Benchmark the HPS Plugin for TensorFlow
Benchmark Setup
The inference SavedModel that leverages HPS can be deployed with the Triton TensorFlow Backend, which is demonstrated in hps_tensorflow_triton_deployment_demo.ipynb. The inference performance of this deployment method needs to be investigated using Triton Performance Analyzer to verify the effectiveness of the HPS integration into TensorFlow.
We train a DLRM model following JoC TensorFlow2 DLRM on the Criteo 1TB dataset filtered with the frequency threshold of 15. The trained model is in the SavedModel format and the size is about 16GB, which is almost the size of embedding weights because the size of dense layer weights is small. We compare three deployment methods on the Triton Infrence Server:
Deploy the trained SavedModel directly with the TensorFlow backend.
Deploy the inference SavedModel that leverages HPS with the TensorFlow backend. The workflow of deriving the inference SavedModel is illustrated in HPS TensorFlow WorkFlow.
Deploy the ensemble model that combines the Triton HPS backend and the Triton TensorFlow backend. The demo for this deployment method can be found at the HPS Triton Ensemble notebook.
The benchmark is conducted on DGX A100, and only one GPU is utilized with one Triton model instance on it. The best-case performance for HPS is studied by sending the same batch of inference data repeatedly. In this case, the embedding lookup is served by the GPU embedding cache of HPS. As for the method of deploying the trained SavedModel, the whole embedding weights (~16GB) can fit into the 80GB GPU memory of A100, which can be regarded as the baseline of the benchmark.
Results and Analysis
The per-batch latency, in milliseconds, measured at the server side is shown in the following table and Fig. 1. The Y-axis is logarithmic.
Batch size |
Native TF Model |
HPS Plugin TF Model |
Ensemble Model |
---|---|---|---|
32 |
0.636 |
0.612 |
0.854 |
64 |
0.754 |
0.651 |
1.008 |
256 |
0.756 |
0.669 |
1.802 |
1024 |
0.922 |
0.870 |
4.420 |
2048 |
1.176 |
1.100 |
8.087 |
4096 |
1.447 |
1.552 |
23.383 |
8192 |
2.443 |
2.524 |
42.303 |
16384 |
3.901 |
4.402 |
82.310 |
32768 |
7.681 |
8.320 |
291.335 |
65536 |
13.665 |
15.577 |
617.968 |
131072 |
29.636 |
29.952 |
1190.025 |
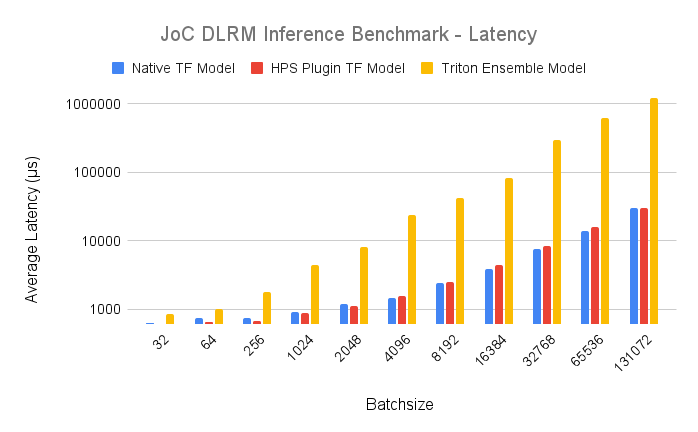
The throughput, in K samples per second, measured at the server side is shown in the following table and Fig. 2. The Y-axis is logarithmic.
Batch size |
Native TF Model |
HPS Plugin TF Model |
Ensemble Model |
---|---|---|---|
32 |
50.314 |
52.287 |
37.470 |
64 |
84.880 |
98.310 |
63.492 |
256 |
338.624 |
382.660 |
142.064 |
1024 |
1110.629 |
1177.011 |
231.674 |
2048 |
1741.496 |
1861.818 |
253.245 |
4096 |
2830.684 |
2639.175 |
175.169 |
8192 |
3353.254 |
3245.641 |
193.650 |
16384 |
4199.948 |
3721.944 |
199.052 |
32768 |
4266.111 |
3938.461 |
112.475 |
65536 |
4795.901 |
4207.228 |
106.050 |
131072 |
4422.729 |
4376.068 |
110.142 |
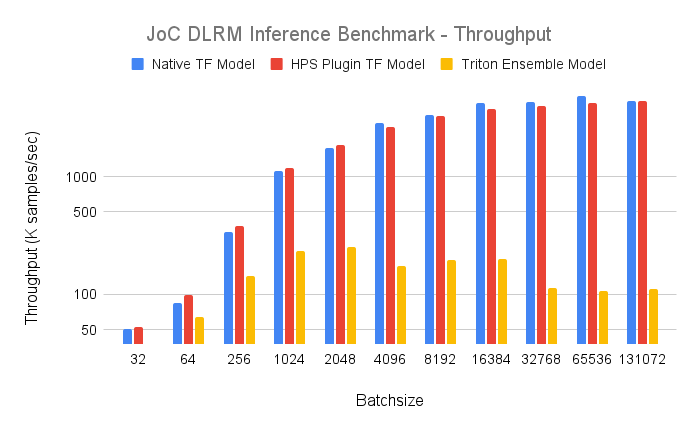
As the benchmark results indicate, the performance of inference SavedModel is comparable to that of trained SavedModel. The results show that the best-case performance of HPS embedding lookup, when serviced by the GPU embedding cache, is on par with that of native TensorFlow GPU embedding lookup. Often, large embedding weights cannot fit in GPU memory. The native TensorFlow GPU embedding lookup does not support this condition. However, the HPS plugin for TensorFlow can handle embedding tables that exceed GPU memory with a hierarchical memory storage and provide a low-latency embedding lookup service with an efficient GPU caching mechanism.
Among the three deployment methods, the performance of the Triton ensemble model is much worse than the other two, which can be attributed to the overhead of data transfer between two backends. Specifically, the embedding vectors output by the HPS backend will be fed to the TensorFlow backend, and the size of embedding vectors increase linearly with the batch size (attaining 131072*128*4 bytes for the batch size 131072). Therefore, the latency is orders of magnitude higher than the other two methods.