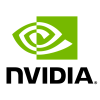
Hierarchical Parameter Server Demo
Overview
In HugeCTR version 3.5, we provide Python APIs for embedding table lookup with HugeCTR Hierarchical Parameter Server (HPS) HPS supports different database backends and GPU embedding caches.
This notebook demonstrates how to use HPS with HugeCTR Python APIs. Without loss of generality, the HPS APIs are utilized together with the ONNX Runtime APIs to create an ensemble inference model, where HPS is responsible for embedding table lookup while the ONNX model takes charge of feed forward of dense neural networks.
Setup
To setup the environment, refer to HugeCTR Example Notebooks and follow the instructions there before running the following.
Data Generation
HugeCTR provides a tool to generate synthetic datasets. The Data Generator is capable of generating datasets of different file formats and different distributions. We will generate one-hot Parquet datasets with power-law distribution for this notebook:
import hugectr
from hugectr.tools import DataGeneratorParams, DataGenerator
data_generator_params = DataGeneratorParams(
format = hugectr.DataReaderType_t.Parquet,
label_dim = 1,
dense_dim = 10,
num_slot = 4,
i64_input_key = True,
nnz_array = [1, 1, 1, 1],
source = "./data_parquet/file_list.txt",
eval_source = "./data_parquet/file_list_test.txt",
slot_size_array = [10000, 10000, 10000, 10000],
check_type = hugectr.Check_t.Non,
dist_type = hugectr.Distribution_t.PowerLaw,
power_law_type = hugectr.PowerLaw_t.Short,
num_files = 16,
eval_num_files = 4,
num_samples_per_file = 40960)
data_generator = DataGenerator(data_generator_params)
data_generator.generate()
[HCTR][11:15:15][INFO][RK0][main]: Generate Parquet dataset
[HCTR][11:15:15][INFO][RK0][main]: train data folder: ./data_parquet, eval data folder: ./data_parquet, slot_size_array: 10000, 10000, 10000, 10000, nnz array: 1, 1, 1, 1, #files for train: 16, #files for eval: 4, #samples per file: 40960, Use power law distribution: 1, alpha of power law: 1.3
[HCTR][11:15:15][INFO][RK0][main]: ./data_parquet exist
[HCTR][11:15:15][INFO][RK0][main]: ./data_parquet exist
[HCTR][11:15:15][INFO][RK0][main]: ./data_parquet/train exist
[HCTR][11:15:15][INFO][RK0][main]: ./data_parquet/train/gen_0.parquet
[HCTR][11:15:17][INFO][RK0][main]: ./data_parquet/train/gen_1.parquet
[HCTR][11:15:17][INFO][RK0][main]: ./data_parquet/train/gen_2.parquet
[HCTR][11:15:17][INFO][RK0][main]: ./data_parquet/train/gen_3.parquet
[HCTR][11:15:17][INFO][RK0][main]: ./data_parquet/train/gen_4.parquet
[HCTR][11:15:18][INFO][RK0][main]: ./data_parquet/train/gen_5.parquet
[HCTR][11:15:18][INFO][RK0][main]: ./data_parquet/train/gen_6.parquet
[HCTR][11:15:18][INFO][RK0][main]: ./data_parquet/train/gen_7.parquet
[HCTR][11:15:18][INFO][RK0][main]: ./data_parquet/train/gen_8.parquet
[HCTR][11:15:18][INFO][RK0][main]: ./data_parquet/train/gen_9.parquet
[HCTR][11:15:19][INFO][RK0][main]: ./data_parquet/train/gen_10.parquet
[HCTR][11:15:19][INFO][RK0][main]: ./data_parquet/train/gen_11.parquet
[HCTR][11:15:19][INFO][RK0][main]: ./data_parquet/train/gen_12.parquet
[HCTR][11:15:19][INFO][RK0][main]: ./data_parquet/train/gen_13.parquet
[HCTR][11:15:19][INFO][RK0][main]: ./data_parquet/train/gen_14.parquet
[HCTR][11:15:20][INFO][RK0][main]: ./data_parquet/train/gen_15.parquet
[HCTR][11:15:20][INFO][RK0][main]: ./data_parquet/file_list.txt done!
[HCTR][11:15:20][INFO][RK0][main]: ./data_parquet/val exist
[HCTR][11:15:20][INFO][RK0][main]: ./data_parquet/val/gen_0.parquet
[HCTR][11:15:20][INFO][RK0][main]: ./data_parquet/val/gen_1.parquet
[HCTR][11:15:20][INFO][RK0][main]: ./data_parquet/val/gen_2.parquet
[HCTR][11:15:20][INFO][RK0][main]: ./data_parquet/val/gen_3.parquet
[HCTR][11:15:21][INFO][RK0][main]: ./data_parquet/file_list_test.txt done!
Train from Scratch
We can train fom scratch by performing the following steps with Python APIs:
Create the solver, reader and optimizer, then initialize the model.
Construct the model graph by adding input, sparse embedding and dense layers in order.
Compile the model and have an overview of the model graph.
Dump the model graph to the JSON file.
Fit the model, save the model weights and optimizer states implicitly.
Dump one batch of evaluation results to files.
%%writefile train.py
import hugectr
from mpi4py import MPI
solver = hugectr.CreateSolver(model_name = "hps_demo",
max_eval_batches = 1,
batchsize_eval = 1024,
batchsize = 1024,
lr = 0.001,
vvgpu = [[0]],
i64_input_key = True,
repeat_dataset = True,
use_cuda_graph = True)
reader = hugectr.DataReaderParams(data_reader_type = hugectr.DataReaderType_t.Parquet,
source = ["./data_parquet/file_list.txt"],
eval_source = "./data_parquet/file_list_test.txt",
check_type = hugectr.Check_t.Non,
slot_size_array = [10000, 10000, 10000, 10000])
optimizer = hugectr.CreateOptimizer(optimizer_type = hugectr.Optimizer_t.Adam)
model = hugectr.Model(solver, reader, optimizer)
model.add(hugectr.Input(label_dim = 1, label_name = "label",
dense_dim = 10, dense_name = "dense",
data_reader_sparse_param_array =
[hugectr.DataReaderSparseParam("data1", [1, 1], True, 2),
hugectr.DataReaderSparseParam("data2", [1, 1], True, 2)]))
model.add(hugectr.SparseEmbedding(embedding_type = hugectr.Embedding_t.DistributedSlotSparseEmbeddingHash,
workspace_size_per_gpu_in_mb = 4,
embedding_vec_size = 16,
combiner = "sum",
sparse_embedding_name = "sparse_embedding1",
bottom_name = "data1",
optimizer = optimizer))
model.add(hugectr.SparseEmbedding(embedding_type = hugectr.Embedding_t.DistributedSlotSparseEmbeddingHash,
workspace_size_per_gpu_in_mb = 8,
embedding_vec_size = 32,
combiner = "sum",
sparse_embedding_name = "sparse_embedding2",
bottom_name = "data2",
optimizer = optimizer))
model.add(hugectr.DenseLayer(layer_type = hugectr.Layer_t.Reshape,
bottom_names = ["sparse_embedding1"],
top_names = ["reshape1"],
leading_dim=32))
model.add(hugectr.DenseLayer(layer_type = hugectr.Layer_t.Reshape,
bottom_names = ["sparse_embedding2"],
top_names = ["reshape2"],
leading_dim=64))
model.add(hugectr.DenseLayer(layer_type = hugectr.Layer_t.Concat,
bottom_names = ["reshape1", "reshape2", "dense"], top_names = ["concat1"]))
model.add(hugectr.DenseLayer(layer_type = hugectr.Layer_t.InnerProduct,
bottom_names = ["concat1"],
top_names = ["fc1"],
num_output=1024))
model.add(hugectr.DenseLayer(layer_type = hugectr.Layer_t.ReLU,
bottom_names = ["fc1"],
top_names = ["relu1"]))
model.add(hugectr.DenseLayer(layer_type = hugectr.Layer_t.InnerProduct,
bottom_names = ["relu1"],
top_names = ["fc2"],
num_output=1))
model.add(hugectr.DenseLayer(layer_type = hugectr.Layer_t.BinaryCrossEntropyLoss,
bottom_names = ["fc2", "label"],
top_names = ["loss"]))
model.compile()
model.summary()
model.graph_to_json("hps_demo.json")
model.fit(max_iter = 1100, display = 200, eval_interval = 1000, snapshot = 1000, snapshot_prefix = "hps_demo")
model.export_predictions("hps_demo_pred_" + str(1000), "hps_demo_label_" + str(1000))
Overwriting train.py
!python3 train.py
HugeCTR Version: 3.4
====================================================Model Init=====================================================
[HCTR][11:15:26][INFO][RK0][main]: Initialize model: hps_demo
[HCTR][11:15:26][INFO][RK0][main]: Global seed is 156170895
[HCTR][11:15:26][INFO][RK0][main]: Device to NUMA mapping:
GPU 0 -> node 0
[HCTR][11:15:27][WARNING][RK0][main]: Peer-to-peer access cannot be fully enabled.
[HCTR][11:15:27][INFO][RK0][main]: Start all2all warmup
[HCTR][11:15:27][INFO][RK0][main]: End all2all warmup
[HCTR][11:15:27][INFO][RK0][main]: Using All-reduce algorithm: NCCL
[HCTR][11:15:27][INFO][RK0][main]: Device 0: Tesla V100-SXM2-32GB
[HCTR][11:15:27][INFO][RK0][main]: num of DataReader workers: 1
[HCTR][11:15:27][INFO][RK0][main]: Vocabulary size: 40000
[HCTR][11:15:27][INFO][RK0][main]: max_vocabulary_size_per_gpu_=21845
[HCTR][11:15:27][INFO][RK0][main]: max_vocabulary_size_per_gpu_=21845
[HCTR][11:15:27][INFO][RK0][main]: Graph analysis to resolve tensor dependency
===================================================Model Compile===================================================
[HCTR][11:15:29][INFO][RK0][main]: gpu0 start to init embedding
[HCTR][11:15:29][INFO][RK0][main]: gpu0 init embedding done
[HCTR][11:15:29][INFO][RK0][main]: gpu0 start to init embedding
[HCTR][11:15:29][INFO][RK0][main]: gpu0 init embedding done
[HCTR][11:15:29][INFO][RK0][main]: Starting AUC NCCL warm-up
[HCTR][11:15:29][INFO][RK0][main]: Warm-up done
===================================================Model Summary===================================================
[HCTR][11:15:29][INFO][RK0][main]: label Dense Sparse
label dense data1,data2
(None, 1) (None, 10)
——————————————————————————————————————————————————————————————————————————————————————————————————————————————————
Layer Type Input Name Output Name Output Shape
——————————————————————————————————————————————————————————————————————————————————————————————————————————————————
DistributedSlotSparseEmbeddingHash data1 sparse_embedding1 (None, 2, 16)
------------------------------------------------------------------------------------------------------------------
DistributedSlotSparseEmbeddingHash data2 sparse_embedding2 (None, 2, 32)
------------------------------------------------------------------------------------------------------------------
Reshape sparse_embedding1 reshape1 (None, 32)
------------------------------------------------------------------------------------------------------------------
Reshape sparse_embedding2 reshape2 (None, 64)
------------------------------------------------------------------------------------------------------------------
Concat reshape1 concat1 (None, 106)
reshape2
dense
------------------------------------------------------------------------------------------------------------------
InnerProduct concat1 fc1 (None, 1024)
------------------------------------------------------------------------------------------------------------------
ReLU fc1 relu1 (None, 1024)
------------------------------------------------------------------------------------------------------------------
InnerProduct relu1 fc2 (None, 1)
------------------------------------------------------------------------------------------------------------------
BinaryCrossEntropyLoss fc2 loss
label
------------------------------------------------------------------------------------------------------------------
[HCTR][11:15:29][INFO][RK0][main]: Save the model graph to hps_demo.json successfully
=====================================================Model Fit=====================================================
[HCTR][11:15:29][INFO][RK0][main]: Use non-epoch mode with number of iterations: 1100
[HCTR][11:15:29][INFO][RK0][main]: Training batchsize: 1024, evaluation batchsize: 1024
[HCTR][11:15:29][INFO][RK0][main]: Evaluation interval: 1000, snapshot interval: 1000
[HCTR][11:15:29][INFO][RK0][main]: Dense network trainable: True
[HCTR][11:15:29][INFO][RK0][main]: Sparse embedding sparse_embedding1 trainable: True
[HCTR][11:15:29][INFO][RK0][main]: Sparse embedding sparse_embedding2 trainable: True
[HCTR][11:15:29][INFO][RK0][main]: Use mixed precision: False, scaler: 1.000000, use cuda graph: True
[HCTR][11:15:29][INFO][RK0][main]: lr: 0.001000, warmup_steps: 1, end_lr: 0.000000
[HCTR][11:15:29][INFO][RK0][main]: decay_start: 0, decay_steps: 1, decay_power: 2.000000
[HCTR][11:15:29][INFO][RK0][main]: Training source file: ./data_parquet/file_list.txt
[HCTR][11:15:29][INFO][RK0][main]: Evaluation source file: ./data_parquet/file_list_test.txt
[HCTR][11:15:29][INFO][RK0][main]: Iter: 200 Time(200 iters): 0.211451s Loss: 0.694128 lr:0.001
[HCTR][11:15:29][INFO][RK0][main]: Iter: 400 Time(200 iters): 0.267199s Loss: 0.689953 lr:0.001
[HCTR][11:15:29][INFO][RK0][main]: Iter: 600 Time(200 iters): 0.216242s Loss: 0.689657 lr:0.001
[HCTR][11:15:29][INFO][RK0][main]: Iter: 800 Time(200 iters): 0.215779s Loss: 0.677149 lr:0.001
[HCTR][11:15:30][INFO][RK0][main]: Iter: 1000 Time(200 iters): 0.219875s Loss: 0.681208 lr:0.001
[HCTR][11:15:30][INFO][RK0][main]: Evaluation, AUC: 0.49589
[HCTR][11:15:30][INFO][RK0][main]: Eval Time for 1 iters: 0.000359s
[HCTR][11:15:30][INFO][RK0][main]: Rank0: Write hash table to file
[HCTR][11:15:30][INFO][RK0][main]: Rank0: Write hash table to file
[HCTR][11:15:30][INFO][RK0][main]: Dumping sparse weights to files, successful
[HCTR][11:15:30][INFO][RK0][main]: Rank0: Write optimzer state to file
[HCTR][11:15:30][INFO][RK0][main]: Done
[HCTR][11:15:30][INFO][RK0][main]: Rank0: Write optimzer state to file
[HCTR][11:15:30][INFO][RK0][main]: Done
[HCTR][11:15:30][INFO][RK0][main]: Rank0: Write optimzer state to file
[HCTR][11:15:30][INFO][RK0][main]: Done
[HCTR][11:15:30][INFO][RK0][main]: Rank0: Write optimzer state to file
[HCTR][11:15:30][INFO][RK0][main]: Done
[HCTR][11:15:30][INFO][RK0][main]: Dumping sparse optimzer states to files, successful
[HCTR][11:15:30][INFO][RK0][main]: Dumping dense weights to file, successful
[HCTR][11:15:30][INFO][RK0][main]: Dumping dense optimizer states to file, successful
[HCTR][11:15:30][INFO][RK0][main]: Finish 1100 iterations with batchsize: 1024 in 1.53s.
