------------------------------------------------------------------------------
InnerProduct relu1 fc2 (8192,256)
------------------------------------------------------------------------------------------------------------------
ReLU fc2 relu2 (8192,256)
------------------------------------------------------------------------------------------------------------------
InnerProduct relu2 fc3 (8192,128)
------------------------------------------------------------------------------------------------------------------
ReLU fc3 relu3 (8192,128)
------------------------------------------------------------------------------------------------------------------
Interaction relu3 interaction1 (8192,480)
sparse_embedding1
------------------------------------------------------------------------------------------------------------------
InnerProduct interaction1 fc4 (8192,1024)
------------------------------------------------------------------------------------------------------------------
ReLU fc4 relu4 (8192,1024)
------------------------------------------------------------------------------------------------------------------
InnerProduct relu4 fc5 (8192,1024)
------------------------------------------------------------------------------------------------------------------
ReLU fc5 relu5 (8192,1024)
------------------------------------------------------------------------------------------------------------------
InnerProduct relu5 fc6 (8192,512)
------------------------------------------------------------------------------------------------------------------
ReLU fc6 relu6 (8192,512)
------------------------------------------------------------------------------------------------------------------
InnerProduct relu6 fc7 (8192,256)
------------------------------------------------------------------------------------------------------------------
ReLU fc7 relu7 (8192,256)
------------------------------------------------------------------------------------------------------------------
InnerProduct relu7 fc8 (8192,1)
------------------------------------------------------------------------------------------------------------------
BinaryCrossEntropyLoss fc8 loss
label
------------------------------------------------------------------------------------------------------------------
=====================================================Model Fit=====================================================
[HCTR][10:36:12.594][INFO][RK0][main]: Use non-epoch mode with number of iterations: 1000
[HCTR][10:36:12.594][INFO][RK0][main]: Training batchsize: 65536, evaluation batchsize: 65536
[HCTR][10:36:12.594][INFO][RK0][main]: Evaluation interval: 100, snapshot interval: 10000000
[HCTR][10:36:12.594][INFO][RK0][main]: Dense network trainable: True
[HCTR][10:36:12.594][INFO][RK0][main]: Use mixed precision: False, scaler: 1.000000, use cuda graph: True
[HCTR][10:36:12.594][INFO][RK0][main]: lr: 0.500000, warmup_steps: 300, end_lr: 0.000000
[HCTR][10:36:12.594][INFO][RK0][main]: decay_start: 0, decay_steps: 1, decay_power: 2.000000
[HCTR][10:36:12.594][INFO][RK0][main]: Training source file: ./deepfm_data_nvt/train/_file_list.txt
[HCTR][10:36:12.594][INFO][RK0][main]: Evaluation source file: ./deepfm_data_nvt/val/_file_list.txt
