# Copyright 2021 NVIDIA Corporation. All Rights Reserved.
#
# Licensed under the Apache License, Version 2.0 (the "License");
# you may not use this file except in compliance with the License.
# You may obtain a copy of the License at
#
# http://www.apache.org/licenses/LICENSE-2.0
#
# Unless required by applicable law or agreed to in writing, software
# distributed under the License is distributed on an "AS IS" BASIS,
# WITHOUT WARRANTIES OR CONDITIONS OF ANY KIND, either express or implied.
# See the License for the specific language governing permissions and
# limitations under the License.
# ==============================================================================
# Each user is responsible for checking the content of datasets and the
# applicable licenses and determining if suitable for the intended use.
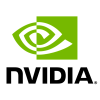
HugeCTR Wide and Deep Model with Criteo
Overview
In this notebook, we provide a tutorial that shows how to train a wide and deep model using the high-level Python API from HugeCTR on the original Criteo dataset as training data. We show how to produce prediction results based on different types of local database.
Setup HugeCTR
To setup the environment, refer to HugeCTR Example Notebooks and follow the instructions there before running the following.
Dataset Preprocessing
Generate training and validation data folders
# define some data folder to store the original and preprocessed data
# Standard Libraries
import os
from time import time
import re
import shutil
import glob
import warnings
BASE_DIR = "/wdl_train"
train_path = os.path.join(BASE_DIR, "train")
val_path = os.path.join(BASE_DIR, "val")
CUDA_VISIBLE_DEVICES = os.environ.get("CUDA_VISIBLE_DEVICES", "0")
n_workers = len(CUDA_VISIBLE_DEVICES.split(","))
frac_size = 0.15
allow_multi_gpu = False
use_rmm_pool = False
max_day = None # (Optional) -- Limit the dataset to day 0-max_day for debugging
if os.path.isdir(train_path):
shutil.rmtree(train_path)
os.makedirs(train_path)
if os.path.isdir(val_path):
shutil.rmtree(val_path)
os.makedirs(val_path)
Download the original Criteo dataset
!apt-get install wget
!wget -P $train_path https://storage.googleapis.com/criteo-cail-datasets/day_0.gz
Split the dataset into training and validation.
#!gzip -d -c $train_path/day_0.gz > day_0
!head -n 10000000 day_0 > $train_path/train.txt
!tail -n 2000000 day_0 > $val_path/test.txt
Preprocessing with NVTabular
%%writefile '/wdl_train/preprocess.py'
import warnings
warnings.filterwarnings("ignore")
warnings.simplefilter("ignore", UserWarning)
import os
import sys
import argparse
import glob
import time
import numpy as np
import shutil
import dask_cudf
from dask_cuda import LocalCUDACluster
from dask.distributed import Client
import nvtabular as nvt
from merlin.core.compat import device_mem_size
from nvtabular.ops import (
Categorify,
Clip,
FillMissing,
Normalize,
get_embedding_sizes,
)
# %load_ext memory_profiler
import logging
logging.basicConfig(format="%(asctime)s %(message)s")
logging.root.setLevel(logging.NOTSET)
logging.getLogger("numba").setLevel(logging.WARNING)
logging.getLogger("asyncio").setLevel(logging.WARNING)
# define dataset schema
CATEGORICAL_COLUMNS = ["C" + str(x) for x in range(1, 27)]
CONTINUOUS_COLUMNS = ["I" + str(x) for x in range(1, 14)]
LABEL_COLUMNS = ["label"]
COLUMNS = LABEL_COLUMNS + CONTINUOUS_COLUMNS + CATEGORICAL_COLUMNS
# /samples/criteo mode doesn't have dense features
criteo_COLUMN = LABEL_COLUMNS + CATEGORICAL_COLUMNS
# For new feature cross columns
CROSS_COLUMNS = []
NUM_INTEGER_COLUMNS = 13
NUM_CATEGORICAL_COLUMNS = 26
NUM_TOTAL_COLUMNS = 1 + NUM_INTEGER_COLUMNS + NUM_CATEGORICAL_COLUMNS
# compute the partition size with GB
def bytesto(bytes, to, bsize=1024):
a = {"k": 1, "m": 2, "g": 3, "t": 4, "p": 5, "e": 6}
r = float(bytes)
return bytes / (bsize ** a[to])
# process the data with NVTabular
def process_NVT(args):
if args.feature_cross_list:
feature_pairs = [pair.split("_") for pair in args.feature_cross_list.split(",")]
for pair in feature_pairs:
CROSS_COLUMNS.append(pair[0] + "_" + pair[1])
logging.info("NVTabular processing")
train_input = os.path.join(args.data_path, "train/train.txt")
val_input = os.path.join(args.data_path, "val/test.txt")
PREPROCESS_DIR_temp_train = os.path.join(args.out_path, "train/temp-parquet-after-conversion")
PREPROCESS_DIR_temp_val = os.path.join(args.out_path, "val/temp-parquet-after-conversion")
PREPROCESS_DIR_temp = [PREPROCESS_DIR_temp_train, PREPROCESS_DIR_temp_val]
train_output = os.path.join(args.out_path, "train")
val_output = os.path.join(args.out_path, "val")
# Make sure we have a clean parquet space for cudf conversion
for one_path in PREPROCESS_DIR_temp:
if os.path.exists(one_path):
shutil.rmtree(one_path)
os.mkdir(one_path)
## Get Dask Client
# Deploy a Single-Machine Multi-GPU Cluster
device_size = device_mem_size(kind="total")
device_pool_size = int(args.device_pool_frac * device_size)
cluster = None
if args.protocol == "ucx":
UCX_TLS = os.environ.get("UCX_TLS", "tcp,cuda_copy,cuda_ipc,sockcm")
os.environ["UCX_TLS"] = UCX_TLS
cluster = LocalCUDACluster(
protocol=args.protocol,
CUDA_VISIBLE_DEVICES=args.devices,
n_workers=len(args.devices.split(",")),
device_memory_limit=int(device_size * args.device_limit_frac),
dashboard_address=":" + args.dashboard_port,
rmm_pool_size=(device_pool_size // 256) * 256,
)
else:
cluster = LocalCUDACluster(
protocol=args.protocol,
n_workers=len(args.devices.split(",")),
CUDA_VISIBLE_DEVICES=args.devices,
device_memory_limit=int(device_size * args.device_limit_frac),
dashboard_address=":" + args.dashboard_port,
rmm_pool_size=(device_pool_size // 256) * 256,
)
# Create the distributed client
if cluster:
client = Client(cluster)
else:
client = Client(processes=False)
# calculate the total processing time
runtime = time.time()
# test dataset without the label feature
if args.dataset_type == "test":
global LABEL_COLUMNS
LABEL_COLUMNS = []
##-----------------------------------##
# Dask rapids converts txt to parquet
# Dask cudf dataframe = ddf
## train/valid txt to parquet
train_valid_paths = [
(train_input, PREPROCESS_DIR_temp_train),
(val_input, PREPROCESS_DIR_temp_val),
]
for input, temp_output in train_valid_paths:
ddf = dask_cudf.read_csv(
input, sep="\t", names=LABEL_COLUMNS + CONTINUOUS_COLUMNS + CATEGORICAL_COLUMNS
)
if args.feature_cross_list:
feature_pairs = [pair.split("_") for pair in args.feature_cross_list.split(",")]
for pair in args.feature_cross_list.split(","):