Convert HugeCTR to ONNX
We will convert the saved HugeCTR models to ONNX using the HugeCTR to ONNX Converter. For more information about the converter, refer to the README in the onnx_converter directory of the repository.
For the sake of double checking the correctness, we will investigate both cases of conversion depending on whether or not to convert the sparse embedding models.
import hugectr2onnx
hugectr2onnx.converter.convert(onnx_model_path = "hps_demo_with_embedding.onnx",
graph_config = "hps_demo.json",
dense_model = "hps_demo_dense_1000.model",
convert_embedding = True,
sparse_models = ["hps_demo0_sparse_1000.model", "hps_demo1_sparse_1000.model"])
hugectr2onnx.converter.convert(onnx_model_path = "hps_demo_without_embedding.onnx",
graph_config = "hps_demo.json",
dense_model = "hps_demo_dense_1000.model",
convert_embedding = False)
[HUGECTR2ONNX][INFO]: Converting Data layer to ONNX
[HUGECTR2ONNX][INFO]: Converting DistributedSlotSparseEmbeddingHash layer to ONNX
[HUGECTR2ONNX][INFO]: Converting DistributedSlotSparseEmbeddingHash layer to ONNX
[HUGECTR2ONNX][INFO]: Converting Reshape layer to ONNX
[HUGECTR2ONNX][INFO]: Converting Reshape layer to ONNX
[HUGECTR2ONNX][INFO]: Converting Concat layer to ONNX
[HUGECTR2ONNX][INFO]: Converting InnerProduct layer to ONNX
[HUGECTR2ONNX][INFO]: Converting ReLU layer to ONNX
[HUGECTR2ONNX][INFO]: Converting InnerProduct layer to ONNX
[HUGECTR2ONNX][INFO]: Converting Sigmoid layer to ONNX
[HUGECTR2ONNX][INFO]: The model is checked!
[HUGECTR2ONNX][INFO]: The model is saved at hps_demo_with_embedding.onnx
[HUGECTR2ONNX][INFO]: Converting Data layer to ONNX
Skip sparse embedding layers in converted ONNX model
[HUGECTR2ONNX][INFO]: Converting DistributedSlotSparseEmbeddingHash layer to ONNX
Skip sparse embedding layers in converted ONNX model
[HUGECTR2ONNX][INFO]: Converting DistributedSlotSparseEmbeddingHash layer to ONNX
[HUGECTR2ONNX][INFO]: Converting Reshape layer to ONNX
[HUGECTR2ONNX][INFO]: Converting Reshape layer to ONNX
[HUGECTR2ONNX][INFO]: Converting Concat layer to ONNX
[HUGECTR2ONNX][INFO]: Converting InnerProduct layer to ONNX
[HUGECTR2ONNX][INFO]: Converting ReLU layer to ONNX
[HUGECTR2ONNX][INFO]: Converting InnerProduct layer to ONNX
[HUGECTR2ONNX][INFO]: Converting Sigmoid layer to ONNX
[HUGECTR2ONNX][INFO]: The model is checked!
[HUGECTR2ONNX][INFO]: The model is saved at hps_demo_without_embedding.onnx
Inference with HPS & ONNX
We will make inference by performing the following steps with Python APIs:
Configure the HPS hyperparameters.
Initialize the HPS object, which is responsible for embedding table lookup.
Loading the Parquet data.
Make inference with the HPS object and the ONNX inference session of
hps_demo_without_embedding.onnx
.Check the correctness by comparing with dumped evaluation results.
Make inference with the ONNX inference session of
hps_demo_with_embedding.onnx
(double check).
from hugectr.inference import HPS, ParameterServerConfig, InferenceParams
import pandas as pd
import numpy as np
import onnxruntime as ort
slot_size_array = [10000, 10000, 10000, 10000]
key_offset = np.insert(np.cumsum(slot_size_array), 0, 0)[:-1]
batch_size = 2048
# 1. Configure the HPS hyperparameters
ps_config = ParameterServerConfig(
emb_table_name = {"hps_demo": ["sparse_embedding1", "sparse_embedding2"]},
embedding_vec_size = {"hps_demo": [16, 32]},
max_feature_num_per_sample_per_emb_table = {"hps_demo": [2, 2]},
inference_params_array = [
InferenceParams(
model_name = "hps_demo",
max_batchsize = batch_size,
hit_rate_threshold = 1.0,
dense_model_file = "",
sparse_model_files = ["hps_demo0_sparse_1000.model", "hps_demo1_sparse_1000.model"],
deployed_devices = [0],
use_gpu_embedding_cache = True,
cache_size_percentage = 0.5,
i64_input_key = True)
])
# 2. Initialize the HPS object
hps = HPS(ps_config)
# 3. Loading the Parquet data.
df = pd.read_parquet("data_parquet/val/gen_0.parquet")
dense_input_columns = df.columns[1:11]
cat_input1_columns = df.columns[11:13]
cat_input2_columns = df.columns[13:15]
dense_input = df[dense_input_columns].loc[0:batch_size-1].to_numpy(dtype=np.float32)
cat_input1 = (df[cat_input1_columns].loc[0:batch_size-1].to_numpy(dtype=np.int64) + key_offset[0:2]).reshape((batch_size, 2, 1))
cat_input2 = (df[cat_input2_columns].loc[0:batch_size-1].to_numpy(dtype=np.int64) + key_offset[2:4]).reshape((batch_size, 2, 1))
# 4. Make inference from the HPS object and the ONNX inference session of `hps_demo_without_embedding.onnx`.
embedding1 = hps.lookup(cat_input1.flatten(), "hps_demo", 0).reshape(batch_size, 2, 16)
embedding2 = hps.lookup(cat_input2.flatten(), "hps_demo", 1).reshape(batch_size, 2, 32)
sess = ort.InferenceSession("hps_demo_without_embedding.onnx")
res = sess.run(output_names=[sess.get_outputs()[0].name],
input_feed={sess.get_inputs()[0].name: dense_input,
sess.get_inputs()[1].name: embedding1,
sess.get_inputs()[2].name: embedding2})
pred = res[0]
# 5. Check the correctness by comparing with dumped evaluation results.
ground_truth = np.loadtxt("hps_demo_pred_1000")
print("ground_truth: ", ground_truth)
diff = pred.flatten()-ground_truth
mse = np.mean(diff*diff)
print("pred: ", pred)
print("mse between pred and ground_truth: ", mse)
# 6. Make inference with the ONNX inference session of `hps_demo_with_embedding.onnx` (double check).
sess_ref = ort.InferenceSession("hps_demo_with_embedding.onnx")
res_ref = sess_ref.run(output_names=[sess_ref.get_outputs()[0].name],
input_feed={sess_ref.get_inputs()[0].name: dense_input,
sess_ref.get_inputs()[1].name: cat_input1,
sess_ref.get_inputs()[2].name: cat_input2})
pred_ref = res_ref[0]
diff_ref = pred_ref.flatten()-ground_truth
mse_ref = np.mean(diff_ref*diff_ref)
print("pred_ref: ", pred_ref)
print("mse between pred_ref and ground_truth: ", mse_ref)
[HCTR][11:58:45.621][WARNING][RK0][main]: default_value_for_each_table.size() is not equal to the number of embedding tables
====================================================HPS Create====================================================
[HCTR][11:58:45.621][INFO][RK0][main]: Creating HashMap CPU database backend...
[HCTR][11:58:45.621][DEBUG][RK0][main]: Created blank database backend in local memory!
[HCTR][11:58:45.621][INFO][RK0][main]: Volatile DB: initial cache rate = 1
[HCTR][11:58:45.621][INFO][RK0][main]: Volatile DB: cache missed embeddings = 0
[HCTR][11:58:45.621][DEBUG][RK0][main]: Created raw model loader in local memory!
[HCTR][11:58:45.621][INFO][RK0][main]: Using Local file system backend.
[HCTR][11:58:45.843][INFO][RK0][main]: Table: hps_et.hps_demo.sparse_embedding1; cached 18401 / 18401 embeddings in volatile database (HashMapBackend); load: 18401 / 18446744073709551615 (0.00%).
[HCTR][11:58:45.843][INFO][RK0][main]: Using Local file system backend.
[HCTR][11:58:46.045][INFO][RK0][main]: Table: hps_et.hps_demo.sparse_embedding2; cached 18436 / 18436 embeddings in volatile database (HashMapBackend); load: 18436 / 18446744073709551615 (0.00%).
[HCTR][11:58:46.045][DEBUG][RK0][main]: Real-time subscribers created!
[HCTR][11:58:46.045][INFO][RK0][main]: Creating embedding cache in device 0.
[HCTR][11:58:46.052][INFO][RK0][main]: Model name: hps_demo
[HCTR][11:58:46.052][INFO][RK0][main]: Max batch size: 1024
[HCTR][11:58:46.052][INFO][RK0][main]: Number of embedding tables: 2
[HCTR][11:58:46.052][INFO][RK0][main]: Use GPU embedding cache: True, cache size percentage: 0.500000
[HCTR][11:58:46.052][INFO][RK0][main]: Use static table: False
[HCTR][11:58:46.052][INFO][RK0][main]: Use I64 input key: True
[HCTR][11:58:46.052][INFO][RK0][main]: Configured cache hit rate threshold: 1.000000
[HCTR][11:58:46.052][INFO][RK0][main]: The size of thread pool: 80
[HCTR][11:58:46.052][INFO][RK0][main]: The size of worker memory pool: 2
[HCTR][11:58:46.052][INFO][RK0][main]: The size of refresh memory pool: 1
[HCTR][11:58:46.052][INFO][RK0][main]: The refresh percentage : 0.000000
[HCTR][11:58:46.053][INFO][RK0][main]: Creating lookup session for hps_demo on device: 0
ground_truth: [0.52604 0.528162 0.510473 ... 0.511216 0.464687 0.420649]
pred: [[0.5260405 ]
[0.52816164]
[0.5104735 ]
...