[HCTR][10:36:16.599][INFO][RK0][main]: Evaluation, AverageLoss: 0.144991
[HCTR][10:36:16.599][INFO][RK0][main]: Eval Time for 70 iters: 1.22035s
[HCTR][10:36:16.633][INFO][RK0][main]: Iter: 100 Time(100 iters): 4.03885s Loss: 0.144124 lr:0.168333
[HCTR][10:36:20.570][INFO][RK0][main]: Evaluation, AverageLoss: 0.144851
[HCTR][10:36:20.570][INFO][RK0][main]: Eval Time for 70 iters: 1.1863s
[HCTR][10:36:20.615][INFO][RK0][main]: Iter: 200 Time(100 iters): 3.98102s Loss: 0.145444 lr:0.335
[HCTR][10:36:24.540][INFO][RK0][main]: Evaluation, AverageLoss: 0.141821
[HCTR][10:36:24.540][INFO][RK0][main]: Eval Time for 70 iters: 1.18638s
[HCTR][10:36:24.580][INFO][RK0][main]: Iter: 300 Time(100 iters): 3.96441s Loss: 0.144249 lr:0.5
[HCTR][10:36:28.514][INFO][RK0][main]: Evaluation, AverageLoss: 0.139519
[HCTR][10:36:28.514][INFO][RK0][main]: Eval Time for 70 iters: 1.18203s
[HCTR][10:36:28.556][INFO][RK0][main]: Iter: 400 Time(100 iters): 3.97548s Loss: 0.140895 lr:0.5
[HCTR][10:36:32.490][INFO][RK0][main]: Evaluation, AverageLoss: 0.13942
[HCTR][10:36:32.491][INFO][RK0][main]: Eval Time for 70 iters: 1.19363s
[HCTR][10:36:32.533][INFO][RK0][main]: Iter: 500 Time(100 iters): 3.97628s Loss: 0.141202 lr:0.5
[HCTR][10:36:36.465][INFO][RK0][main]: Evaluation, AverageLoss: 0.13947
[HCTR][10:36:36.465][INFO][RK0][main]: Eval Time for 70 iters: 1.18342s
[HCTR][10:36:36.512][INFO][RK0][main]: Iter: 600 Time(100 iters): 3.97817s Loss: 0.136504 lr:0.5
[HCTR][10:36:40.440][INFO][RK0][main]: Evaluation, AverageLoss: 0.138534
[HCTR][10:36:40.440][INFO][RK0][main]: Eval Time for 70 iters: 1.19586s
[HCTR][10:36:40.476][INFO][RK0][main]: Iter: 700 Time(100 iters): 3.96355s Loss: 0.14067 lr:0.5
[HCTR][10:36:44.421][INFO][RK0][main]: Evaluation, AverageLoss: 0.138213
[HCTR][10:36:44.421][INFO][RK0][main]: Eval Time for 70 iters: 1.20188s
[HCTR][10:36:44.465][INFO][RK0][main]: Iter: 800 Time(100 iters): 3.98811s Loss: 0.142139 lr:0.5
[HCTR][10:36:48.390][INFO][RK0][main]: Evaluation, AverageLoss: 0.138044
[HCTR][10:36:48.390][INFO][RK0][main]: Eval Time for 70 iters: 1.19324s
[HCTR][10:36:48.427][INFO][RK0][main]: Iter: 900 Time(100 iters): 3.96149s Loss: 0.136835 lr:0.5
[HCTR][10:36:52.363][INFO][RK0][main]: Evaluation, AverageLoss: 0.137419
[HCTR][10:36:52.363][INFO][RK0][main]: Eval Time for 70 iters: 1.18732s
[HCTR][10:36:52.363][INFO][RK0][main]: Finish 1000 iterations with batchsize: 65536 in 39.77s.
Use Dynamic Hash Table with Round Robin Table Placement Strategy
Embedding collection supports user configure dynamic hash table so the table will support hash input key and the table size will grow when the table is full.
!python3 dlrm_train.py --shard_plan round_robin --use_dynamic_hash_table
HugeCTR Version: 23.2
====================================================Model Init=====================================================
[HCTR][10:29:29.407][WARNING][RK0][main]: The model name is not specified when creating the solver.
[HCTR][10:29:29.407][INFO][RK0][main]: Global seed is 1217153067
[HCTR][10:29:29.506][INFO][RK0][main]: Device to NUMA mapping:
GPU 0 -> node 0
GPU 1 -> node 0
GPU 2 -> node 0
GPU 3 -> node 0
GPU 4 -> node 1
GPU 5 -> node 1
GPU 6 -> node 1
GPU 7 -> node 1
[HCTR][10:29:40.485][WARNING][RK0][main]: Peer-to-peer access cannot be fully enabled.