feature_pair = pair.split("_")
ddf[pair] = ddf[feature_pair[0]] + ddf[feature_pair[1]]
## Convert label col to FP32
if args.parquet_format and args.dataset_type == "train":
ddf["label"] = ddf["label"].astype("float32")
# Save it as parquet format for better memory usage
ddf.to_parquet(temp_output, header=True)
##-----------------------------------##
COLUMNS = LABEL_COLUMNS + CONTINUOUS_COLUMNS + CROSS_COLUMNS + CATEGORICAL_COLUMNS
train_paths = glob.glob(os.path.join(PREPROCESS_DIR_temp_train, "*.parquet"))
valid_paths = glob.glob(os.path.join(PREPROCESS_DIR_temp_val, "*.parquet"))
categorify_op = Categorify(freq_threshold=args.freq_limit)
cat_features = CATEGORICAL_COLUMNS >> categorify_op
cont_features = CONTINUOUS_COLUMNS >> FillMissing() >> Clip(min_value=0) >> Normalize()
cross_cat_op = Categorify(freq_threshold=args.freq_limit)
features = LABEL_COLUMNS
if args.criteo_mode == 0:
features += cont_features
for pair in args.feature_cross_list.split(","):
features += [pair] >> cross_cat_op
features += cat_features
workflow = nvt.Workflow(features, client=client)
logging.info("Preprocessing")
output_format = "hugectr"
if args.parquet_format:
output_format = "parquet"
# just for /samples/criteo model
train_ds_iterator = nvt.Dataset(
train_paths, engine="parquet", part_size=int(args.part_mem_frac * device_size)
)
valid_ds_iterator = nvt.Dataset(
valid_paths, engine="parquet", part_size=int(args.part_mem_frac * device_size)
)
shuffle = None
if args.shuffle == "PER_WORKER":
shuffle = nvt.io.Shuffle.PER_WORKER
elif args.shuffle == "PER_PARTITION":
shuffle = nvt.io.Shuffle.PER_PARTITION
logging.info("Train Datasets Preprocessing.....")
dict_dtypes = {}
for col in CATEGORICAL_COLUMNS:
dict_dtypes[col] = np.int64
if not args.criteo_mode:
for col in CONTINUOUS_COLUMNS:
dict_dtypes[col] = np.float32
for col in CROSS_COLUMNS:
dict_dtypes[col] = np.int64
for col in LABEL_COLUMNS:
dict_dtypes[col] = np.float32
conts = CONTINUOUS_COLUMNS if not args.criteo_mode else []
workflow.fit(train_ds_iterator)
if output_format == "hugectr":
workflow.transform(train_ds_iterator).to_hugectr(
cats=CROSS_COLUMNS + CATEGORICAL_COLUMNS,
conts=conts,
labels=LABEL_COLUMNS,
output_path=train_output,
shuffle=shuffle,
out_files_per_proc=args.out_files_per_proc,
num_threads=args.num_io_threads,
)
else:
workflow.transform(train_ds_iterator).to_parquet(
output_path=train_output,
dtypes=dict_dtypes,
cats=CROSS_COLUMNS + CATEGORICAL_COLUMNS,
conts=conts,
labels=LABEL_COLUMNS,
shuffle=shuffle,
out_files_per_proc=args.out_files_per_proc,
num_threads=args.num_io_threads,
)
logging.info("Valid Datasets Preprocessing.....")
if output_format == "hugectr":
workflow.transform(valid_ds_iterator).to_hugectr(
cats=CATEGORICAL_COLUMNS + CROSS_COLUMNS,
conts=conts,
labels=LABEL_COLUMNS,
output_path=val_output,
shuffle=shuffle,
out_files_per_proc=args.out_files_per_proc,
num_threads=args.num_io_threads,
)
else:
workflow.transform(valid_ds_iterator).to_parquet(
output_path=val_output,
dtypes=dict_dtypes,
cats=CATEGORICAL_COLUMNS + CROSS_COLUMNS,
conts=conts,
labels=LABEL_COLUMNS,
shuffle=shuffle,
out_files_per_proc=args.out_files_per_proc,
num_threads=args.num_io_threads,
)
embeddings_dict_cat = categorify_op.get_embedding_sizes(CATEGORICAL_COLUMNS)
embeddings_dict_cross = cross_cat_op.get_embedding_sizes(CROSS_COLUMNS)
embeddings = [embeddings_dict_cross[c][0] for c in CROSS_COLUMNS] + [
embeddings_dict_cat[c][0] for c in CATEGORICAL_COLUMNS
]
print("Slot size array is: ", embeddings)
##--------------------##
logging.info("NVTabular processing done")
runtime = time.time() - runtime
print("\nDask-NVTabular Criteo Preprocessing")
print("--------------------------------------")
print(f"data_path | {args.data_path}")
print(f"output_path | {args.out_path}")
print(f"partition size | {'%.2f GB'%bytesto(int(args.part_mem_frac * device_size),'g')}")
print(f"protocol | {args.protocol}")
print(f"device(s) | {args.devices}")
print(f"rmm-pool-frac | {(args.device_pool_frac)}")
print(f"out-files-per-proc | {args.out_files_per_proc}")
print(f"num_io_threads | {args.num_io_threads}")
print(f"shuffle | {args.shuffle}")
print("======================================")
print(f"Runtime[s] | {runtime}")
print("======================================\n")
def parse_args():
parser = argparse.ArgumentParser(description=("Multi-GPU Criteo Preprocessing"))
#
# System Options
#
parser.add_argument("--data_path", type=str, help="Input dataset path (Required)")
parser.add_argument("--out_path", type=str, help="Directory path to write output (Required)")
parser.add_argument(
"-d",
"--devices",
default=os.environ.get("CUDA_VISIBLE_DEVICES", "0"),
type=str,
help='Comma-separated list of visible devices (e.g. "0,1,2,3"). ',
)
parser.add_argument(
"-p",
"--protocol",
choices=["tcp", "ucx"],
default="tcp",
type=str,
help="Communication protocol to use (Default 'tcp')",
)
parser.add_argument(
"--device_limit_frac",
default=0.5,
type=float,
help="Worker device-memory limit as a fraction of GPU capacity (Default 0.8). ",
)
parser.add_argument(
"--device_pool_frac",
default=0.9,
type=float,
help="RMM pool size for each worker as a fraction of GPU capacity (Default 0.9). "
"The RMM pool frac is the same for all GPUs, make sure each one has enough memory size",
)
parser.add_argument(
"--num_io_threads",
default=0,
type=int,
help="Number of threads to use when writing output data (Default 0). "
"If 0 is specified, multi-threading will not be used for IO.",
)
#
# Data-Decomposition Parameters
#
parser.add_argument(
"--part_mem_frac",
default=0.125,
type=float,
help="Maximum size desired for dataset partitions as a fraction "
"of GPU capacity (Default 0.125)",
)
parser.add_argument(
"--out_files_per_proc",
default=8,
type=int,
help="Number of output files to write on each worker (Default 8)",
)
#
# Preprocessing Options
#
parser.add_argument(
"-f",
"--freq_limit",
default=0,
type=int,
help="Frequency limit for categorical encoding (Default 0)",
)
parser.add_argument(
"-s",
"--shuffle",
choices=["PER_WORKER", "PER_PARTITION", "NONE"],
default="PER_PARTITION",
help="Shuffle algorithm to use when writing output data to disk (Default PER_PARTITION)",
)
parser.add_argument(
"--feature_cross_list",
default=None,
type=str,
help="List of feature crossing cols (e.g. C1_C2, C3_C4)",
)
#
# Diagnostics Options
#
parser.add_argument(
"--profile",
metavar="PATH",
default=None,
type=str,
help="Specify a file path to export a Dask profile report (E.g. dask-report.html)."