[0.5112164 ]
[0.46468708]
[0.42064884]]
mse between pred and ground_truth: 8.653384858862709e-14
pred_ref: [[0.5260405 ]
[0.52816164]
[0.5104735 ]
...
[0.5112164 ]
[0.46468708]
[0.42064884]]
mse between pred_ref and ground_truth: 8.653384858862709e-14
2022-12-13 11:58:46.521333358 [W:onnxruntime:, graph.cc:3559 CleanUnusedInitializersAndNodeArgs] Removing initializer 'key_to_indice_hash_all_tables'. It is not used by any node and should be removed from the model.
Lookup the Embedding Vector from DLPack
We also provide a lookup_fromdlpack
interface that could query embedding keys on the CPU
and return the embedding vectors on the GPU/CPU
.
Suppose you have created a Pytorch/Tensorflow tensor that stores the embedded keys.
Convert the embedding key tensor to DLPack capsule through the corresponding platform’s
to_dlpack
function.Creates an empty tensor as a buffer to store embedding vectors.
Convert a buffer tensor to DLPack capsule.
Lookup the embedding vector of the corresponding embedding key directly through
lookup_fromdlpack
interface, and output it to the embedding vector buffer tensorIf the output capsule is allocated on the GPU, then a
device_id
needs to be specified inlookup_fromdlpack
interface for corresponding embedding cache. If not specified, the default value is device 0
Note: Please make sure that tensorflow or pytorch have been installed correctly in the container
embedding1 = hps.lookup(cat_input1.flatten(), "hps_demo", 0).reshape(batch_size, 2, 16)
embedding2 = hps.lookup(cat_input2.flatten(), "hps_demo", 1).reshape(batch_size, 2, 32)
# 1. Look up from dlpack for Pytorch tensor on CPU
print(" Look up from dlpack for Pytorch tensor")
import torch.utils.dlpack
import os
print("************Look up from pytorch dlpack on CPU")
device = torch.device("cpu")
key = torch.tensor(cat_input1.flatten(),dtype=torch.int64, device=device)
out = torch.empty((1,cat_input1.flatten().shape[0]*16), dtype=torch.float32, device=device)
key_capsule = torch.utils.dlpack.to_dlpack(key)
print("The device type of embedding keys that lookup dlpack from hps interface for embedding table 0 of hps_demo: {}, the keys: {}".format(key.device, key))
out_capsule = torch.utils.dlpack.to_dlpack(out)
# Lookup the embedding vectors from dlpack
hps.lookup_fromdlpack(key_capsule, out_capsule,"hps_demo", 0)
out_put = torch.utils.dlpack.from_dlpack(out_capsule)
print("[The device type of embedding vectors that lookup dlpack from hps interface for embedding table 0 of hps_demo: {}, the vectors: {}\n".format(out_put.device, out_put))
diff = out_put-embedding1.reshape(1,cat_input1.flatten().shape[0]*16)
if diff.mean() > 1e-4:
raise RuntimeError("Too large mse between pytorch dlpack on cpu and native HPS lookup api: {}".format(diff.mean()))
sys.exit(1)
else:
print("Pytorch dlpack on cpu results are consistent with native HPS lookup api, mse: {}".format(diff.mean()))
# 2. Look up from dlpack for Pytorch tensor on GPU
print("************Look up from pytorch dlpack on GPU")
cuda_device = torch.device("cuda:0" if torch.cuda.is_available else "cpu")
key = torch.tensor(cat_input1.flatten(),dtype=torch.int64, device=device)
key_capsule = torch.utils.dlpack.to_dlpack(key)
out = torch.empty((cat_input1.flatten().shape[0]*16), dtype=torch.float32, device=cuda_device)
out_capsule = torch.utils.dlpack.to_dlpack(out)
hps.lookup_fromdlpack(key_capsule, out_capsule,"hps_demo", 0)
out_put = torch.utils.dlpack.from_dlpack(out_capsule)
print("The device type of embedding vectors that lookup dlpack from hps interface for embedding table 0 of hps_demo: {}, the vectors: {}\n\n".format(out_put.device, out_put))
diff = out_put.cpu()-embedding1.reshape(1,cat_input1.flatten().shape[0]*16)
if diff.mean() > 1e-3:
raise RuntimeError("Too large mse between pytorch dlpack on cpu and native HPS lookup api: {}".format(diff.mean()))
sys.exit(1)
else:
print("Pytorch dlpack on GPU results are consistent with native HPS lookup api, mse: {}".format(diff.mean()))
Look up from dlpack for Pytorch tensor
************Look up from pytorch dlpack on CPU
The device type of embedding keys that lookup dlpack from hps interface for embedding table 0 of hps_demo: cpu, the keys: tensor([ 4, 10000, 17, ..., 10208, 5, 10012])
[The device type of embedding vectors that lookup dlpack from hps interface for embedding table 0 of hps_demo: cpu, the vectors: tensor([[ 0.0201, 0.0179, 0.0029, ..., 0.0168, -0.0059, 0.0017]])
Pytorch dlpack on cpu results are consistent with native HPS lookup api, mse: 0.0
************Look up from pytorch dlpack on GPU
The device type of embedding vectors that lookup dlpack from hps interface for embedding table 0 of hps_demo: cuda:0, the vectors: tensor([ 0.0201, 0.0179, 0.0029, ..., 0.0168, -0.0059, 0.0017],
device='cuda:0')
Pytorch dlpack on GPU results are consistent with native HPS lookup api, mse: 0.0
# 3. Look up from dlpack for tensorflow tensor on CPU
print("Look up from dlpack for Tensorflow tensor")
from tensorflow.python.dlpack import dlpack
import tensorflow as tf
from tensorflow.python.eager import context
from tensorflow.python.framework import dtypes
print("***************Look up from tensorflow dlpack on CPU**********")
with tf.device('/CPU:0'):
key_tensor = tf.constant(cat_input2.flatten(),dtype=tf.int64)
out_tensor = tf.zeros([1, cat_input2.flatten().shape[0]*32],dtype=tf.float32)
print("The device type of embedding keys that lookup dlpack from hps interface for embedding table 1 of hps_demo: {}, the keys: {}".format(key_tensor.device, key_tensor))
key_capsule = tf.experimental.dlpack.to_dlpack(key_tensor)
out_dlcapsule = tf.experimental.dlpack.to_dlpack(out_tensor)
hps.lookup_fromdlpack(key_capsule,out_dlcapsule, "hps_demo", 1)
out= tf.experimental.dlpack.from_dlpack(out_dlcapsule)
print("The device type of embedding vectors that lookup dlpack from hps interface for embedding table 1 of hps_demo: {}, the vectors: {}\n".format(out.device, out))
diff = out-embedding2.reshape(1,cat_input2.flatten().shape[0]*32)
mse = tf.reduce_mean(diff)
if mse> 1e-3:
raise RuntimeError("Too large mse between tensorflow dlpack on cpu and native HPS lookup api: {}".format(mse))
sys.exit(1)
else:
print("tensorflow dlpack on CPU results are consistent with native HPS lookup api, mse: {}".format(mse))
# 4. Look up from dlpack for tensorflow tensor on GPU
print("***************Look up from tensorflow dlpack on GPU**********")
with tf.device('/GPU:0'):
key_tensor = tf.constant(cat_input2.flatten(),dtype=tf.int64)
out_tensor = tf.zeros([cat_input2.flatten().shape[0]*32],dtype=tf.float32)
key_capsule = tf.experimental.dlpack.to_dlpack(key_tensor)
out_dlcapsule = tf.experimental.dlpack.to_dlpack(out_tensor)
hps.lookup_fromdlpack(key_capsule,out_dlcapsule, "hps_demo", 1)
out= tf.experimental.dlpack.from_dlpack(out_dlcapsule)
print("[HUGECTR][INFO] The device type of embedding vectors that lookup dlpack from hps interface for embedding table 1 of wdl: {}, the vectors: {}\n".format(out.device, out))
diff = out-embedding2.reshape(1,cat_input2.flatten().shape[0]*32)
mse = tf.reduce_mean(diff)
if mse> 1e-3:
raise RuntimeError("Too large mse between tensorflow dlpack on cpu and native HPS lookup api: {}".format(mse))
sys.exit(1)
else:
print("tensorflow dlpack on GPU results are consistent with native HPS lookup api, mse: {}".format(mse))
Look up from dlpack for Tensorflow tensor
***************Look up from tensorflow dlpack on CPU**********
The device type of embedding keys that lookup dlpack from hps interface for embedding table 1 of hps_demo: /job:localhost/replica:0/task:0/device:CPU:0, the keys: [20005 30347 20001 ... 30174 20000 30013]
The device type of embedding vectors that lookup dlpack from hps interface for embedding table 1 of hps_demo: /job:localhost/replica:0/task:0/device:CPU:0, the vectors: [[ 0.02120136 0.03807243 -0.04021286 ... -0.00556568 0.00462132
0.01774719]]
tensorflow dlpack on CPU results are consistent with native HPS lookup api, mse: 0.0
***************Look up from tensorflow dlpack on GPU**********
[HUGECTR][INFO] The device type of embedding vectors that lookup dlpack from hps interface for embedding table 1 of wdl: /job:localhost/replica:0/task:0/device:GPU:0, the vectors: [ 0.02120136 0.03807243 -0.04021286 ... -0.00556568 0.00462132
0.01774719]
tensorflow dlpack on GPU results are consistent with native HPS lookup api, mse: 0.0
Multi-process inference
It is possible to share the a hashmap database between multiple processes. The followng example launches 3 processes which achieve this using the operating system’s shared memory, which is located at /dev/shm
in most unix systems. In this example, we separate processes into a primary and multiple secondary processes, and only the primary process initializes the shared memory database. The secondary processes wait until the shared memory has been fully initialized. However, note that inter-process database access is guaranteed to be thread-safe. Therefore, it is also possible to implement more complicated initialization/refresh mechanisms for your use-case.
%%writefile multi_process_hps.py
import os
import time
import multiprocessing as mp
import pandas as pd
import numpy as np
import onnxruntime as ort
from hugectr import DatabaseType_t
from hugectr.inference import HPS, ParameterServerConfig, InferenceParams, VolatileDatabaseParams
slot_size_array = [10000, 10000, 10000, 10000]
key_offset = np.insert(np.cumsum(slot_size_array), 0, 0)[:-1]
batch_size = 1024
def create_hps(name, initialized,device_id=0):
print(f'subprocess:{name}({os.getpid()})launch...')
# 1. Let secondary processes wait until shared memory is initialized.
while name != 'primary' and initialized.value == 0:
print(f'Subprocess {name} awaiting initialization...')
time.sleep(1)
# 2. Configure the HPS hyperparameters
ps_config = ParameterServerConfig(
emb_table_name = {"hps_demo": ["sparse_embedding1", "sparse_embedding2"]},
embedding_vec_size = {"hps_demo": [16, 32]},
max_feature_num_per_sample_per_emb_table = {"hps_demo": [2, 2]},
inference_params_array = [
InferenceParams(
model_name = "hps_demo",
max_batchsize = batch_size,
hit_rate_threshold = 1.0,
dense_model_file = "",
sparse_model_files = ["hps_demo0_sparse_1000.model", "hps_demo1_sparse_1000.model"],
device_id=device_id,
deployed_devices = [device_id],
use_gpu_embedding_cache = True,
cache_size_percentage = 0.5,
i64_input_key = True)
],
volatile_db = VolatileDatabaseParams(
DatabaseType_t.multi_process_hash_map, # Use /dev/shm instead of normal memory for storage.