[HCTR][10:29:40.485][DEBUG][RK0][main]: [device 0] allocating 0.0000 GB, available 30.4714
[HCTR][10:29:40.486][DEBUG][RK0][main]: [device 1] allocating 0.0000 GB, available 30.4441
[HCTR][10:29:40.486][DEBUG][RK0][main]: [device 2] allocating 0.0000 GB, available 30.5378
[HCTR][10:29:40.486][DEBUG][RK0][main]: [device 3] allocating 0.0000 GB, available 30.5339
[HCTR][10:29:40.486][DEBUG][RK0][main]: [device 4] allocating 0.0000 GB, available 30.4636
[HCTR][10:29:40.486][DEBUG][RK0][main]: [device 5] allocating 0.0000 GB, available 30.4480
[HCTR][10:29:40.486][DEBUG][RK0][main]: [device 6] allocating 0.0000 GB, available 30.4949
[HCTR][10:29:40.486][DEBUG][RK0][main]: [device 7] allocating 0.0000 GB, available 30.5183
[HCTR][10:29:40.486][INFO][RK0][main]: Start all2all warmup
[HCTR][10:29:40.651][INFO][RK0][main]: End all2all warmup
[HCTR][10:29:40.662][INFO][RK0][main]: Using All-reduce algorithm: NCCL
[HCTR][10:29:40.668][INFO][RK0][main]: Device 0: Tesla V100-SXM2-32GB
[HCTR][10:29:40.668][INFO][RK0][main]: Device 1: Tesla V100-SXM2-32GB
[HCTR][10:29:40.669][INFO][RK0][main]: Device 2: Tesla V100-SXM2-32GB
[HCTR][10:29:40.670][INFO][RK0][main]: Device 3: Tesla V100-SXM2-32GB
[HCTR][10:29:40.670][INFO][RK0][main]: Device 4: Tesla V100-SXM2-32GB
[HCTR][10:29:40.671][INFO][RK0][main]: Device 5: Tesla V100-SXM2-32GB
[HCTR][10:29:40.671][INFO][RK0][main]: Device 6: Tesla V100-SXM2-32GB
[HCTR][10:29:40.672][INFO][RK0][main]: Device 7: Tesla V100-SXM2-32GB
[HCTR][10:29:40.773][INFO][RK0][main]: eval source ./deepfm_data_nvt/val/_file_list.txt max_row_group_size 133678
[HCTR][10:29:40.862][INFO][RK0][main]: train source ./deepfm_data_nvt/train/_file_list.txt max_row_group_size 134102
[HCTR][10:29:40.866][INFO][RK0][main]: num of DataReader workers for train: 8
[HCTR][10:29:40.866][INFO][RK0][main]: num of DataReader workers for eval: 8
[HCTR][10:29:40.868][DEBUG][RK0][main]: [device 0] allocating 0.0804 GB, available 30.0457
[HCTR][10:29:40.869][DEBUG][RK0][main]: [device 1] allocating 0.0804 GB, available 30.0183
[HCTR][10:29:40.871][DEBUG][RK0][main]: [device 2] allocating 0.0804 GB, available 30.1121
[HCTR][10:29:40.872][DEBUG][RK0][main]: [device 3] allocating 0.0804 GB, available 30.1082
[HCTR][10:29:40.873][DEBUG][RK0][main]: [device 4] allocating 0.0804 GB, available 30.0378
[HCTR][10:29:40.875][DEBUG][RK0][main]: [device 5] allocating 0.0804 GB, available 30.0222
[HCTR][10:29:40.876][DEBUG][RK0][main]: [device 6] allocating 0.0804 GB, available 30.0691
[HCTR][10:29:40.878][DEBUG][RK0][main]: [device 7] allocating 0.0804 GB, available 30.0925
[HCTR][10:29:40.879][DEBUG][RK0][main]: [device 0] allocating 0.0804 GB, available 29.9636
[HCTR][10:29:40.881][DEBUG][RK0][main]: [device 1] allocating 0.0804 GB, available 29.9363
[HCTR][10:29:40.882][DEBUG][RK0][main]: [device 2] allocating 0.0804 GB, available 30.0300
[HCTR][10:29:40.884][DEBUG][RK0][main]: [device 3] allocating 0.0804 GB, available 30.0261
[HCTR][10:29:40.885][DEBUG][RK0][main]: [device 4] allocating 0.0804 GB, available 29.9558
[HCTR][10:29:40.886][DEBUG][RK0][main]: [device 5] allocating 0.0804 GB, available 29.9402
[HCTR][10:29:40.888][DEBUG][RK0][main]: [device 6] allocating 0.0804 GB, available 29.9871
[HCTR][10:29:40.889][DEBUG][RK0][main]: [device 7] allocating 0.0804 GB, available 30.0105