"If this option is excluded from the command, not profile will be exported",
)
parser.add_argument(
"--dashboard_port",
default="8787",
type=str,
help="Specify the desired port of Dask's diagnostics-dashboard (Default `3787`). "
"The dashboard will be hosted at http://<IP>:<PORT>/status",
)
parser.add_argument("--criteo_mode", type=int, default=0)
parser.add_argument("--parquet_format", type=int, default=1)
parser.add_argument("--dataset_type", type=str, default="train")
args = parser.parse_args()
args.n_workers = len(args.devices.split(","))
return args
if __name__ == "__main__":
args = parse_args()
process_NVT(args)
Overwriting /wdl_train/preprocess.py
!python3 /wdl_train/preprocess.py --data_path /wdl_train/ \
--out_path /wdl_train/ --freq_limit 6 --feature_cross_list C1_C2,C3_C4 \
--device_pool_frac 0.5 --devices '0' --num_io_threads 2
2023-05-26 04:30:43,128 NVTabular processing
2023-05-26 04:30:45,000 - distributed.preloading - INFO - Creating preload: dask_cuda.initialize
2023-05-26 04:30:45,000 - distributed.preloading - INFO - Import preload module: dask_cuda.initialize
2023-05-26 04:30:53,847 Preprocessing
2023-05-26 04:30:54,160 Train Datasets Preprocessing.....
2023-05-26 04:31:14,725 Valid Datasets Preprocessing.....
Slot size array is: [62962, 127889, 56869, 12448, 11969, 6832, 18364, 4, 5960, 1170, 43, 57084, 29015, 33861, 11, 1956, 5598, 55, 4, 913, 15, 56488, 48591, 57463, 26037, 7790, 58, 34]
2023-05-26 04:31:18,677 NVTabular processing done
Dask-NVTabular Criteo Preprocessing
--------------------------------------
data_path | /wdl_train/
output_path | /wdl_train/
partition size | 3.97 GB
protocol | tcp
device(s) | 0
rmm-pool-frac | 0.5
out-files-per-proc | 8
num_io_threads | 2
shuffle | PER_PARTITION
======================================
Runtime[s] | 32.06131315231323
======================================
2023-05-26 04:31:18,682 Attempted to close worker that is already Status.closing. Reason: worker-handle-scheduler-connection-broken
2023-05-26 04:31:18,683 Attempted to close worker that is already Status.closed. Reason: worker-close
Check the preprocessed training data
!ls -ll /wdl_train/train
total 3103496
-rw-r--r-- 1 root root 258 May 26 04:31 _file_list.txt
-rw-r--r-- 1 root root 271567 May 26 04:31 _metadata
-rw-r--r-- 1 root root 1887 May 26 04:31 _metadata.json
-rw-r--r-- 1 root root 79777109 May 26 04:31 part_0.parquet
-rw-r--r-- 1 root root 79821862 May 26 04:31 part_1.parquet
-rw-r--r-- 1 root root 79946970 May 26 04:31 part_2.parquet
-rw-r--r-- 1 root root 79783392 May 26 04:31 part_3.parquet
-rw-r--r-- 1 root root 79875076 May 26 04:31 part_4.parquet
-rw-r--r-- 1 root root 79844899 May 26 04:31 part_5.parquet
-rw-r--r-- 1 root root 79876452 May 26 04:31 part_6.parquet
-rw-r--r-- 1 root root 79767942 May 26 04:31 part_7.parquet
-rw-r--r-- 1 root root 31277 May 26 04:31 schema.pbtxt
drwxr-xr-x 2 root root 226 May 26 04:30 temp-parquet-after-conversion
-rw-r--r-- 1 root root 2538954147 May 26 04:30 train.txt
WDL Model Training
%%writefile './model.py'
import hugectr
#from mpi4py import MPI
solver = hugectr.CreateSolver(max_eval_batches = 4000,
batchsize_eval = 2720,
batchsize = 2720,
lr = 0.001,
vvgpu = [[1]],
repeat_dataset = True,
i64_input_key = True)
reader = hugectr.DataReaderParams(data_reader_type = hugectr.DataReaderType_t.Parquet,
source = ["/wdl_train/train/_file_list.txt"],
eval_source = "/wdl_train/val/_file_list.txt",
check_type = hugectr.Check_t.Non,
slot_size_array = [278018, 415262, 249058, 19561, 14212, 6890, 18592, 4, 6356, 1254, 52, 226170, 80508, 72308, 11, 2169, 7597, 61, 4, 923, 15, 249619, 168974, 243480, 68212, 9169, 75, 34])
optimizer = hugectr.CreateOptimizer(optimizer_type = hugectr.Optimizer_t.Adam,
update_type = hugectr.Update_t.Global,
beta1 = 0.9,
beta2 = 0.999,
epsilon = 0.0000001)
model = hugectr.Model(solver, reader, optimizer)
model.add(hugectr.Input(label_dim = 1, label_name = "label",
dense_dim = 13, dense_name = "dense",
data_reader_sparse_param_array =
[hugectr.DataReaderSparseParam("wide_data", 1, True, 2),
hugectr.DataReaderSparseParam("deep_data", 2, False, 26)]))
model.add(hugectr.SparseEmbedding(embedding_type = hugectr.Embedding_t.DistributedSlotSparseEmbeddingHash,
workspace_size_per_gpu_in_mb = 80,
embedding_vec_size = 1,
combiner = "sum",
sparse_embedding_name = "sparse_embedding2",
bottom_name = "wide_data",
optimizer = optimizer))
model.add(hugectr.SparseEmbedding(embedding_type = hugectr.Embedding_t.DistributedSlotSparseEmbeddingHash,
workspace_size_per_gpu_in_mb = 1350,
embedding_vec_size = 16,
combiner = "sum",
sparse_embedding_name = "sparse_embedding1",
bottom_name = "deep_data",
optimizer = optimizer))
model.add(hugectr.DenseLayer(layer_type = hugectr.Layer_t.Reshape,
bottom_names = ["sparse_embedding1"],
top_names = ["reshape1"],
leading_dim=416))
model.add(hugectr.DenseLayer(layer_type = hugectr.Layer_t.Reshape,
bottom_names = ["sparse_embedding2"],
top_names = ["reshape2"],
leading_dim=2))
model.add(hugectr.DenseLayer(layer_type = hugectr.Layer_t.ReduceSum,
bottom_names = ["reshape2"],
top_names = ["wide_redn"],
axis = 1))
model.add(hugectr.DenseLayer(layer_type = hugectr.Layer_t.Concat,
bottom_names = ["reshape1", "dense"],
top_names = ["concat1"]))
model.add(hugectr.DenseLayer(layer_type = hugectr.Layer_t.InnerProduct,
bottom_names = ["concat1"],
top_names = ["fc1"],
num_output=1024))
model.add(hugectr.DenseLayer(layer_type = hugectr.Layer_t.ReLU,
bottom_names = ["fc1"],
top_names = ["relu1"]))
model.add(hugectr.DenseLayer(layer_type = hugectr.Layer_t.Dropout,
bottom_names = ["relu1"],
top_names = ["dropout1"],
dropout_rate=0.5))
model.add(hugectr.DenseLayer(layer_type = hugectr.Layer_t.InnerProduct,
bottom_names = ["dropout1"],
top_names = ["fc2"],
num_output=1024))