# Skips initializing modl. If we run HPS in multiple processes, only one needs to initialize.
initialize_after_startup = name == 'primary',
))
# 3. Initialize the HPS object
hps = HPS(ps_config)
initialized.value += 1
print(f'Subprocess {name} initialized')
# 4. Load query data.
df = pd.read_parquet("data_parquet/val/gen_0.parquet")
dense_input_columns = df.columns[1:11]
cat_input1_columns = df.columns[11:13]
cat_input2_columns = df.columns[13:15]
dense_input = df[dense_input_columns].loc[0:batch_size-1].to_numpy(dtype=np.float32)
cat_input1 = (df[cat_input1_columns].loc[0:batch_size-1].to_numpy(dtype=np.int64) + key_offset[0:2]).reshape((batch_size, 2, 1))
cat_input2 = (df[cat_input2_columns].loc[0:batch_size-1].to_numpy(dtype=np.int64) + key_offset[2:4]).reshape((batch_size, 2, 1))
# 5. Make inference from the HPS object and the ONNX inference session of `hps_demo_without_embedding.onnx`.
embedding1 = hps.lookup(cat_input1.flatten(), "hps_demo", 0,device_id).reshape(batch_size, 2, 16)
embedding2 = hps.lookup(cat_input2.flatten(), "hps_demo", 1,device_id).reshape(batch_size, 2, 32)
sess = ort.InferenceSession("hps_demo_without_embedding.onnx")
res = sess.run(output_names=[sess.get_outputs()[0].name],
input_feed={sess.get_inputs()[0].name: dense_input,
sess.get_inputs()[1].name: embedding1,
sess.get_inputs()[2].name: embedding2})
pred = res[0]
# 6. Check the correctness by comparing with dumped evaluation results.
ground_truth = np.loadtxt("hps_demo_pred_1000")
print(f'Subprocess {name}; ground_truth: {ground_truth}')
diff = pred.flatten()-ground_truth
mse = np.mean(diff*diff)
print(f'Subprocess {name}; pred: {pred}')
print(f'Subprocess {name}; mse between pred and ground_truth: {mse}')
# 7. Make inference with the ONNX inference session of `hps_demo_with_embedding.onnx` (double check).
sess_ref = ort.InferenceSession("hps_demo_with_embedding.onnx")
res_ref = sess_ref.run(output_names=[sess_ref.get_outputs()[0].name],
input_feed={sess_ref.get_inputs()[0].name: dense_input,
sess_ref.get_inputs()[1].name: cat_input1,
sess_ref.get_inputs()[2].name: cat_input2})
pred_ref = res_ref[0]
diff_ref = pred_ref.flatten()-ground_truth
mse_ref = np.mean(diff_ref*diff_ref)
print(f'Subprocess {name}; pred_ref: {pred_ref}')
print(f'Subprocess {name}; mse between pred_ref and ground_truth: {mse_ref}')
print(f'Subprocess {name} exiting...')
#Make sure the primary process is not detached prematurely
time.sleep(10)
if __name__ == '__main__':
# Destroy shared memory.
try:
os.remove('/dev/shm/hctr_mp_hash_map_database')
except:
pass
initialized = mp.Value('i', 0)
# Create sub processes.
processes = [
mp.Process(target=create_hps, args=('primary', initialized,0)),
mp.Process(target=create_hps, args=('secondary', initialized,1)),
mp.Process(target=create_hps, args=('secondary', initialized,2)),
]
for p in processes:
p.start()
# Go to sleep until subprocesses are initialized.
while initialized.value < len(processes):
print(f'Main process; awaiting subprocess initializatiopn... So far {initialized.value} initialized...')
time.sleep(1)
# Wait for subprocesses to exit.
for i, p in enumerate(processes):
print(f'Main process; awaiting subprocess {i} to exit...')
p.join()
print(f'Main process; exiting...')
Overwriting multi_process_hps.py
!python3 multi_process_hps.py
subprocess:primary(23604)launch...
[HCTR][12:00:52.960][WARNING][RK0][main]: default_value_for_each_table.size() is not equal to the number of embedding tables
====================================================HPS Create====================================================
[HCTR][12:00:52.960][INFO][RK0][main]: Creating Multi-Process HashMap CPU database backend...
[HCTR][12:00:52.960][INFO][RK0][main]: Connecting to shared memory 'hctr_mp_hash_map_database'...
subprocess:secondary(23605)launch...
Subprocess secondary awaiting initialization...
Main process; awaiting subprocess initializatiopn... So far 0 initialized...
subprocess:secondary(23607)launch...
Subprocess secondary awaiting initialization...
[HCTR][12:00:52.960][INFO][RK0][main]: Connected to shared memory 'hctr_mp_hash_map_database'; OS total = 17179869184 bytes, OS available = 17179852800 bytes, HCTR allocated = 17179869184 bytes, HCTR free = 17179868640 bytes; other processes connected = 0
[HCTR][12:00:53.460][INFO][RK0][main]: Volatile DB: initial cache rate = 1
[HCTR][12:00:53.461][INFO][RK0][main]: Volatile DB: cache missed embeddings = 0
[HCTR][12:00:53.461][DEBUG][RK0][main]: Created raw model loader in local memory!
[HCTR][12:00:53.461][INFO][RK0][main]: Using Local file system backend.
Subprocess secondary awaiting initialization...
Main process; awaiting subprocess initializatiopn... So far 0 initialized...
Subprocess secondary awaiting initialization...
[HCTR][12:00:54.230][INFO][RK0][main]: Table: hps_et.hps_demo.sparse_embedding1; cached 18401 / 18401 embeddings in volatile database (MultiProcessHashMapBackend); load: 18401 / 18446744073709551615 (0.00%).
[HCTR][12:00:54.231][INFO][RK0][main]: Using Local file system backend.
[HCTR][12:00:54.748][INFO][RK0][main]: Table: hps_et.hps_demo.sparse_embedding2; cached 18436 / 18436 embeddings in volatile database (MultiProcessHashMapBackend); load: 18436 / 18446744073709551615 (0.00%).
[HCTR][12:00:54.748][DEBUG][RK0][main]: Real-time subscribers created!
[HCTR][12:00:54.748][INFO][RK0][main]: Creating embedding cache in device 0.
[HCTR][12:00:54.755][INFO][RK0][main]: Model name: hps_demo
[HCTR][12:00:54.755][INFO][RK0][main]: Max batch size: 1024
[HCTR][12:00:54.755][INFO][RK0][main]: Number of embedding tables: 2
[HCTR][12:00:54.755][INFO][RK0][main]: Use GPU embedding cache: True, cache size percentage: 0.500000
[HCTR][12:00:54.755][INFO][RK0][main]: Use static table: False
[HCTR][12:00:54.755][INFO][RK0][main]: Use I64 input key: True
[HCTR][12:00:54.755][INFO][RK0][main]: Configured cache hit rate threshold: 1.000000
[HCTR][12:00:54.755][INFO][RK0][main]: The size of thread pool: 80
[HCTR][12:00:54.755][INFO][RK0][main]: The size of worker memory pool: 2
[HCTR][12:00:54.755][INFO][RK0][main]: The size of refresh memory pool: 1
[HCTR][12:00:54.755][INFO][RK0][main]: The refresh percentage : 0.000000
Subprocess secondary awaiting initialization...
Main process; awaiting subprocess initializatiopn... So far 0 initialized...
Subprocess secondary awaiting initialization...
[HCTR][12:00:55.750][INFO][RK0][main]: Creating lookup session for hps_demo on device: 0
Subprocess primary initialized
2022-12-13 12:00:55.835179528 [W:onnxruntime:, graph.cc:3559 CleanUnusedInitializersAndNodeArgs] Removing initializer 'key_to_indice_hash_all_tables'. It is not used by any node and should be removed from the model.
Subprocess primary; ground_truth: [0.52604 0.528162 0.510473 ... 0.511216 0.464687 0.420649]
Subprocess primary; pred: [[0.5260405 ]
[0.52816164]
[0.5104735 ]
...
[0.5112164 ]
[0.46468708]
[0.42064884]]
Subprocess primary; mse between pred and ground_truth: 8.653384858862709e-14
Subprocess primary; pred_ref: [[0.5260405 ]
[0.52816164]
[0.5104735 ]
...
[0.5112164 ]
[0.46468708]
[0.42064884]]
Subprocess primary; mse between pred_ref and ground_truth: 8.653384858862709e-14
Subprocess primary exiting...
Main process; awaiting subprocess initializatiopn... So far 1 initialized...
[HCTR][12:00:55.964][WARNING][RK0][main]: default_value_for_each_table.size() is not equal to the number of embedding tables
====================================================HPS Create====================================================
[HCTR][12:00:55.965][INFO][RK0][main]: Creating Multi-Process HashMap CPU database backend...
[HCTR][12:00:55.965][WARNING][RK0][main]: default_value_for_each_table.size() is not equal to the number of embedding tables
[HCTR][12:00:55.966][INFO][RK0][main]: Connecting to shared memory 'hctr_mp_hash_map_database'...
====================================================HPS Create====================================================
[HCTR][12:00:55.966][INFO][RK0][main]: Creating Multi-Process HashMap CPU database backend...
[HCTR][12:00:55.966][INFO][RK0][main]: Connecting to shared memory 'hctr_mp_hash_map_database'...
[HCTR][12:00:55.966][INFO][RK0][main]: Connected to shared memory 'hctr_mp_hash_map_database'; OS total = 17179869184 bytes, OS available = 7913971712 bytes, HCTR allocated = 17179869184 bytes, HCTR free = 7914009984 bytes; other processes connected = 1
[HCTR][12:00:56.466][INFO][RK0][main]: Volatile DB: initial cache rate = 1
[HCTR][12:00:56.466][INFO][RK0][main]: Volatile DB: cache missed embeddings = 0
[HCTR][12:00:56.466][DEBUG][RK0][main]: Created raw model loader in local memory!
[HCTR][12:00:56.466][INFO][RK0][main]: Using Local file system backend.
[HCTR][12:00:56.469][INFO][RK0][main]: Using Local file system backend.
[HCTR][12:00:56.472][DEBUG][RK0][main]: Real-time subscribers created!
[HCTR][12:00:56.472][INFO][RK0][main]: Creating embedding cache in device 1.
[HCTR][12:00:56.481][INFO][RK0][main]: Model name: hps_demo
[HCTR][12:00:56.481][INFO][RK0][main]: Max batch size: 1024
[HCTR][12:00:56.481][INFO][RK0][main]: Number of embedding tables: 2
[HCTR][12:00:56.481][INFO][RK0][main]: Use GPU embedding cache: True, cache size percentage: 0.500000
[HCTR][12:00:56.481][INFO][RK0][main]: Use static table: False
[HCTR][12:00:56.481][INFO][RK0][main]: Use I64 input key: True
[HCTR][12:00:56.481][INFO][RK0][main]: Configured cache hit rate threshold: 1.000000
[HCTR][12:00:56.481][INFO][RK0][main]: The size of thread pool: 80
[HCTR][12:00:56.481][INFO][RK0][main]: The size of worker memory pool: 2
[HCTR][12:00:56.481][INFO][RK0][main]: The size of refresh memory pool: 1
[HCTR][12:00:56.481][INFO][RK0][main]: The refresh percentage : 0.000000
Main process; awaiting subprocess initializatiopn... So far 1 initialized...
[HCTR][12:00:56.466][INFO][RK0][main]: Connected to shared memory 'hctr_mp_hash_map_database'; OS total = 17179869184 bytes, OS available = 7913971712 bytes, HCTR allocated = 17179869184 bytes, HCTR free = 7914009984 bytes; other processes connected = 1
[HCTR][12:00:56.967][INFO][RK0][main]: Volatile DB: initial cache rate = 1
[HCTR][12:00:56.967][INFO][RK0][main]: Volatile DB: cache missed embeddings = 0
[HCTR][12:00:56.967][DEBUG][RK0][main]: Created raw model loader in local memory!
[HCTR][12:00:56.967][INFO][RK0][main]: Using Local file system backend.
[HCTR][12:00:56.969][INFO][RK0][main]: Using Local file system backend.
[HCTR][12:00:56.972][DEBUG][RK0][main]: Real-time subscribers created!
[HCTR][12:00:56.972][INFO][RK0][main]: Creating embedding cache in device 2.
[HCTR][12:00:56.980][INFO][RK0][main]: Model name: hps_demo
[HCTR][12:00:56.980][INFO][RK0][main]: Max batch size: 1024
[HCTR][12:00:56.980][INFO][RK0][main]: Number of embedding tables: 2
[HCTR][12:00:56.980][INFO][RK0][main]: Use GPU embedding cache: True, cache size percentage: 0.500000
[HCTR][12:00:56.980][INFO][RK0][main]: Use static table: False
[HCTR][12:00:56.980][INFO][RK0][main]: Use I64 input key: True
[HCTR][12:00:56.980][INFO][RK0][main]: Configured cache hit rate threshold: 1.000000
[HCTR][12:00:56.980][INFO][RK0][main]: The size of thread pool: 80
[HCTR][12:00:56.980][INFO][RK0][main]: The size of worker memory pool: 2
[HCTR][12:00:56.980][INFO][RK0][main]: The size of refresh memory pool: 1
[HCTR][12:00:56.980][INFO][RK0][main]: The refresh percentage : 0.000000
Main process; awaiting subprocess initializatiopn... So far 1 initialized...