[HCTR][10:29:40.949][DEBUG][RK0][main]: [device 0] allocating 0.0000 GB, available 29.6863
[HCTR][10:29:41.055][DEBUG][RK0][main]: [device 1] allocating 0.0000 GB, available 29.6589
[HCTR][10:29:41.157][DEBUG][RK0][main]: [device 2] allocating 0.0000 GB, available 29.7527
[HCTR][10:29:41.250][DEBUG][RK0][main]: [device 3] allocating 0.0000 GB, available 29.7488
[HCTR][10:29:41.333][DEBUG][RK0][main]: [device 4] allocating 0.0000 GB, available 29.6785
[HCTR][10:29:41.419][DEBUG][RK0][main]: [device 5] allocating 0.0000 GB, available 29.6628
[HCTR][10:29:41.525][DEBUG][RK0][main]: [device 6] allocating 0.0000 GB, available 29.7097
[HCTR][10:29:41.619][DEBUG][RK0][main]: [device 7] allocating 0.0000 GB, available 29.7332
[HCTR][10:29:41.780][DEBUG][RK0][main]: [device 0] allocating 0.0000 GB, available 29.4089
[HCTR][10:29:41.866][DEBUG][RK0][main]: [device 1] allocating 0.0000 GB, available 29.3816
[HCTR][10:29:41.953][DEBUG][RK0][main]: [device 2] allocating 0.0000 GB, available 29.4753
[HCTR][10:29:42.059][DEBUG][RK0][main]: [device 3] allocating 0.0000 GB, available 29.4714
[HCTR][10:29:42.150][DEBUG][RK0][main]: [device 4] allocating 0.0000 GB, available 29.4011
[HCTR][10:29:42.245][DEBUG][RK0][main]: [device 5] allocating 0.0000 GB, available 29.3855
[HCTR][10:29:42.332][DEBUG][RK0][main]: [device 6] allocating 0.0000 GB, available 29.4324
[HCTR][10:29:42.434][DEBUG][RK0][main]: [device 7] allocating 0.0000 GB, available 29.4558
[HCTR][10:29:42.537][INFO][RK0][main]: Vocabulary size: 0
[HCTR][10:29:42.786][DEBUG][RK0][main]: [device 0] allocating 0.1016 GB, available 28.8152
[HCTR][10:29:42.787][DEBUG][RK0][main]: [device 1] allocating 0.1016 GB, available 28.7878
[HCTR][10:29:42.789][DEBUG][RK0][main]: [device 2] allocating 0.1016 GB, available 28.9441
[HCTR][10:29:42.790][DEBUG][RK0][main]: [device 3] allocating 0.1016 GB, available 28.9402
[HCTR][10:29:42.791][DEBUG][RK0][main]: [device 4] allocating 0.1016 GB, available 28.8699
[HCTR][10:29:42.793][DEBUG][RK0][main]: [device 5] allocating 0.1016 GB, available 28.8542
[HCTR][10:29:42.794][DEBUG][RK0][main]: [device 6] allocating 0.1016 GB, available 28.9011
[HCTR][10:29:42.795][DEBUG][RK0][main]: [device 7] allocating 0.1016 GB, available 28.9246
[HCTR][10:29:42.797][DEBUG][RK0][main]: [device 0] allocating 0.1016 GB, available 28.7136
[HCTR][10:29:42.798][DEBUG][RK0][main]: [device 1] allocating 0.1016 GB, available 28.6863
[HCTR][10:29:42.799][DEBUG][RK0][main]: [device 2] allocating 0.1016 GB, available 28.8425
[HCTR][10:29:42.801][DEBUG][RK0][main]: [device 3] allocating 0.1016 GB, available 28.8386
[HCTR][10:29:42.802][DEBUG][RK0][main]: [device 4] allocating 0.1016 GB, available 28.7683
[HCTR][10:29:42.803][DEBUG][RK0][main]: [device 5] allocating 0.1016 GB, available 28.7527
[HCTR][10:29:42.805][DEBUG][RK0][main]: [device 6] allocating 0.1016 GB, available 28.7996
[HCTR][10:29:42.806][DEBUG][RK0][main]: [device 7] allocating 0.1016 GB, available 28.8230
[HCTR][10:29:42.934][INFO][RK0][main]: Graph analysis to resolve tensor dependency
===================================================Model Compile===================================================
[HCTR][10:29:42.940][DEBUG][RK0][main]: [device 0] allocating 1.4051 GB, available 27.2527
[HCTR][10:29:42.943][DEBUG][RK0][main]: [device 1] allocating 1.4051 GB, available 27.2253