model.add(hugectr.DenseLayer(layer_type = hugectr.Layer_t.ReLU,
bottom_names = ["fc2"],
top_names = ["relu2"]))
model.add(hugectr.DenseLayer(layer_type = hugectr.Layer_t.Dropout,
bottom_names = ["relu2"],
top_names = ["dropout2"],
dropout_rate=0.5))
model.add(hugectr.DenseLayer(layer_type = hugectr.Layer_t.InnerProduct,
bottom_names = ["dropout2"],
top_names = ["fc3"],
num_output=1))
model.add(hugectr.DenseLayer(layer_type = hugectr.Layer_t.Add,
bottom_names = ["fc3", "wide_redn"],
top_names = ["add1"]))
model.add(hugectr.DenseLayer(layer_type = hugectr.Layer_t.BinaryCrossEntropyLoss,
bottom_names = ["add1", "label"],
top_names = ["loss"]))
model.compile()
model.summary()
model.fit(max_iter = 21000, display = 1000, eval_interval = 4000, snapshot = 20000, snapshot_prefix = "/wdl_train/model/wdl/")
model.graph_to_json(graph_config_file = "/wdl_train/model/wdl.json")
Overwriting ./model.py
!python ./model.py
MpiInitService: Initialized!
HugeCTR Version: 23.4
====================================================Model Init=====================================================
[HCTR][05:22:57.077][WARNING][RK0][main]: The model name is not specified when creating the solver.
[HCTR][05:22:57.077][INFO][RK0][main]: Global seed is 1262996030
[HCTR][05:22:57.759][INFO][RK0][main]: Device to NUMA mapping:
GPU 1 -> node 0
[HCTR][05:23:01.750][WARNING][RK0][main]: Peer-to-peer access cannot be fully enabled.
[HCTR][05:23:01.750][DEBUG][RK0][main]: [device 1] allocating 0.0000 GB, available 30.6238
[HCTR][05:23:01.750][INFO][RK0][main]: Start all2all warmup
[HCTR][05:23:01.751][INFO][RK0][main]: End all2all warmup
[HCTR][05:23:01.780][INFO][RK0][main]: Using All-reduce algorithm: NCCL
[HCTR][05:23:01.786][INFO][RK0][main]: Device 1: Tesla V100-SXM2-32GB
[HCTR][05:23:01.789][INFO][RK0][main]: eval source /wdl_train/val/_file_list.txt max_row_group_size 33421
[HCTR][05:23:01.792][INFO][RK0][main]: train source /wdl_train/train/_file_list.txt max_row_group_size 133025
[HCTR][05:23:01.793][INFO][RK0][main]: num of DataReader workers for train: 1
[HCTR][05:23:01.793][INFO][RK0][main]: num of DataReader workers for eval: 1
[HCTR][05:23:01.794][DEBUG][RK0][main]: [device 1] allocating 0.0018 GB, available 30.3679
[HCTR][05:23:01.795][DEBUG][RK0][main]: [device 1] allocating 0.0018 GB, available 30.3621
[HCTR][05:23:01.808][INFO][RK0][main]: Vocabulary size: 2138588
[HCTR][05:23:01.808][INFO][RK0][main]: max_vocabulary_size_per_gpu_=6990506
[HCTR][05:23:01.812][DEBUG][RK0][main]: [device 1] allocating 0.0788 GB, available 29.8347
[HCTR][05:23:01.812][INFO][RK0][main]: max_vocabulary_size_per_gpu_=7372800
[HCTR][05:23:01.816][DEBUG][RK0][main]: [device 1] allocating 1.3516 GB, available 28.3562
[HCTR][05:23:01.817][INFO][RK0][main]: Graph analysis to resolve tensor dependency
===================================================Model Compile===================================================
[HCTR][05:23:01.821][DEBUG][RK0][main]: [device 1] allocating 0.2162 GB, available 28.1238
[HCTR][05:23:01.821][DEBUG][RK0][main]: [device 1] allocating 0.0056 GB, available 28.1179
[HCTR][05:23:10.289][INFO][RK0][main]: gpu0 start to init embedding
[HCTR][05:23:10.289][INFO][RK0][main]: gpu0 init embedding done
[HCTR][05:23:10.289][INFO][RK0][main]: gpu0 start to init embedding
[HCTR][05:23:10.292][INFO][RK0][main]: gpu0 init embedding done
[HCTR][05:23:10.292][DEBUG][RK0][main]: [device 1] allocating 0.0001 GB, available 28.1179
[HCTR][05:23:10.294][INFO][RK0][main]: Starting AUC NCCL warm-up
[HCTR][05:23:10.299][INFO][RK0][main]: Warm-up done
[HCTR][05:23:10.299][DEBUG][RK0][main]: Nothing to preallocate
===================================================Model Summary===================================================
[HCTR][05:23:10.299][INFO][RK0][main]: Model structure on each GPU
Label Dense Sparse
label dense wide_data,deep_data
(2720,1) (2720,13)
——————————————————————————————————————————————————————————————————————————————————————————————————————————————————
Layer Type Input Name Output Name Output Shape
——————————————————————————————————————————————————————————————————————————————————————————————————————————————————
DistributedSlotSparseEmbeddingHash wide_data sparse_embedding2 (2720,2,1)
------------------------------------------------------------------------------------------------------------------
DistributedSlotSparseEmbeddingHash deep_data sparse_embedding1 (2720,26,16)
------------------------------------------------------------------------------------------------------------------
Reshape sparse_embedding1 reshape1 (2720,416)
------------------------------------------------------------------------------------------------------------------
Reshape sparse_embedding2 reshape2 (2720,2)
------------------------------------------------------------------------------------------------------------------
ReduceSum reshape2 wide_redn (2720,1)
------------------------------------------------------------------------------------------------------------------
Concat reshape1 concat1 (2720,429)
dense
------------------------------------------------------------------------------------------------------------------
InnerProduct concat1 fc1 (2720,1024)
------------------------------------------------------------------------------------------------------------------
ReLU fc1 relu1 (2720,1024)
------------------------------------------------------------------------------------------------------------------
Dropout relu1 dropout1 (2720,1024)
------------------------------------------------------------------------------------------------------------------
InnerProduct dropout1 fc2 (2720,1024)
------------------------------------------------------------------------------------------------------------------