[HCTR][12:00:58.465][INFO][RK0][main]: Creating lookup session for hps_demo on device: 1
Subprocess secondary initialized
2022-12-13 12:00:58.556145398 [W:onnxruntime:, graph.cc:3559 CleanUnusedInitializersAndNodeArgs] Removing initializer 'key_to_indice_hash_all_tables'. It is not used by any node and should be removed from the model.
Subprocess secondary; ground_truth: [0.52604 0.528162 0.510473 ... 0.511216 0.464687 0.420649]
Subprocess secondary; pred: [[0.5260405 ]
[0.52816164]
[0.5104735 ]
...
[0.5112164 ]
[0.46468708]
[0.42064884]]
Subprocess secondary; mse between pred and ground_truth: 8.653384858862709e-14
Subprocess secondary; pred_ref: [[0.5260405 ]
[0.52816164]
[0.5104735 ]
...
[0.5112164 ]
[0.46468708]
[0.42064884]]
Subprocess secondary; mse between pred_ref and ground_truth: 8.653384858862709e-14
Subprocess secondary exiting...
Main process; awaiting subprocess initializatiopn... So far 2 initialized...
[HCTR][12:00:59.131][INFO][RK0][main]: Creating lookup session for hps_demo on device: 2
Subprocess secondary initialized
2022-12-13 12:00:59.192390075 [W:onnxruntime:, graph.cc:3559 CleanUnusedInitializersAndNodeArgs] Removing initializer 'key_to_indice_hash_all_tables'. It is not used by any node and should be removed from the model.
Subprocess secondary; ground_truth: [0.52604 0.528162 0.510473 ... 0.511216 0.464687 0.420649]
Subprocess secondary; pred: [[0.5260405 ]
[0.52816164]
[0.5104735 ]
...
[0.5112164 ]
[0.46468708]
[0.42064884]]
Subprocess secondary; mse between pred and ground_truth: 8.653384858862709e-14
Subprocess secondary; pred_ref: [[0.5260405 ]
[0.52816164]
[0.5104735 ]
...
[0.5112164 ]
[0.46468708]
[0.42064884]]
Subprocess secondary; mse between pred_ref and ground_truth: 8.653384858862709e-14
Subprocess secondary exiting...
Main process; awaiting subprocess 0 to exit...
[HCTR][12:01:05.906][INFO][RK0][main]: Disconnecting from shared memory 'hctr_mp_hash_map_database'.
Main process; awaiting subprocess 1 to exit...
[HCTR][12:01:08.627][INFO][RK0][main]: Disconnecting from shared memory 'hctr_mp_hash_map_database'.
[HCTR][12:01:09.261][INFO][RK0][main]: Disconnecting from shared memory 'hctr_mp_hash_map_database'.
Main process; awaiting subprocess 2 to exit...
[HCTR][12:01:09.781][INFO][RK0][main]: Detached last process from shared memory 'hctr_mp_hash_map_database'. Auto remove in progress...
Main process; exiting...
Redis Cluster deployment (without TLS/SSL)
HugeCTR can use Redis clusters as backing storage. In the following steps we show how to setup a mock Redis / HugeCTR deployment in a single machine. We assume that you have started this notebook in a HugeCTR docker container.
Step 1: Get + build Redis
!wget https://github.com/redis/redis/archive/7.0.5.tar.gz
!tar -xf 7.0.5.tar.gz && rm -f 7.0.5.tar.gz
![ -f redis-7.0.5 ] && rm -rf redis-7.0.5
!cd redis-7.0.5 && make BUILD_TLS=yes
--2022-12-08 12:13:59-- https://github.com/redis/redis/archive/7.0.5.tar.gz
Resolving github.com (github.com)... 192.30.255.113
Connecting to github.com (github.com)|192.30.255.113|:443... connected.
HTTP request sent, awaiting response... 302 Found
Location: https://codeload.github.com/redis/redis/tar.gz/refs/tags/7.0.5 [following]
--2022-12-08 12:13:59-- https://codeload.github.com/redis/redis/tar.gz/refs/tags/7.0.5
Resolving codeload.github.com (codeload.github.com)... 192.30.255.120
Connecting to codeload.github.com (codeload.github.com)|192.30.255.120|:443... connected.
HTTP request sent, awaiting response... 200 OK
Length: 2998759 (2.9M) [application/x-gzip]
Saving to: ‘7.0.5.tar.gz’
7.0.5.tar.gz 100%[===================>] 2.86M 18.1MB/s in 0.2s
2022-12-08 12:13:59 (18.1 MB/s) - ‘7.0.5.tar.gz’ saved [2998759/2998759]
cd src && make all
make[1]: Entering directory '/scratch/proj/hugectr/notebooks/redis-7.0.5/src'
./mkreleasehdr.sh: 1: echo: echo: I/O error
CC Makefile.dep
./mkreleasehdr.sh: 1: echo: echo: I/O error
rm -rf redis-server redis-sentinel redis-cli redis-benchmark redis-check-rdb redis-check-aof *.o *.gcda *.gcno *.gcov redis.info lcov-html Makefile.dep
rm -f adlist.d quicklist.d ae.d anet.d dict.d server.d sds.d zmalloc.d lzf_c.d lzf_d.d pqsort.d zipmap.d sha1.d ziplist.d release.d networking.d util.d object.d db.d replication.d rdb.d t_string.d t_list.d t_set.d t_zset.d t_hash.d config.d aof.d pubsub.d multi.d debug.d sort.d intset.d syncio.d cluster.d crc16.d endianconv.d slowlog.d eval.d bio.d rio.d rand.d memtest.d syscheck.d crcspeed.d crc64.d bitops.d sentinel.d notify.d setproctitle.d blocked.d hyperloglog.d latency.d sparkline.d redis-check-rdb.d redis-check-aof.d geo.d lazyfree.d module.d evict.d expire.d geohash.d geohash_helper.d childinfo.d defrag.d siphash.d rax.d t_stream.d listpack.d localtime.d lolwut.d lolwut5.d lolwut6.d acl.d tracking.d connection.d tls.d sha256.d timeout.d setcpuaffinity.d monotonic.d mt19937-64.d resp_parser.d call_reply.d script_lua.d script.d functions.d function_lua.d commands.d anet.d adlist.d dict.d redis-cli.d zmalloc.d release.d ae.d redisassert.d crcspeed.d crc64.d siphash.d crc16.d monotonic.d cli_common.d mt19937-64.d ae.d anet.d redis-benchmark.d adlist.d dict.d zmalloc.d redisassert.d release.d crcspeed.d crc64.d siphash.d crc16.d monotonic.d cli_common.d mt19937-64.d
(cd ../deps && make distclean)
make[2]: Entering directory '/scratch/proj/hugectr/notebooks/redis-7.0.5/deps'
(cd hiredis && make clean) > /dev/null || true
(cd linenoise && make clean) > /dev/null || true
(cd lua && make clean) > /dev/null || true
(cd jemalloc && [ -f Makefile ] && make distclean) > /dev/null || true
(cd hdr_histogram && make clean) > /dev/null || true
(rm -f .make-*)
make[2]: Leaving directory '/scratch/proj/hugectr/notebooks/redis-7.0.5/deps'
(cd modules && make clean)
make[2]: Entering directory '/scratch/proj/hugectr/notebooks/redis-7.0.5/src/modules'
rm -rf *.xo *.so
make[2]: Leaving directory '/scratch/proj/hugectr/notebooks/redis-7.0.5/src/modules'
(cd ../tests/modules && make clean)
make[2]: Entering directory '/scratch/proj/hugectr/notebooks/redis-7.0.5/tests/modules'
rm -f commandfilter.so basics.so testrdb.so fork.so infotest.so propagate.so misc.so hooks.so blockonkeys.so blockonbackground.so scan.so datatype.so datatype2.so auth.so keyspace_events.so blockedclient.so getkeys.so getchannels.so test_lazyfree.so timer.so defragtest.so keyspecs.so hash.so zset.so stream.so mallocsize.so aclcheck.so list.so subcommands.so reply.so cmdintrospection.so eventloop.so moduleconfigs.so moduleconfigstwo.so publish.so commandfilter.xo basics.xo testrdb.xo fork.xo infotest.xo propagate.xo misc.xo hooks.xo blockonkeys.xo blockonbackground.xo scan.xo datatype.xo datatype2.xo auth.xo keyspace_events.xo blockedclient.xo getkeys.xo getchannels.xo test_lazyfree.xo timer.xo defragtest.xo keyspecs.xo hash.xo zset.xo stream.xo mallocsize.xo aclcheck.xo list.xo subcommands.xo reply.xo cmdintrospection.xo eventloop.xo moduleconfigs.xo moduleconfigstwo.xo publish.xo
make[2]: Leaving directory '/scratch/proj/hugectr/notebooks/redis-7.0.5/tests/modules'
(rm -f .make-*)
echo STD=-pedantic -DREDIS_STATIC='' -std=c11 >> .make-settings
echo WARN=-Wall -W -Wno-missing-field-initializers >> .make-settings
echo OPT=-O2 >> .make-settings
echo MALLOC=jemalloc >> .make-settings
echo BUILD_TLS=yes >> .make-settings
echo USE_SYSTEMD= >> .make-settings
echo CFLAGS= >> .make-settings
echo LDFLAGS= >> .make-settings
echo REDIS_CFLAGS= >> .make-settings
echo REDIS_LDFLAGS= >> .make-settings
echo PREV_FINAL_CFLAGS=-pedantic -DREDIS_STATIC='' -std=c11 -Wall -W -Wno-missing-field-initializers -O2 -g -ggdb -I../deps/hiredis -I../deps/linenoise -I../deps/lua/src -I../deps/hdr_histogram -DUSE_JEMALLOC -I../deps/jemalloc/include -DUSE_OPENSSL >> .make-settings
echo PREV_FINAL_LDFLAGS= -g -ggdb -rdynamic >> .make-settings