[HCTR][10:29:42.946][DEBUG][RK0][main]: [device 2] allocating 1.4051 GB, available 27.3816
[HCTR][10:29:42.949][DEBUG][RK0][main]: [device 3] allocating 1.4051 GB, available 27.3777
[HCTR][10:29:42.952][DEBUG][RK0][main]: [device 4] allocating 1.4051 GB, available 27.3074
[HCTR][10:29:42.955][DEBUG][RK0][main]: [device 5] allocating 1.4051 GB, available 27.2917
[HCTR][10:29:42.958][DEBUG][RK0][main]: [device 6] allocating 1.4051 GB, available 27.3386
[HCTR][10:29:42.961][DEBUG][RK0][main]: [device 7] allocating 1.4051 GB, available 27.3621
[HCTR][10:29:42.962][DEBUG][RK0][main]: [device 0] allocating 0.0088 GB, available 27.2429
[HCTR][10:29:42.964][DEBUG][RK0][main]: [device 1] allocating 0.0088 GB, available 27.2156
[HCTR][10:29:42.965][DEBUG][RK0][main]: [device 2] allocating 0.0088 GB, available 27.3718
[HCTR][10:29:42.966][DEBUG][RK0][main]: [device 3] allocating 0.0088 GB, available 27.3679
[HCTR][10:29:42.967][DEBUG][RK0][main]: [device 4] allocating 0.0088 GB, available 27.2976
[HCTR][10:29:42.968][DEBUG][RK0][main]: [device 5] allocating 0.0088 GB, available 27.2820
[HCTR][10:29:42.969][DEBUG][RK0][main]: [device 6] allocating 0.0088 GB, available 27.3289
[HCTR][10:29:42.970][DEBUG][RK0][main]: [device 7] allocating 0.0088 GB, available 27.3523
===================================================Model Summary===================================================
[HCTR][10:30:20.859][INFO][RK0][main]: Model structure on each GPU
Label Dense Sparse
label dense data0,data1,data2,data3,data4,data5,data6,data7,data8,data9,data10,data11,data12,data13,data14,data15,data16,data17,data18,data19,data20,data21,data22,data23,data24,data25
(8192,1) (8192,13)
——————————————————————————————————————————————————————————————————————————————————————————————————————————————————
Layer Type Input Name Output Name Output Shape
——————————————————————————————————————————————————————————————————————————————————————————————————————————————————
EmbeddingCollection0 data0 emb_vec0 (8192,1,128)
data1 emb_vec1 (8192,1,128)
data2 emb_vec2 (8192,1,128)
data3 emb_vec3 (8192,1,128)
data4 emb_vec4 (8192,1,128)
data5 emb_vec5 (8192,1,128)
data6 emb_vec6 (8192,1,128)
data7 emb_vec7 (8192,1,128)
data8 emb_vec8 (8192,1,128)
data9 emb_vec9 (8192,1,128)
data10 emb_vec10 (8192,1,128)
data11 emb_vec11 (8192,1,128)
data12 emb_vec12 (8192,1,128)
data13 emb_vec13 (8192,1,128)
data14 emb_vec14 (8192,1,128)
data15 emb_vec15 (8192,1,128)
data16 emb_vec16 (8192,1,128)
data17 emb_vec17 (8192,1,128)
data18 emb_vec18 (8192,1,128)
data19 emb_vec19 (8192,1,128)
data20 emb_vec20 (8192,1,128)
data21 emb_vec21 (8192,1,128)
data22 emb_vec22 (8192,1,128)
data23 emb_vec23 (8192,1,128)
data24 emb_vec24 (8192,1,128)
data25 emb_vec25 (8192,1,128)
------------------------------------------------------------------------------------------------------------------
Concat emb_vec0 sparse_embedding1 (8192,26,128)
emb_vec1
emb_vec2
emb_vec3
emb_vec4
emb_vec5
emb_vec6
emb_vec7
emb_vec8
emb_vec9
emb_vec10
emb_vec11
emb_vec12
emb_vec13
emb_vec14
emb_vec15
emb_vec16
emb_vec17
emb_vec18
emb_vec19
emb_vec20
emb_vec21
emb_vec22
emb_vec23
emb_vec24
emb_vec25
------------------------------------------------------------------------------------------------------------------
InnerProduct dense fc1 (8192,512)
------------------------------------------------------------------------------------------------------------------