ReLU fc2 relu2 (2720,1024)
------------------------------------------------------------------------------------------------------------------
Dropout relu2 dropout2 (2720,1024)
------------------------------------------------------------------------------------------------------------------
InnerProduct dropout2 fc3 (2720,1)
------------------------------------------------------------------------------------------------------------------
Add fc3 add1 (2720,1)
wide_redn
------------------------------------------------------------------------------------------------------------------
BinaryCrossEntropyLoss add1 loss
label
------------------------------------------------------------------------------------------------------------------
=====================================================Model Fit=====================================================
[HCTR][05:23:10.299][INFO][RK0][main]: Use non-epoch mode with number of iterations: 21000
[HCTR][05:23:10.299][INFO][RK0][main]: Training batchsize: 2720, evaluation batchsize: 2720
[HCTR][05:23:10.299][INFO][RK0][main]: Evaluation interval: 4000, snapshot interval: 20000
[HCTR][05:23:10.299][INFO][RK0][main]: Dense network trainable: True
[HCTR][05:23:10.299][INFO][RK0][main]: Sparse embedding sparse_embedding1 trainable: True
[HCTR][05:23:10.299][INFO][RK0][main]: Sparse embedding sparse_embedding2 trainable: True
[HCTR][05:23:10.299][INFO][RK0][main]: Use mixed precision: False, scaler: 1.000000, use cuda graph: True
[HCTR][05:23:10.299][INFO][RK0][main]: lr: 0.001000, warmup_steps: 1, end_lr: 0.000000
[HCTR][05:23:10.299][INFO][RK0][main]: decay_start: 0, decay_steps: 1, decay_power: 2.000000
[HCTR][05:23:10.299][INFO][RK0][main]: Training source file: /wdl_train/train/_file_list.txt
[HCTR][05:23:10.299][INFO][RK0][main]: Evaluation source file: /wdl_train/val/_file_list.txt
[HCTR][05:23:18.987][INFO][RK0][main]: Iter: 1000 Time(1000 iters): 8.68321s Loss: 0.125823 lr:0.001
[HCTR][05:23:27.587][INFO][RK0][main]: Iter: 2000 Time(1000 iters): 8.5956s Loss: 0.11697 lr:0.001
[HCTR][05:23:36.175][INFO][RK0][main]: Iter: 3000 Time(1000 iters): 8.58384s Loss: 0.115881 lr:0.001
[HCTR][05:23:44.749][INFO][RK0][main]: Iter: 4000 Time(1000 iters): 8.56965s Loss: 0.114301 lr:0.001
[HCTR][05:23:49.496][INFO][RK0][main]: Evaluation, AUC: 0.747877
[HCTR][05:23:49.496][INFO][RK0][main]: Eval Time for 4000 iters: 4.74613s
[HCTR][05:23:58.100][INFO][RK0][main]: Iter: 5000 Time(1000 iters): 13.3459s Loss: 0.126996 lr:0.001
[HCTR][05:24:06.702][INFO][RK0][main]: Iter: 6000 Time(1000 iters): 8.59771s Loss: 0.108037 lr:0.001
[HCTR][05:24:15.301][INFO][RK0][main]: Iter: 7000 Time(1000 iters): 8.59455s Loss: 0.126929 lr:0.001
[HCTR][05:24:23.899][INFO][RK0][main]: Iter: 8000 Time(1000 iters): 8.59391s Loss: 0.105164 lr:0.001
[HCTR][05:24:28.506][INFO][RK0][main]: Evaluation, AUC: 0.719409
[HCTR][05:24:28.506][INFO][RK0][main]: Eval Time for 4000 iters: 4.60613s
[HCTR][05:24:37.102][INFO][RK0][main]: Iter: 9000 Time(1000 iters): 13.1988s Loss: 0.108601 lr:0.001
[HCTR][05:24:45.695][INFO][RK0][main]: Iter: 10000 Time(1000 iters): 8.58771s Loss: 0.114181 lr:0.001
[HCTR][05:24:54.294][INFO][RK0][main]: Iter: 11000 Time(1000 iters): 8.59515s Loss: 0.102487 lr:0.001
[HCTR][05:25:02.895][INFO][RK0][main]: Iter: 12000 Time(1000 iters): 8.59662s Loss: 0.101383 lr:0.001
[HCTR][05:25:07.517][INFO][RK0][main]: Evaluation, AUC: 0.696508
[HCTR][05:25:07.517][INFO][RK0][main]: Eval Time for 4000 iters: 4.62149s
[HCTR][05:25:16.101][INFO][RK0][main]: Iter: 13000 Time(1000 iters): 13.2012s Loss: 0.106047 lr:0.001
[HCTR][05:25:24.690][INFO][RK0][main]: Iter: 14000 Time(1000 iters): 8.58473s Loss: 0.114361 lr:0.001
[HCTR][05:25:33.283][INFO][RK0][main]: Iter: 15000 Time(1000 iters): 8.58798s Loss: 0.0902672 lr:0.001
[HCTR][05:25:41.863][INFO][RK0][main]: Iter: 16000 Time(1000 iters): 8.57613s Loss: 0.0979891 lr:0.001
[HCTR][05:25:46.496][INFO][RK0][main]: Evaluation, AUC: 0.686972
[HCTR][05:25:46.496][INFO][RK0][main]: Eval Time for 4000 iters: 4.63229s
[HCTR][05:25:55.091][INFO][RK0][main]: Iter: 17000 Time(1000 iters): 13.2233s Loss: 0.115308 lr:0.001
[HCTR][05:26:03.673][INFO][RK0][main]: Iter: 18000 Time(1000 iters): 8.57796s Loss: 0.0990158 lr:0.001
[HCTR][05:26:12.256][INFO][RK0][main]: Iter: 19000 Time(1000 iters): 8.578s Loss: 0.0970965 lr:0.001
[HCTR][05:26:20.853][INFO][RK0][main]: Iter: 20000 Time(1000 iters): 8.59287s Loss: 0.0835783 lr:0.001
[HCTR][05:26:25.485][INFO][RK0][main]: Evaluation, AUC: 0.662475
[HCTR][05:26:25.485][INFO][RK0][main]: Eval Time for 4000 iters: 4.63122s
[HCTR][05:26:25.497][INFO][RK0][main]: Rank0: Write hash table to file
[HCTR][05:26:25.519][INFO][RK0][main]: Rank0: Write hash table to file
[HCTR][05:26:25.537][INFO][RK0][main]: Dumping sparse weights to files, successful
[HCTR][05:26:25.550][INFO][RK0][main]: Rank0: Write optimzer state to file
[HCTR][05:26:25.563][INFO][RK0][main]: Done
[HCTR][05:26:25.578][INFO][RK0][main]: Rank0: Write optimzer state to file
[HCTR][05:26:25.591][INFO][RK0][main]: Done
[HCTR][05:26:25.856][INFO][RK0][main]: Rank0: Write optimzer state to file
[HCTR][05:26:26.071][INFO][RK0][main]: Done
[HCTR][05:26:26.348][INFO][RK0][main]: Rank0: Write optimzer state to file
[HCTR][05:26:26.561][INFO][RK0][main]: Done
[HCTR][05:26:26.576][INFO][RK0][main]: Dumping sparse optimzer states to files, successful
[HCTR][05:26:26.579][INFO][RK0][main]: Dumping dense weights to file, successful
[HCTR][05:26:26.586][INFO][RK0][main]: Dumping dense optimizer states to file, successful
[HCTR][05:26:35.196][INFO][RK0][main]: Iter: 21000 Time(1000 iters): 14.3386s Loss: 0.0844829 lr:0.001
[HCTR][05:26:35.196][INFO][RK0][main]: Finish 21000 iterations with batchsize: 2720 in 204.90s.