(cd ../deps && make hiredis linenoise lua hdr_histogram jemalloc)
make[2]: Entering directory '/scratch/proj/hugectr/notebooks/redis-7.0.5/deps'
(cd hiredis && make clean) > /dev/null || true
(cd linenoise && make clean) > /dev/null || true
(cd lua && make clean) > /dev/null || true
(cd jemalloc && [ -f Makefile ] && make distclean) > /dev/null || true
(cd hdr_histogram && make clean) > /dev/null || true
(rm -f .make-*)
(echo "" > .make-cflags)
(echo "" > .make-ldflags)
MAKE hiredis
cd hiredis && make static USE_SSL=1
make[3]: Entering directory '/scratch/proj/hugectr/notebooks/redis-7.0.5/deps/hiredis'
cc -std=c99 -c -O3 -fPIC -DHIREDIS_TEST_SSL -Wall -W -Wstrict-prototypes -Wwrite-strings -Wno-missing-field-initializers -g -ggdb -pedantic alloc.c
cc -std=c99 -c -O3 -fPIC -DHIREDIS_TEST_SSL -Wall -W -Wstrict-prototypes -Wwrite-strings -Wno-missing-field-initializers -g -ggdb -pedantic net.c
cc -std=c99 -c -O3 -fPIC -DHIREDIS_TEST_SSL -Wall -W -Wstrict-prototypes -Wwrite-strings -Wno-missing-field-initializers -g -ggdb -pedantic hiredis.c
cc -std=c99 -c -O3 -fPIC -DHIREDIS_TEST_SSL -Wall -W -Wstrict-prototypes -Wwrite-strings -Wno-missing-field-initializers -g -ggdb -pedantic sds.c
cc -std=c99 -c -O3 -fPIC -DHIREDIS_TEST_SSL -Wall -W -Wstrict-prototypes -Wwrite-strings -Wno-missing-field-initializers -g -ggdb -pedantic async.c
cc -std=c99 -c -O3 -fPIC -DHIREDIS_TEST_SSL -Wall -W -Wstrict-prototypes -Wwrite-strings -Wno-missing-field-initializers -g -ggdb -pedantic read.c
cc -std=c99 -c -O3 -fPIC -DHIREDIS_TEST_SSL -Wall -W -Wstrict-prototypes -Wwrite-strings -Wno-missing-field-initializers -g -ggdb -pedantic sockcompat.c
ar rcs libhiredis.a alloc.o net.o hiredis.o sds.o async.o read.o sockcompat.o
cc -std=c99 -c -O3 -fPIC -DHIREDIS_TEST_SSL -Wall -W -Wstrict-prototypes -Wwrite-strings -Wno-missing-field-initializers -g -ggdb -pedantic ssl.c
ar rcs libhiredis_ssl.a ssl.o
make[3]: Leaving directory '/scratch/proj/hugectr/notebooks/redis-7.0.5/deps/hiredis'
MAKE linenoise
cd linenoise && make
make[3]: Entering directory '/scratch/proj/hugectr/notebooks/redis-7.0.5/deps/linenoise'
cc -Wall -Os -g -c linenoise.c
make[3]: Leaving directory '/scratch/proj/hugectr/notebooks/redis-7.0.5/deps/linenoise'
MAKE lua
cd lua/src && make all CFLAGS="-Wall -DLUA_ANSI -DENABLE_CJSON_GLOBAL -DREDIS_STATIC='' -DLUA_USE_MKSTEMP -O2 " MYLDFLAGS="" AR="ar rc"
make[3]: Entering directory '/scratch/proj/hugectr/notebooks/redis-7.0.5/deps/lua/src'
cc -Wall -DLUA_ANSI -DENABLE_CJSON_GLOBAL -DREDIS_STATIC='' -DLUA_USE_MKSTEMP -O2 -c -o lapi.o lapi.c
cc -Wall -DLUA_ANSI -DENABLE_CJSON_GLOBAL -DREDIS_STATIC='' -DLUA_USE_MKSTEMP -O2 -c -o lcode.o lcode.c
cc -Wall -DLUA_ANSI -DENABLE_CJSON_GLOBAL -DREDIS_STATIC='' -DLUA_USE_MKSTEMP -O2 -c -o ldebug.o ldebug.c
cc -Wall -DLUA_ANSI -DENABLE_CJSON_GLOBAL -DREDIS_STATIC='' -DLUA_USE_MKSTEMP -O2 -c -o ldo.o ldo.c
cc -Wall -DLUA_ANSI -DENABLE_CJSON_GLOBAL -DREDIS_STATIC='' -DLUA_USE_MKSTEMP -O2 -c -o ldump.o ldump.c
cc -Wall -DLUA_ANSI -DENABLE_CJSON_GLOBAL -DREDIS_STATIC='' -DLUA_USE_MKSTEMP -O2 -c -o lfunc.o lfunc.c
cc -Wall -DLUA_ANSI -DENABLE_CJSON_GLOBAL -DREDIS_STATIC='' -DLUA_USE_MKSTEMP -O2 -c -o lgc.o lgc.c
cc -Wall -DLUA_ANSI -DENABLE_CJSON_GLOBAL -DREDIS_STATIC='' -DLUA_USE_MKSTEMP -O2 -c -o llex.o llex.c
cc -Wall -DLUA_ANSI -DENABLE_CJSON_GLOBAL -DREDIS_STATIC='' -DLUA_USE_MKSTEMP -O2 -c -o lmem.o lmem.c
cc -Wall -DLUA_ANSI -DENABLE_CJSON_GLOBAL -DREDIS_STATIC='' -DLUA_USE_MKSTEMP -O2 -c -o lobject.o lobject.c
cc -Wall -DLUA_ANSI -DENABLE_CJSON_GLOBAL -DREDIS_STATIC='' -DLUA_USE_MKSTEMP -O2 -c -o lopcodes.o lopcodes.c
cc -Wall -DLUA_ANSI -DENABLE_CJSON_GLOBAL -DREDIS_STATIC='' -DLUA_USE_MKSTEMP -O2 -c -o lparser.o lparser.c
cc -Wall -DLUA_ANSI -DENABLE_CJSON_GLOBAL -DREDIS_STATIC='' -DLUA_USE_MKSTEMP -O2 -c -o lstate.o lstate.c
cc -Wall -DLUA_ANSI -DENABLE_CJSON_GLOBAL -DREDIS_STATIC='' -DLUA_USE_MKSTEMP -O2 -c -o lstring.o lstring.c
cc -Wall -DLUA_ANSI -DENABLE_CJSON_GLOBAL -DREDIS_STATIC='' -DLUA_USE_MKSTEMP -O2 -c -o ltable.o ltable.c
cc -Wall -DLUA_ANSI -DENABLE_CJSON_GLOBAL -DREDIS_STATIC='' -DLUA_USE_MKSTEMP -O2 -c -o ltm.o ltm.c
cc -Wall -DLUA_ANSI -DENABLE_CJSON_GLOBAL -DREDIS_STATIC='' -DLUA_USE_MKSTEMP -O2 -c -o lundump.o lundump.c
cc -Wall -DLUA_ANSI -DENABLE_CJSON_GLOBAL -DREDIS_STATIC='' -DLUA_USE_MKSTEMP -O2 -c -o lvm.o lvm.c
cc -Wall -DLUA_ANSI -DENABLE_CJSON_GLOBAL -DREDIS_STATIC='' -DLUA_USE_MKSTEMP -O2 -c -o lzio.o lzio.c
cc -Wall -DLUA_ANSI -DENABLE_CJSON_GLOBAL -DREDIS_STATIC='' -DLUA_USE_MKSTEMP -O2 -c -o strbuf.o strbuf.c
cc -Wall -DLUA_ANSI -DENABLE_CJSON_GLOBAL -DREDIS_STATIC='' -DLUA_USE_MKSTEMP -O2 -c -o fpconv.o fpconv.c
cc -Wall -DLUA_ANSI -DENABLE_CJSON_GLOBAL -DREDIS_STATIC='' -DLUA_USE_MKSTEMP -O2 -c -o lauxlib.o lauxlib.c
cc -Wall -DLUA_ANSI -DENABLE_CJSON_GLOBAL -DREDIS_STATIC='' -DLUA_USE_MKSTEMP -O2 -c -o lbaselib.o lbaselib.c
cc -Wall -DLUA_ANSI -DENABLE_CJSON_GLOBAL -DREDIS_STATIC='' -DLUA_USE_MKSTEMP -O2 -c -o ldblib.o ldblib.c
cc -Wall -DLUA_ANSI -DENABLE_CJSON_GLOBAL -DREDIS_STATIC='' -DLUA_USE_MKSTEMP -O2 -c -o liolib.o liolib.c
cc -Wall -DLUA_ANSI -DENABLE_CJSON_GLOBAL -DREDIS_STATIC='' -DLUA_USE_MKSTEMP -O2 -c -o lmathlib.o lmathlib.c
cc -Wall -DLUA_ANSI -DENABLE_CJSON_GLOBAL -DREDIS_STATIC='' -DLUA_USE_MKSTEMP -O2 -c -o loslib.o loslib.c
cc -Wall -DLUA_ANSI -DENABLE_CJSON_GLOBAL -DREDIS_STATIC='' -DLUA_USE_MKSTEMP -O2 -c -o ltablib.o ltablib.c
cc -Wall -DLUA_ANSI -DENABLE_CJSON_GLOBAL -DREDIS_STATIC='' -DLUA_USE_MKSTEMP -O2 -c -o lstrlib.o lstrlib.c
cc -Wall -DLUA_ANSI -DENABLE_CJSON_GLOBAL -DREDIS_STATIC='' -DLUA_USE_MKSTEMP -O2 -c -o loadlib.o loadlib.c
cc -Wall -DLUA_ANSI -DENABLE_CJSON_GLOBAL -DREDIS_STATIC='' -DLUA_USE_MKSTEMP -O2 -c -o linit.o linit.c
cc -Wall -DLUA_ANSI -DENABLE_CJSON_GLOBAL -DREDIS_STATIC='' -DLUA_USE_MKSTEMP -O2 -c -o lua_cjson.o lua_cjson.c
cc -Wall -DLUA_ANSI -DENABLE_CJSON_GLOBAL -DREDIS_STATIC='' -DLUA_USE_MKSTEMP -O2 -c -o lua_struct.o lua_struct.c
cc -Wall -DLUA_ANSI -DENABLE_CJSON_GLOBAL -DREDIS_STATIC='' -DLUA_USE_MKSTEMP -O2 -c -o lua_cmsgpack.o lua_cmsgpack.c
cc -Wall -DLUA_ANSI -DENABLE_CJSON_GLOBAL -DREDIS_STATIC='' -DLUA_USE_MKSTEMP -O2 -c -o lua_bit.o lua_bit.c
ar rc liblua.a lapi.o lcode.o ldebug.o ldo.o ldump.o lfunc.o lgc.o llex.o lmem.o lobject.o lopcodes.o lparser.o lstate.o lstring.o ltable.o ltm.o lundump.o lvm.o lzio.o strbuf.o fpconv.o lauxlib.o lbaselib.o ldblib.o liolib.o lmathlib.o loslib.o ltablib.o lstrlib.o loadlib.o linit.o lua_cjson.o lua_struct.o lua_cmsgpack.o lua_bit.o # DLL needs all object files
ranlib liblua.a
cc -Wall -DLUA_ANSI -DENABLE_CJSON_GLOBAL -DREDIS_STATIC='' -DLUA_USE_MKSTEMP -O2 -c -o lua.o lua.c
cc -o lua lua.o liblua.a -lm
cc -Wall -DLUA_ANSI -DENABLE_CJSON_GLOBAL -DREDIS_STATIC='' -DLUA_USE_MKSTEMP -O2 -c -o luac.o luac.c
cc -Wall -DLUA_ANSI -DENABLE_CJSON_GLOBAL -DREDIS_STATIC='' -DLUA_USE_MKSTEMP -O2 -c -o print.o print.c
cc -o luac luac.o print.o liblua.a -lm
make[3]: Leaving directory '/scratch/proj/hugectr/notebooks/redis-7.0.5/deps/lua/src'
MAKE hdr_histogram
cd hdr_histogram && make
make[3]: Entering directory '/scratch/proj/hugectr/notebooks/redis-7.0.5/deps/hdr_histogram'
cc -std=c99 -Wall -Os -g -DHDR_MALLOC_INCLUDE=\"hdr_redis_malloc.h\" -c hdr_histogram.c
ar rcs libhdrhistogram.a hdr_histogram.o
make[3]: Leaving directory '/scratch/proj/hugectr/notebooks/redis-7.0.5/deps/hdr_histogram'
MAKE jemalloc
cd jemalloc && ./configure --with-version=5.2.1-0-g0 --with-lg-quantum=3 --with-jemalloc-prefix=je_ CFLAGS="-std=gnu99 -Wall -pipe -g3 -O3 -funroll-loops " LDFLAGS=""
checking for xsltproc... false
checking for gcc... gcc
checking whether the C compiler works... yes
checking for C compiler default output file name... a.out
checking for suffix of executables...