ReLU fc1 relu1 (8192,512)
------------------------------------------------------------------------------------------------------------------
InnerProduct relu1 fc2 (8192,256)
------------------------------------------------------------------------------------------------------------------
ReLU fc2 relu2 (8192,256)
------------------------------------------------------------------------------------------------------------------
InnerProduct relu2 fc3 (8192,128)
------------------------------------------------------------------------------------------------------------------
ReLU fc3 relu3 (8192,128)
------------------------------------------------------------------------------------------------------------------
Interaction relu3 interaction1 (8192,480)
sparse_embedding1
------------------------------------------------------------------------------------------------------------------
InnerProduct interaction1 fc4 (8192,1024)
------------------------------------------------------------------------------------------------------------------
ReLU fc4 relu4 (8192,1024)
------------------------------------------------------------------------------------------------------------------
InnerProduct relu4 fc5 (8192,1024)
------------------------------------------------------------------------------------------------------------------
ReLU fc5 relu5 (8192,1024)
------------------------------------------------------------------------------------------------------------------
InnerProduct relu5 fc6 (8192,512)
------------------------------------------------------------------------------------------------------------------
ReLU fc6 relu6 (8192,512)
------------------------------------------------------------------------------------------------------------------
InnerProduct relu6 fc7 (8192,256)
------------------------------------------------------------------------------------------------------------------
ReLU fc7 relu7 (8192,256)
------------------------------------------------------------------------------------------------------------------
InnerProduct relu7 fc8 (8192,1)
------------------------------------------------------------------------------------------------------------------
BinaryCrossEntropyLoss fc8 loss
label
------------------------------------------------------------------------------------------------------------------
=====================================================Model Fit=====================================================
[HCTR][10:30:20.860][INFO][RK0][main]: Use non-epoch mode with number of iterations: 1000
[HCTR][10:30:20.860][INFO][RK0][main]: Training batchsize: 65536, evaluation batchsize: 65536
[HCTR][10:30:20.860][INFO][RK0][main]: Evaluation interval: 100, snapshot interval: 10000000
[HCTR][10:30:20.860][INFO][RK0][main]: Dense network trainable: True
[HCTR][10:30:20.860][INFO][RK0][main]: Use mixed precision: False, scaler: 1.000000, use cuda graph: True
[HCTR][10:30:20.860][INFO][RK0][main]: lr: 0.500000, warmup_steps: 300, end_lr: 0.000000
[HCTR][10:30:20.860][INFO][RK0][main]: decay_start: 0, decay_steps: 1, decay_power: 2.000000
[HCTR][10:30:20.860][INFO][RK0][main]: Training source file: ./deepfm_data_nvt/train/_file_list.txt
[HCTR][10:30:20.860][INFO][RK0][main]: Evaluation source file: ./deepfm_data_nvt/val/_file_list.txt
static_map allocated, size=553648128