[HCTR][05:26:35.197][INFO][RK0][main]: Save the model graph to /wdl_train/model/wdl.json successfully
Prepare Inference Request
!ls -l /wdl_train/val
total 634936
-rw-r--r-- 1 root root 242 May 26 04:31 _file_list.txt
-rw-r--r-- 1 root root 217718 May 26 04:31 _metadata
-rw-r--r-- 1 root root 1879 May 26 04:31 _metadata.json
-rw-r--r-- 1 root root 17489097 May 26 04:31 part_0.parquet
-rw-r--r-- 1 root root 17521515 May 26 04:31 part_1.parquet
-rw-r--r-- 1 root root 17459606 May 26 04:31 part_2.parquet
-rw-r--r-- 1 root root 17556341 May 26 04:31 part_3.parquet
-rw-r--r-- 1 root root 17527364 May 26 04:31 part_4.parquet
-rw-r--r-- 1 root root 17492305 May 26 04:31 part_5.parquet
-rw-r--r-- 1 root root 17508965 May 26 04:31 part_6.parquet
-rw-r--r-- 1 root root 17575602 May 26 04:31 part_7.parquet
-rw-r--r-- 1 root root 31277 May 26 04:31 schema.pbtxt
drwxr-xr-x 2 root root 50 May 26 04:30 temp-parquet-after-conversion
-rw-r--r-- 1 root root 509766965 May 26 04:30 test.txt
import pandas as pd
df = pd.read_parquet("/wdl_train/val/part_0.parquet")
df.head()
I1 | I2 | I3 | I4 | I5 | I6 | I7 | I8 | I9 | I10 | ... | C17 | C18 | C19 | C20 | C21 | C22 | C23 | C24 | C25 | C26 | |
---|---|---|---|---|---|---|---|---|---|---|---|---|---|---|---|---|---|---|---|---|---|
0 | -0.051831 | -0.490904 | -0.512615 | -0.135830 | -0.222800 | -0.164725 | -0.053983 | -0.298238 | -0.435927 | -0.409435 | ... | 2 | 2 | 4 | 0 | 24804 | 0 | 14164 | 2028 | 1 | 5 |
1 | -0.067326 | -0.015481 | -0.512615 | -0.135830 | -0.222800 | -0.164725 | -0.053983 | -0.298238 | 2.166419 | -0.409435 | ... | 0 | 27 | 7 | 2 | 2 | 2 | 0 | 813 | 2 | 1 |
2 | -0.065389 | -0.486852 | -0.626555 | -0.100009 | -0.172440 | -0.164725 | -0.053983 | -0.295652 | -0.761220 | -0.409435 | ... | 2 | 3 | 1 | 0 | 890 | 0 | 1483 | 167 | 5 | 9 |
3 | 0.198029 | -0.494956 | -0.398675 | -0.045562 | -0.206014 | -0.164725 | -0.053983 | -0.202561 | -0.679897 | -0.409435 | ... | 3 | 1 | 1 | 672 | 466 | 722 | 794 | 2261 | 5 | 1 |
4 | -0.007282 | -0.484151 | 0.740724 | -0.125800 | -0.222800 | 0.398216 | -0.053983 | -0.298238 | 0.214659 | 1.698777 | ... | 1 | 1 | 1 | 338 | 482 | 364 | 102 | 5759 | 1 | 1 |
5 rows × 42 columns
df.head(10).to_csv('/wdl_train/infer_test.csv', sep=',', index=False,header=True)
Create prediction scripts
%%writefile '/wdl_train/wdl_predict.py'
from hugectr.inference import InferenceParams, CreateInferenceSession
import hugectr
import pandas as pd
import numpy as np
import sys
from mpi4py import MPI
def wdl_inference(model_name, network_file, dense_file, embedding_file_list, data_file, enable_cache, use_rocksdb=False, rocksdb_path=None):
CATEGORICAL_COLUMNS=["C" + str(x) for x in range(1, 27)]+["C1_C2","C3_C4"]
CONTINUOUS_COLUMNS=["I" + str(x) for x in range(1, 14)]
LABEL_COLUMNS = ['label']
emb_size = [249058, 19561, 14212, 6890, 18592, 4, 6356, 1254, 52, 226170, 80508, 72308, 11, 2169, 7597, 61, 4, 923, 15, 249619, 168974, 243480, 68212, 9169, 75, 34, 278018, 415262]
shift = np.insert(np.cumsum(emb_size), 0, 0)[:-1]
test_df=pd.read_csv(data_file,sep=',')
config_file = network_file
row_ptrs = list(range(0,21))+list(range(0,261))
dense_features = list(test_df[CONTINUOUS_COLUMNS].values.flatten())
test_df[CATEGORICAL_COLUMNS].astype(np.int64)
embedding_columns = list((test_df[CATEGORICAL_COLUMNS]+shift).values.flatten())
persistent_db_params = hugectr.inference.PersistentDatabaseParams()
if use_rocksdb:
persistent_db_params = hugectr.inference.PersistentDatabaseParams(
backend = hugectr.DatabaseType_t.rocks_db,
path = rocksdb_path
)
# create parameter server, embedding cache and inference session
inference_params = InferenceParams(model_name = model_name,
max_batchsize = 64,
hit_rate_threshold = 0.5,
dense_model_file = dense_file,
sparse_model_files = embedding_file_list,
device_id = 0,
use_gpu_embedding_cache = enable_cache,
cache_size_percentage = 0.9,
persistent_db = persistent_db_params,
i64_input_key = True,
use_mixed_precision = False)
inference_session = CreateInferenceSession(config_file, inference_params)
output = inference_session.predict(dense_features, embedding_columns, row_ptrs)
print("WDL multi-embedding table inference result is {}".format(output))
if __name__ == "__main__":
model_name = sys.argv[1]
print("{} multi-embedding table prediction".format(model_name))
network_file = sys.argv[2]
print("{} multi-embedding table prediction network is {}".format(model_name,network_file))
dense_file = sys.argv[3]
print("{} multi-embedding table prediction dense file is {}".format(model_name,dense_file))
embedding_file_list = str(sys.argv[4]).split(',')
print("{} multi-embedding table prediction sparse files are {}".format(model_name,embedding_file_list))
data_file = sys.argv[5]
print("{} multi-embedding table prediction input data path is {}".format(model_name,data_file))
input_dbtype = sys.argv[6]
print("{} multi-embedding table prediction input dbtype path is {}".format(model_name,input_dbtype))
if input_dbtype=="disabled":
wdl_inference(model_name, network_file, dense_file, embedding_file_list, data_file, True)
if input_dbtype=="rocksdb":
rocksdb_path = sys.argv[7]
print("{} multi-embedding table prediction rocksdb_path path is {}".format(model_name,rocksdb_path))
wdl_inference(model_name, network_file, dense_file, embedding_file_list, data_file, True, True, rocksdb_path)
Overwriting /wdl_train/wdl_predict.py
Prediction
Use different types of databases as a local parameter server to get the wide and deep model prediction results.