checking whether we are cross compiling... no
checking for suffix of object files... o
checking whether we are using the GNU C compiler... yes
checking whether gcc accepts -g... yes
checking for gcc option to accept ISO C89... none needed
checking whether compiler is cray... no
checking whether compiler supports -std=gnu11... yes
checking whether compiler supports -Wall... yes
checking whether compiler supports -Wextra... yes
checking whether compiler supports -Wshorten-64-to-32... no
checking whether compiler supports -Wsign-compare... yes
checking whether compiler supports -Wundef... yes
checking whether compiler supports -Wno-format-zero-length... yes
checking whether compiler supports -pipe... yes
checking whether compiler supports -g3... yes
checking how to run the C preprocessor... gcc -E
checking for g++... g++
checking whether we are using the GNU C++ compiler... yes
checking whether g++ accepts -g... yes
checking whether g++ supports C++14 features by default... yes
checking whether compiler supports -Wall... yes
checking whether compiler supports -Wextra... yes
checking whether compiler supports -g3... yes
checking whether libstdc++ linkage is compilable... yes
checking for grep that handles long lines and -e... /usr/bin/grep
checking for egrep... /usr/bin/grep -E
checking for ANSI C header files... yes
checking for sys/types.h... yes
checking for sys/stat.h... yes
checking for stdlib.h... yes
checking for string.h... yes
checking for memory.h... yes
checking for strings.h... yes
checking for inttypes.h... yes
checking for stdint.h... yes
checking for unistd.h... yes
checking whether byte ordering is bigendian... no
checking size of void *... 8
checking size of int... 4
checking size of long... 8
checking size of long long... 8
checking size of intmax_t... 8
checking build system type... x86_64-pc-linux-gnu
checking host system type... x86_64-pc-linux-gnu
checking whether pause instruction is compilable... yes
checking number of significant virtual address bits... 48
checking for ar... ar
checking for nm... nm
checking for gawk... no
checking for mawk... mawk
checking malloc.h usability... yes
checking malloc.h presence... yes
checking for malloc.h... yes
checking whether malloc_usable_size definition can use const argument... no
checking for library containing log... -lm
checking whether __attribute__ syntax is compilable... yes
checking whether compiler supports -fvisibility=hidden... yes
checking whether compiler supports -fvisibility=hidden... yes
checking whether compiler supports -Werror... yes
checking whether compiler supports -herror_on_warning... no
checking whether tls_model attribute is compilable... yes
checking whether compiler supports -Werror... yes
checking whether compiler supports -herror_on_warning... no
checking whether alloc_size attribute is compilable... yes
checking whether compiler supports -Werror... yes
checking whether compiler supports -herror_on_warning... no
checking whether format(gnu_printf, ...) attribute is compilable... yes
checking whether compiler supports -Werror... yes
checking whether compiler supports -herror_on_warning... no
checking whether format(printf, ...) attribute is compilable... yes
checking whether compiler supports -Werror... yes
checking whether compiler supports -herror_on_warning... no
checking whether format(printf, ...) attribute is compilable... yes
checking for a BSD-compatible install... /usr/bin/install -c
checking for ranlib... ranlib
checking for ld... /usr/bin/ld
checking for autoconf... /usr/bin/autoconf
checking for memalign... yes
checking for valloc... yes
checking whether compiler supports -O3... yes
checking whether compiler supports -O3... yes
checking whether compiler supports -funroll-loops... yes
checking configured backtracing method... N/A
checking for sbrk... yes
checking whether utrace(2) is compilable... no
checking whether a program using __builtin_unreachable is compilable... yes
checking whether a program using __builtin_ffsl is compilable... yes
checking whether a program using __builtin_popcountl is compilable... yes
checking LG_PAGE... 12
checking pthread.h usability... yes
checking pthread.h presence... yes
checking for pthread.h... yes
checking for pthread_create in -lpthread... yes
checking dlfcn.h usability... yes
checking dlfcn.h presence... yes
checking for dlfcn.h... yes
checking for dlsym... no
checking for dlsym in -ldl... yes
checking whether pthread_atfork(3) is compilable... yes
checking whether pthread_setname_np(3) is compilable... yes
checking for library containing clock_gettime... none required
checking whether clock_gettime(CLOCK_MONOTONIC_COARSE, ...) is compilable... yes
checking whether clock_gettime(CLOCK_MONOTONIC, ...) is compilable... yes
checking whether mach_absolute_time() is compilable... no
checking whether compiler supports -Werror... yes
checking whether syscall(2) is compilable... yes
checking for secure_getenv... yes
checking for sched_getcpu... yes
checking for sched_setaffinity... yes
checking for issetugid... no
checking for _malloc_thread_cleanup... no
checking for _pthread_mutex_init_calloc_cb... no
checking for TLS... yes
checking whether C11 atomics is compilable... no
checking whether GCC __atomic atomics is compilable... yes
checking whether GCC 8-bit __atomic atomics is compilable... yes
checking whether GCC __sync atomics is compilable... yes
checking whether GCC 8-bit __sync atomics is compilable... yes
checking whether Darwin OSAtomic*() is compilable... no
checking whether madvise(2) is compilable... yes
checking whether madvise(..., MADV_FREE) is compilable... yes
checking whether madvise(..., MADV_DONTNEED) is compilable... yes
checking whether madvise(..., MADV_DO[NT]DUMP) is compilable... yes
checking whether madvise(..., MADV_[NO]HUGEPAGE) is compilable... yes
checking for __builtin_clz... yes
checking whether Darwin os_unfair_lock_*() is compilable... no
checking whether glibc malloc hook is compilable... yes
checking whether glibc memalign hook is compilable... yes
checking whether pthreads adaptive mutexes is compilable... yes
checking whether compiler supports -D_GNU_SOURCE... yes
checking whether compiler supports -Werror... yes
checking whether compiler supports -herror_on_warning... no
checking whether strerror_r returns char with gnu source is compilable... yes
checking for stdbool.h that conforms to C99... yes
checking for _Bool... yes
configure: creating ./config.status
config.status: creating Makefile
config.status: creating jemalloc.pc
config.status: creating doc/html.xsl
config.status: creating doc/manpages.xsl
config.status: creating doc/jemalloc.xml
config.status: creating include/jemalloc/jemalloc_macros.h
config.status: creating include/jemalloc/jemalloc_protos.h
config.status: creating include/jemalloc/jemalloc_typedefs.h
config.status: creating include/jemalloc/internal/jemalloc_preamble.h
config.status: creating test/test.sh
config.status: creating test/include/test/jemalloc_test.h
config.status: creating config.stamp
config.status: creating bin/jemalloc-config
config.status: creating bin/jemalloc.sh
config.status: creating bin/jeprof
config.status: creating include/jemalloc/jemalloc_defs.h
config.status: creating include/jemalloc/internal/jemalloc_internal_defs.h
config.status: creating test/include/test/jemalloc_test_defs.h
config.status: executing include/jemalloc/internal/public_symbols.txt commands
config.status: executing include/jemalloc/internal/private_symbols.awk commands
config.status: executing include/jemalloc/internal/private_symbols_jet.awk commands
config.status: executing include/jemalloc/internal/public_namespace.h commands
config.status: executing include/jemalloc/internal/public_unnamespace.h commands
config.status: executing include/jemalloc/jemalloc_protos_jet.h commands
config.status: executing include/jemalloc/jemalloc_rename.h commands
config.status: executing include/jemalloc/jemalloc_mangle.h commands
config.status: executing include/jemalloc/jemalloc_mangle_jet.h commands
config.status: executing include/jemalloc/jemalloc.h commands
===============================================================================
jemalloc version : 5.2.1-0-g0
library revision : 2
CONFIG : --with-version=5.2.1-0-g0 --with-lg-quantum=3 --with-jemalloc-prefix=je_ 'CFLAGS=-std=gnu99 -Wall -pipe -g3 -O3 -funroll-loops ' LDFLAGS=
CC : gcc
CONFIGURE_CFLAGS : -std=gnu11 -Wall -Wextra -Wsign-compare -Wundef -Wno-format-zero-length -pipe -g3 -fvisibility=hidden -O3 -funroll-loops
SPECIFIED_CFLAGS : -std=gnu99 -Wall -pipe -g3 -O3 -funroll-loops
EXTRA_CFLAGS :
CPPFLAGS : -D_GNU_SOURCE -D_REENTRANT
CXX : g++
CONFIGURE_CXXFLAGS : -Wall -Wextra -g3 -fvisibility=hidden -O3
SPECIFIED_CXXFLAGS :
EXTRA_CXXFLAGS :
LDFLAGS :
EXTRA_LDFLAGS :
DSO_LDFLAGS : -shared -Wl,-soname,$(@F)
LIBS : -lm -lstdc++ -pthread -ldl
RPATH_EXTRA :
XSLTPROC : false
XSLROOT :
PREFIX : /usr/local
BINDIR : /usr/local/bin
DATADIR : /usr/local/share
INCLUDEDIR : /usr/local/include
LIBDIR : /usr/local/lib
MANDIR : /usr/local/share/man
srcroot :
abs_srcroot : /scratch/proj/hugectr/notebooks/redis-7.0.5/deps/jemalloc/
objroot :
abs_objroot : /scratch/proj/hugectr/notebooks/redis-7.0.5/deps/jemalloc/
JEMALLOC_PREFIX : je_
JEMALLOC_PRIVATE_NAMESPACE
: je_
install_suffix :
malloc_conf :
documentation : 1
shared libs : 1
static libs : 1
autogen : 0
debug : 0
stats : 1
experimetal_smallocx : 0
prof : 0
prof-libunwind : 0
prof-libgcc : 0
prof-gcc : 0
fill : 1
utrace : 0
xmalloc : 0
log : 0
lazy_lock : 0
cache-oblivious : 1
cxx : 1
===============================================================================
cd jemalloc && make CFLAGS="-std=gnu99 -Wall -pipe -g3 -O3 -funroll-loops " LDFLAGS="" lib/libjemalloc.a
make[3]: Entering directory '/scratch/proj/hugectr/notebooks/redis-7.0.5/deps/jemalloc'
gcc -std=gnu99 -Wall -pipe -g3 -O3 -funroll-loops -c -D_GNU_SOURCE -D_REENTRANT -Iinclude -Iinclude -DJEMALLOC_NO_PRIVATE_NAMESPACE -o src/jemalloc.sym.o src/jemalloc.c
nm -a src/jemalloc.sym.o | mawk -f include/jemalloc/internal/private_symbols.awk > src/jemalloc.sym
gcc -std=gnu99 -Wall -pipe -g3 -O3 -funroll-loops -c -D_GNU_SOURCE -D_REENTRANT -Iinclude -Iinclude -DJEMALLOC_NO_PRIVATE_NAMESPACE -o src/arena.sym.o src/arena.c