static_map allocated, size=553648128
static_map allocated, size=553648128
static_map allocated, size=553648128
static_map allocated, size=553648128
static_map allocated, size=553648128
static_map allocated, size=553648128
static_map allocated, size=553648128
static_map allocated, size=553648128
static_map allocated, size=553648128
static_map allocated, size=553648128
static_map allocated, size=553648128
static_map allocated, size=553648128
static_map allocated, size=553648128
static_map allocated, size=553648128
static_map allocated, size=553648128
static_map allocated, size=553648128
static_map allocated, size=553648128
static_map allocated, size=553648128
static_map allocated, size=553648128
static_map allocated, size=553648128
static_map allocated, size=553648128
static_map allocated, size=553648128
static_map allocated, size=553648128
static_map allocated, size=553648128
static_map allocated, size=553648128
[HCTR][10:30:26.070][INFO][RK0][main]: Evaluation, AverageLoss: 0.142151
[HCTR][10:30:26.070][INFO][RK0][main]: Eval Time for 70 iters: 1.53912s
[HCTR][10:30:26.123][INFO][RK0][main]: Iter: 100 Time(100 iters): 5.26107s Loss: 0.141023 lr:0.168333
[HCTR][10:30:31.183][INFO][RK0][main]: Evaluation, AverageLoss: 0.141078
[HCTR][10:30:31.183][INFO][RK0][main]: Eval Time for 70 iters: 1.57008s
[HCTR][10:30:31.225][INFO][RK0][main]: Iter: 200 Time(100 iters): 5.10267s Loss: 0.141925 lr:0.335
[HCTR][10:30:36.309][INFO][RK0][main]: Evaluation, AverageLoss: 0.140561
[HCTR][10:30:36.309][INFO][RK0][main]: Eval Time for 70 iters: 1.55499s
[HCTR][10:30:36.362][INFO][RK0][main]: Iter: 300 Time(100 iters): 5.13614s Loss: 0.14338 lr:0.5
[HCTR][10:30:41.415][INFO][RK0][main]: Evaluation, AverageLoss: 0.139972
[HCTR][10:30:41.415][INFO][RK0][main]: Eval Time for 70 iters: 1.54929s
[HCTR][10:30:41.464][INFO][RK0][main]: Iter: 400 Time(100 iters): 5.10246s Loss: 0.141379 lr:0.5
[HCTR][10:30:46.534][INFO][RK0][main]: Evaluation, AverageLoss: 0.139553
[HCTR][10:30:46.534][INFO][RK0][main]: Eval Time for 70 iters: 1.56729s
[HCTR][10:30:46.582][INFO][RK0][main]: Iter: 500 Time(100 iters): 5.11698s Loss: 0.141421 lr:0.5
[HCTR][10:30:51.642][INFO][RK0][main]: Evaluation, AverageLoss: 0.139362
[HCTR][10:30:51.642][INFO][RK0][main]: Eval Time for 70 iters: 1.56153s
[HCTR][10:30:51.696][INFO][RK0][main]: Iter: 600 Time(100 iters): 5.11376s Loss: 0.136499 lr:0.5
[HCTR][10:30:56.755][INFO][RK0][main]: Evaluation, AverageLoss: 0.138972
[HCTR][10:30:56.755][INFO][RK0][main]: Eval Time for 70 iters: 1.60721s
[HCTR][10:30:56.811][INFO][RK0][main]: Iter: 700 Time(100 iters): 5.11548s Loss: 0.141355 lr:0.5
[HCTR][10:31:01.873][INFO][RK0][main]: Evaluation, AverageLoss: 0.138726
[HCTR][10:31:01.873][INFO][RK0][main]: Eval Time for 70 iters: 1.56329s
[HCTR][10:31:01.913][INFO][RK0][main]: Iter: 800 Time(100 iters): 5.10124s Loss: 0.142614 lr:0.5
[HCTR][10:31:07.016][INFO][RK0][main]: Evaluation, AverageLoss: 0.139617
[HCTR][10:31:07.016][INFO][RK0][main]: Eval Time for 70 iters: 1.5483s
[HCTR][10:31:07.063][INFO][RK0][main]: Iter: 900 Time(100 iters): 5.14957s Loss: 0.138442 lr:0.5
[HCTR][10:31:12.147][INFO][RK0][main]: Evaluation, AverageLoss: 0.138159
[HCTR][10:31:12.147][INFO][RK0][main]: Eval Time for 70 iters: 1.57499s
[HCTR][10:31:12.147][INFO][RK0][main]: Finish 1000 iterations with batchsize: 65536 in 51.29s.