Load model embedding tables into local memory as parameter server
!python /wdl_train/wdl_predict.py "wdl" "/wdl_train/model/wdl.json" "/wdl_train/model/wdl/_dense_20000.model" "/wdl_train/model/wdl/0_sparse_20000.model/,/wdl_train/model/wdl/1_sparse_20000.model" "/wdl_train/infer_test.csv" "disabled"
wdl multi-embedding table prediction
wdl multi-embedding table prediction network is /wdl_train/model/wdl.json
wdl multi-embedding table prediction dense file is /wdl_train/model/wdl/_dense_20000.model
wdl multi-embedding table prediction sparse files are ['/wdl_train/model/wdl/0_sparse_20000.model/', '/wdl_train/model/wdl/1_sparse_20000.model']
wdl multi-embedding table prediction input data path is /wdl_train/infer_test.csv
wdl multi-embedding table prediction input dbtype path is disabled
MpiInitService: MPI was already initialized by another (non-HugeCTR) mechanism.
[HCTR][05:28:49.476][WARNING][RK0][main]: default_value_for_each_table.size() is not equal to the number of embedding tables
[HCTR][05:28:49.476][INFO][RK0][main]: default_emb_vec_value is not specified using default: 0
[HCTR][05:28:49.476][INFO][RK0][main]: default_emb_vec_value is not specified using default: 0
====================================================HPS Create====================================================
[HCTR][05:28:49.476][INFO][RK0][main]: Creating HashMap CPU database backend...
[HCTR][05:28:49.476][DEBUG][RK0][main]: Created blank database backend in local memory!
[HCTR][05:28:49.476][INFO][RK0][main]: Volatile DB: initial cache rate = 1
[HCTR][05:28:49.476][INFO][RK0][main]: Volatile DB: cache missed embeddings = 0
[HCTR][05:28:49.476][DEBUG][RK0][main]: Created raw model loader in local memory!
[HCTR][05:28:49.675][INFO][RK0][main]: Table: hps_et.wdl.sparse_embedding2; cached 190851 / 190851 embeddings in volatile database (HashMapBackend); load: 190851 / 18446744073709551615 (0.00%).
[HCTR][05:28:49.881][INFO][RK0][main]: Table: hps_et.wdl.sparse_embedding1; cached 438628 / 438628 embeddings in volatile database (HashMapBackend); load: 438628 / 18446744073709551615 (0.00%).
[HCTR][05:28:49.881][DEBUG][RK0][main]: Real-time subscribers created!
[HCTR][05:28:49.881][INFO][RK0][main]: Creating embedding cache in device 0.
[HCTR][05:28:49.886][INFO][RK0][main]: Model name: wdl
[HCTR][05:28:49.886][INFO][RK0][main]: Max batch size: 64
[HCTR][05:28:49.886][INFO][RK0][main]: Fuse embedding tables: False
[HCTR][05:28:49.886][INFO][RK0][main]: Number of embedding tables: 2
[HCTR][05:28:49.886][INFO][RK0][main]: Use GPU embedding cache: True, cache size percentage: 0.900000
[HCTR][05:28:49.886][INFO][RK0][main]: Embedding cache type: dynamic
[HCTR][05:28:49.886][INFO][RK0][main]: Use I64 input key: True
[HCTR][05:28:49.886][INFO][RK0][main]: Configured cache hit rate threshold: 0.500000
[HCTR][05:28:49.886][INFO][RK0][main]: The size of thread pool: 80
[HCTR][05:28:49.886][INFO][RK0][main]: The size of worker memory pool: 2
[HCTR][05:28:49.886][INFO][RK0][main]: The size of refresh memory pool: 1
[HCTR][05:28:49.886][INFO][RK0][main]: The refresh percentage : 0.000000
[HCTR][05:28:51.447][INFO][RK0][main]: Global seed is 3595974557
[HCTR][05:28:51.531][INFO][RK0][main]: Device to NUMA mapping:
GPU 0 -> node 0
[HCTR][05:28:53.402][WARNING][RK0][main]: Peer-to-peer access cannot be fully enabled.
[HCTR][05:28:53.402][DEBUG][RK0][main]: [device 0] allocating 0.0000 GB, available 30.5847
[HCTR][05:28:53.402][INFO][RK0][main]: Start all2all warmup
[HCTR][05:28:53.402][INFO][RK0][main]: End all2all warmup
[HCTR][05:28:53.403][INFO][RK0][main]: Model name: wdl
[HCTR][05:28:53.403][INFO][RK0][main]: Use mixed precision: False
[HCTR][05:28:53.403][INFO][RK0][main]: Use cuda graph: True
[HCTR][05:28:53.403][INFO][RK0][main]: Max batchsize: 64
[HCTR][05:28:53.403][INFO][RK0][main]: Use I64 input key: True
[HCTR][05:28:53.403][INFO][RK0][main]: start create embedding for inference
[HCTR][05:28:53.403][INFO][RK0][main]: sparse_input name wide_data
[HCTR][05:28:53.403][INFO][RK0][main]: sparse_input name deep_data
[HCTR][05:28:53.403][INFO][RK0][main]: create embedding for inference success
[HCTR][05:28:53.403][DEBUG][RK0][main]: [device 0] allocating 0.0001 GB, available 30.3347
[HCTR][05:28:53.404][INFO][RK0][main]: Inference stage skip BinaryCrossEntropyLoss layer, replaced by Sigmoid layer
[HCTR][05:28:53.404][DEBUG][RK0][main]: [device 0] allocating 0.0128 GB, available 30.3132
[HCTR][05:28:53.985][WARNING][RK0][main]: InferenceSession is not suitable for multi-GPU offline inference. Please use InferenceModel: https://nvidia-merlin.github.io/HugeCTR/main/api/python_interface.html#inferencemodel
WDL multi-embedding table inference result is [0.09066542983055115, 0.26852551102638245, 0.28295737504959106, 0.07364904880523682, 0.2965098023414612, 0.10407719761133194, 0.4754742681980133, 0.5024058818817139, 0.05602413788437843, 0.07264009118080139]
Load model embedding tables into local RocksDB as a parameter Server
Create a RocksDB directory with read and write permissions for storing model embedded tables.
!mkdir -p -m 700 /wdl_train/rocksdb
!python /wdl_train/wdl_predict.py "wdl" "./wdl.json" \
"/wdl_train/model/wdl/_dense_20000.model" \
"/wdl_train/model/wdl/0_sparse_20000.model/,/wdl_train/model/wdl/1_sparse_20000.model" \
"/wdl_train/infer_test.csv" \
"rocksdb" "/wdl_train/rocksdb"
wdl multi-embedding table prediction
wdl multi-embedding table prediction network is ./wdl.json
wdl multi-embedding table prediction dense file is /wdl_train/model/wdl/_dense_20000.model
wdl multi-embedding table prediction sparse files are ['/wdl_train/model/wdl/0_sparse_20000.model/', '/wdl_train/model/wdl/1_sparse_20000.model']
wdl multi-embedding table prediction input data path is /wdl_train/infer_test.csv
wdl multi-embedding table prediction input dbtype path is rocksdb
wdl multi-embedding table prediction rocksdb_path path is /wdl_train/rocksdb
MpiInitService: MPI was already initialized by another (non-HugeCTR) mechanism.
[HCTR][05:29:24.931][WARNING][RK0][main]: default_value_for_each_table.size() is not equal to the number of embedding tables
[HCTR][05:29:24.932][INFO][RK0][main]: default_emb_vec_value is not specified using default: 0
[HCTR][05:29:24.932][INFO][RK0][main]: default_emb_vec_value is not specified using default: 0
====================================================HPS Create====================================================
[HCTR][05:29:24.932][INFO][RK0][main]: Creating HashMap CPU database backend...
[HCTR][05:29:24.932][DEBUG][RK0][main]: Created blank database backend in local memory!
[HCTR][05:29:24.932][INFO][RK0][main]: Volatile DB: initial cache rate = 1
[HCTR][05:29:24.932][INFO][RK0][main]: Volatile DB: cache missed embeddings = 0
[HCTR][05:29:24.932][INFO][RK0][main]: Creating RocksDB backend...
[HCTR][05:29:24.932][INFO][RK0][main]: Connecting to RocksDB database...
[HCTR][05:29:24.934][INFO][RK0][main]: RocksDB /wdl_train/rocksdb, found column family `default`.
[HCTR][05:29:24.962][INFO][RK0][main]: Connected to RocksDB database!
[HCTR][05:29:24.962][DEBUG][RK0][main]: Created raw model loader in local memory!
[HCTR][05:29:25.190][INFO][RK0][main]: Table: hps_et.wdl.sparse_embedding2; cached 190851 / 190851 embeddings in volatile database (HashMapBackend); load: 190851 / 18446744073709551615 (0.00%).
[HCTR][05:29:25.734][INFO][RK0][main]: Table: hps_et.wdl.sparse_embedding1; cached 438628 / 438628 embeddings in volatile database (HashMapBackend); load: 438628 / 18446744073709551615 (0.00%).
[HCTR][05:29:26.579][DEBUG][RK0][main]: Real-time subscribers created!
[HCTR][05:29:26.579][INFO][RK0][main]: Creating embedding cache in device 0.
[HCTR][05:29:26.584][INFO][RK0][main]: Model name: wdl
[HCTR][05:29:26.584][INFO][RK0][main]: Max batch size: 64
[HCTR][05:29:26.584][INFO][RK0][main]: Fuse embedding tables: False
[HCTR][05:29:26.584][INFO][RK0][main]: Number of embedding tables: 2
[HCTR][05:29:26.584][INFO][RK0][main]: Use GPU embedding cache: True, cache size percentage: 0.900000
[HCTR][05:29:26.584][INFO][RK0][main]: Embedding cache type: dynamic
[HCTR][05:29:26.584][INFO][RK0][main]: Use I64 input key: True
[HCTR][05:29:26.584][INFO][RK0][main]: Configured cache hit rate threshold: 0.500000
[HCTR][05:29:26.584][INFO][RK0][main]: The size of thread pool: 80
[HCTR][05:29:26.584][INFO][RK0][main]: The size of worker memory pool: 2
[HCTR][05:29:26.584][INFO][RK0][main]: The size of refresh memory pool: 1
[HCTR][05:29:26.584][INFO][RK0][main]: The refresh percentage : 0.000000
[HCTR][05:29:28.096][INFO][RK0][main]: Global seed is 1275207064
[HCTR][05:29:28.175][INFO][RK0][main]: Device to NUMA mapping:
GPU 0 -> node 0
[HCTR][05:29:30.024][WARNING][RK0][main]: Peer-to-peer access cannot be fully enabled.
[HCTR][05:29:30.024][DEBUG][RK0][main]: [device 0] allocating 0.0000 GB, available 30.5847
[HCTR][05:29:30.024][INFO][RK0][main]: Start all2all warmup
[HCTR][05:29:30.024][INFO][RK0][main]: End all2all warmup
[HCTR][05:29:30.025][INFO][RK0][main]: Model name: wdl
[HCTR][05:29:30.025][INFO][RK0][main]: Use mixed precision: False
[HCTR][05:29:30.025][INFO][RK0][main]: Use cuda graph: True
[HCTR][05:29:30.025][INFO][RK0][main]: Max batchsize: 64
[HCTR][05:29:30.025][INFO][RK0][main]: Use I64 input key: True
[HCTR][05:29:30.025][INFO][RK0][main]: start create embedding for inference
[HCTR][05:29:30.025][INFO][RK0][main]: sparse_input name wide_data
[HCTR][05:29:30.025][INFO][RK0][main]: sparse_input name deep_data
[HCTR][05:29:30.025][INFO][RK0][main]: create embedding for inference success
[HCTR][05:29:30.025][DEBUG][RK0][main]: [device 0] allocating 0.0001 GB, available 30.3347
[HCTR][05:29:30.026][INFO][RK0][main]: Inference stage skip BinaryCrossEntropyLoss layer, replaced by Sigmoid layer
[HCTR][05:29:30.026][DEBUG][RK0][main]: [device 0] allocating 0.0128 GB, available 30.3132
[HCTR][05:29:30.573][WARNING][RK0][main]: InferenceSession is not suitable for multi-GPU offline inference. Please use InferenceModel: https://nvidia-merlin.github.io/HugeCTR/main/api/python_interface.html#inferencemodel
WDL multi-embedding table inference result is [0.09066542983055115, 0.26852551102638245, 0.28295737504959106, 0.07364904880523682, 0.2965098023414612, 0.10407719761133194, 0.4754742681980133, 0.5024058818817139, 0.05602413788437843, 0.07264009118080139]
[HCTR][05:29:30.576][INFO][RK0][main]: Disconnecting from RocksDB database...
[HCTR][05:29:30.578][INFO][RK0][main]: Disconnected from RocksDB database!