nm -a src/arena.sym.o | mawk -f include/jemalloc/internal/private_symbols.awk > src/arena.sym
gcc -std=gnu99 -Wall -pipe -g3 -O3 -funroll-loops -c -D_GNU_SOURCE -D_REENTRANT -Iinclude -Iinclude -DJEMALLOC_NO_PRIVATE_NAMESPACE -o src/background_thread.sym.o src/background_thread.c
nm -a src/background_thread.sym.o | mawk -f include/jemalloc/internal/private_symbols.awk > src/background_thread.sym
gcc -std=gnu99 -Wall -pipe -g3 -O3 -funroll-loops -c -D_GNU_SOURCE -D_REENTRANT -Iinclude -Iinclude -DJEMALLOC_NO_PRIVATE_NAMESPACE -o src/base.sym.o src/base.c
nm -a src/base.sym.o | mawk -f include/jemalloc/internal/private_symbols.awk > src/base.sym
gcc -std=gnu99 -Wall -pipe -g3 -O3 -funroll-loops -c -D_GNU_SOURCE -D_REENTRANT -Iinclude -Iinclude -DJEMALLOC_NO_PRIVATE_NAMESPACE -o src/bin.sym.o src/bin.c
nm -a src/bin.sym.o | mawk -f include/jemalloc/internal/private_symbols.awk > src/bin.sym
gcc -std=gnu99 -Wall -pipe -g3 -O3 -funroll-loops -c -D_GNU_SOURCE -D_REENTRANT -Iinclude -Iinclude -DJEMALLOC_NO_PRIVATE_NAMESPACE -o src/bitmap.sym.o src/bitmap.c
nm -a src/bitmap.sym.o | mawk -f include/jemalloc/internal/private_symbols.awk > src/bitmap.sym
gcc -std=gnu99 -Wall -pipe -g3 -O3 -funroll-loops -c -D_GNU_SOURCE -D_REENTRANT -Iinclude -Iinclude -DJEMALLOC_NO_PRIVATE_NAMESPACE -o src/ckh.sym.o src/ckh.c
nm -a src/ckh.sym.o | mawk -f include/jemalloc/internal/private_symbols.awk > src/ckh.sym
gcc -std=gnu99 -Wall -pipe -g3 -O3 -funroll-loops -c -D_GNU_SOURCE -D_REENTRANT -Iinclude -Iinclude -DJEMALLOC_NO_PRIVATE_NAMESPACE -o src/ctl.sym.o src/ctl.c
nm -a src/ctl.sym.o | mawk -f include/jemalloc/internal/private_symbols.awk > src/ctl.sym
gcc -std=gnu99 -Wall -pipe -g3 -O3 -funroll-loops -c -D_GNU_SOURCE -D_REENTRANT -Iinclude -Iinclude -DJEMALLOC_NO_PRIVATE_NAMESPACE -o src/div.sym.o src/div.c
nm -a src/div.sym.o | mawk -f include/jemalloc/internal/private_symbols.awk > src/div.sym
gcc -std=gnu99 -Wall -pipe -g3 -O3 -funroll-loops -c -D_GNU_SOURCE -D_REENTRANT -Iinclude -Iinclude -DJEMALLOC_NO_PRIVATE_NAMESPACE -o src/extent.sym.o src/extent.c
nm -a src/extent.sym.o | mawk -f include/jemalloc/internal/private_symbols.awk > src/extent.sym
gcc -std=gnu99 -Wall -pipe -g3 -O3 -funroll-loops -c -D_GNU_SOURCE -D_REENTRANT -Iinclude -Iinclude -DJEMALLOC_NO_PRIVATE_NAMESPACE -o src/extent_dss.sym.o src/extent_dss.c
nm -a src/extent_dss.sym.o | mawk -f include/jemalloc/internal/private_symbols.awk > src/extent_dss.sym
gcc -std=gnu99 -Wall -pipe -g3 -O3 -funroll-loops -c -D_GNU_SOURCE -D_REENTRANT -Iinclude -Iinclude -DJEMALLOC_NO_PRIVATE_NAMESPACE -o src/extent_mmap.sym.o src/extent_mmap.c
nm -a src/extent_mmap.sym.o | mawk -f include/jemalloc/internal/private_symbols.awk > src/extent_mmap.sym
gcc -std=gnu99 -Wall -pipe -g3 -O3 -funroll-loops -c -D_GNU_SOURCE -D_REENTRANT -Iinclude -Iinclude -DJEMALLOC_NO_PRIVATE_NAMESPACE -o src/hash.sym.o src/hash.c
nm -a src/hash.sym.o | mawk -f include/jemalloc/internal/private_symbols.awk > src/hash.sym
gcc -std=gnu99 -Wall -pipe -g3 -O3 -funroll-loops -c -D_GNU_SOURCE -D_REENTRANT -Iinclude -Iinclude -DJEMALLOC_NO_PRIVATE_NAMESPACE -o src/hook.sym.o src/hook.c
nm -a src/hook.sym.o | mawk -f include/jemalloc/internal/private_symbols.awk > src/hook.sym
gcc -std=gnu99 -Wall -pipe -g3 -O3 -funroll-loops -c -D_GNU_SOURCE -D_REENTRANT -Iinclude -Iinclude -DJEMALLOC_NO_PRIVATE_NAMESPACE -o src/large.sym.o src/large.c
nm -a src/large.sym.o | mawk -f include/jemalloc/internal/private_symbols.awk > src/large.sym
gcc -std=gnu99 -Wall -pipe -g3 -O3 -funroll-loops -c -D_GNU_SOURCE -D_REENTRANT -Iinclude -Iinclude -DJEMALLOC_NO_PRIVATE_NAMESPACE -o src/log.sym.o src/log.c
nm -a src/log.sym.o | mawk -f include/jemalloc/internal/private_symbols.awk > src/log.sym
gcc -std=gnu99 -Wall -pipe -g3 -O3 -funroll-loops -c -D_GNU_SOURCE -D_REENTRANT -Iinclude -Iinclude -DJEMALLOC_NO_PRIVATE_NAMESPACE -o src/malloc_io.sym.o src/malloc_io.c
nm -a src/malloc_io.sym.o | mawk -f include/jemalloc/internal/private_symbols.awk > src/malloc_io.sym
gcc -std=gnu99 -Wall -pipe -g3 -O3 -funroll-loops -c -D_GNU_SOURCE -D_REENTRANT -Iinclude -Iinclude -DJEMALLOC_NO_PRIVATE_NAMESPACE -o src/mutex.sym.o src/mutex.c
nm -a src/mutex.sym.o | mawk -f include/jemalloc/internal/private_symbols.awk > src/mutex.sym
gcc -std=gnu99 -Wall -pipe -g3 -O3 -funroll-loops -c -D_GNU_SOURCE -D_REENTRANT -Iinclude -Iinclude -DJEMALLOC_NO_PRIVATE_NAMESPACE -o src/mutex_pool.sym.o src/mutex_pool.c
nm -a src/mutex_pool.sym.o | mawk -f include/jemalloc/internal/private_symbols.awk > src/mutex_pool.sym
gcc -std=gnu99 -Wall -pipe -g3 -O3 -funroll-loops -c -D_GNU_SOURCE -D_REENTRANT -Iinclude -Iinclude -DJEMALLOC_NO_PRIVATE_NAMESPACE -o src/nstime.sym.o src/nstime.c
nm -a src/nstime.sym.o | mawk -f include/jemalloc/internal/private_symbols.awk > src/nstime.sym
gcc -std=gnu99 -Wall -pipe -g3 -O3 -funroll-loops -c -D_GNU_SOURCE -D_REENTRANT -Iinclude -Iinclude -DJEMALLOC_NO_PRIVATE_NAMESPACE -o src/pages.sym.o src/pages.c
nm -a src/pages.sym.o | mawk -f include/jemalloc/internal/private_symbols.awk > src/pages.sym
gcc -std=gnu99 -Wall -pipe -g3 -O3 -funroll-loops -c -D_GNU_SOURCE -D_REENTRANT -Iinclude -Iinclude -DJEMALLOC_NO_PRIVATE_NAMESPACE -o src/prng.sym.o src/prng.c
nm -a src/prng.sym.o | mawk -f include/jemalloc/internal/private_symbols.awk > src/prng.sym
gcc -std=gnu99 -Wall -pipe -g3 -O3 -funroll-loops -c -D_GNU_SOURCE -D_REENTRANT -Iinclude -Iinclude -DJEMALLOC_NO_PRIVATE_NAMESPACE -o src/prof.sym.o src/prof.c
nm -a src/prof.sym.o | mawk -f include/jemalloc/internal/private_symbols.awk > src/prof.sym
gcc -std=gnu99 -Wall -pipe -g3 -O3 -funroll-loops -c -D_GNU_SOURCE -D_REENTRANT -Iinclude -Iinclude -DJEMALLOC_NO_PRIVATE_NAMESPACE -o src/rtree.sym.o src/rtree.c
nm -a src/rtree.sym.o | mawk -f include/jemalloc/internal/private_symbols.awk > src/rtree.sym
gcc -std=gnu99 -Wall -pipe -g3 -O3 -funroll-loops -c -D_GNU_SOURCE -D_REENTRANT -Iinclude -Iinclude -DJEMALLOC_NO_PRIVATE_NAMESPACE -o src/safety_check.sym.o src/safety_check.c
nm -a src/safety_check.sym.o | mawk -f include/jemalloc/internal/private_symbols.awk > src/safety_check.sym
gcc -std=gnu99 -Wall -pipe -g3 -O3 -funroll-loops -c -D_GNU_SOURCE -D_REENTRANT -Iinclude -Iinclude -DJEMALLOC_NO_PRIVATE_NAMESPACE -o src/stats.sym.o src/stats.c
nm -a src/stats.sym.o | mawk -f include/jemalloc/internal/private_symbols.awk > src/stats.sym
gcc -std=gnu99 -Wall -pipe -g3 -O3 -funroll-loops -c -D_GNU_SOURCE -D_REENTRANT -Iinclude -Iinclude -DJEMALLOC_NO_PRIVATE_NAMESPACE -o src/sc.sym.o src/sc.c
nm -a src/sc.sym.o | mawk -f include/jemalloc/internal/private_symbols.awk > src/sc.sym
gcc -std=gnu99 -Wall -pipe -g3 -O3 -funroll-loops -c -D_GNU_SOURCE -D_REENTRANT -Iinclude -Iinclude -DJEMALLOC_NO_PRIVATE_NAMESPACE -o src/sz.sym.o src/sz.c
nm -a src/sz.sym.o | mawk -f include/jemalloc/internal/private_symbols.awk > src/sz.sym
gcc -std=gnu99 -Wall -pipe -g3 -O3 -funroll-loops -c -D_GNU_SOURCE -D_REENTRANT -Iinclude -Iinclude -DJEMALLOC_NO_PRIVATE_NAMESPACE -o src/tcache.sym.o src/tcache.c
nm -a src/tcache.sym.o | mawk -f include/jemalloc/internal/private_symbols.awk > src/tcache.sym
gcc -std=gnu99 -Wall -pipe -g3 -O3 -funroll-loops -c -D_GNU_SOURCE -D_REENTRANT -Iinclude -Iinclude -DJEMALLOC_NO_PRIVATE_NAMESPACE -o src/test_hooks.sym.o src/test_hooks.c
nm -a src/test_hooks.sym.o | mawk -f include/jemalloc/internal/private_symbols.awk > src/test_hooks.sym
gcc -std=gnu99 -Wall -pipe -g3 -O3 -funroll-loops -c -D_GNU_SOURCE -D_REENTRANT -Iinclude -Iinclude -DJEMALLOC_NO_PRIVATE_NAMESPACE -o src/ticker.sym.o src/ticker.c
nm -a src/ticker.sym.o | mawk -f include/jemalloc/internal/private_symbols.awk > src/ticker.sym
gcc -std=gnu99 -Wall -pipe -g3 -O3 -funroll-loops -c -D_GNU_SOURCE -D_REENTRANT -Iinclude -Iinclude -DJEMALLOC_NO_PRIVATE_NAMESPACE -o src/tsd.sym.o src/tsd.c
nm -a src/tsd.sym.o | mawk -f include/jemalloc/internal/private_symbols.awk > src/tsd.sym
gcc -std=gnu99 -Wall -pipe -g3 -O3 -funroll-loops -c -D_GNU_SOURCE -D_REENTRANT -Iinclude -Iinclude -DJEMALLOC_NO_PRIVATE_NAMESPACE -o src/witness.sym.o src/witness.c
nm -a src/witness.sym.o | mawk -f include/jemalloc/internal/private_symbols.awk > src/witness.sym
/bin/sh include/jemalloc/internal/private_namespace.sh src/jemalloc.sym src/arena.sym src/background_thread.sym src/base.sym src/bin.sym src/bitmap.sym src/ckh.sym src/ctl.sym src/div.sym src/extent.sym src/extent_dss.sym src/extent_mmap.sym src/hash.sym src/hook.sym src/large.sym src/log.sym src/malloc_io.sym src/mutex.sym src/mutex_pool.sym src/nstime.sym src/pages.sym src/prng.sym src/prof.sym src/rtree.sym src/safety_check.sym src/stats.sym src/sc.sym src/sz.sym src/tcache.sym src/test_hooks.sym src/ticker.sym src/tsd.sym src/witness.sym > include/jemalloc/internal/private_namespace.gen.h
cp include/jemalloc/internal/private_namespace.gen.h include/jemalloc/internal/private_namespace.gen.h
gcc -std=gnu99 -Wall -pipe -g3 -O3 -funroll-loops -c -D_GNU_SOURCE -D_REENTRANT -Iinclude -Iinclude -o src/jemalloc.o src/jemalloc.c
gcc -std=gnu99 -Wall -pipe -g3 -O3 -funroll-loops -c -D_GNU_SOURCE -D_REENTRANT -Iinclude -Iinclude -o src/arena.o src/arena.c
gcc -std=gnu99 -Wall -pipe -g3 -O3 -funroll-loops -c -D_GNU_SOURCE -D_REENTRANT -Iinclude -Iinclude -o src/background_thread.o src/background_thread.c
gcc -std=gnu99 -Wall -pipe -g3 -O3 -funroll-loops -c -D_GNU_SOURCE -D_REENTRANT -Iinclude -Iinclude -o src/base.o src/base.c
gcc -std=gnu99 -Wall -pipe -g3 -O3 -funroll-loops -c -D_GNU_SOURCE -D_REENTRANT -Iinclude -Iinclude -o src/bin.o src/bin.c
gcc -std=gnu99 -Wall -pipe -g3 -O3 -funroll-loops -c -D_GNU_SOURCE -D_REENTRANT -Iinclude -Iinclude -o src/bitmap.o src/bitmap.c
gcc -std=gnu99 -Wall -pipe -g3 -O3 -funroll-loops -c -D_GNU_SOURCE -D_REENTRANT -Iinclude -Iinclude -o src/ckh.o src/ckh.c
gcc -std=gnu99 -Wall -pipe -g3 -O3 -funroll-loops -c -D_GNU_SOURCE -D_REENTRANT -Iinclude -Iinclude -o src/ctl.o src/ctl.c
gcc -std=gnu99 -Wall -pipe -g3 -O3 -funroll-loops -c -D_GNU_SOURCE -D_REENTRANT -Iinclude -Iinclude -o src/div.o src/div.c
gcc -std=gnu99 -Wall -pipe -g3 -O3 -funroll-loops -c -D_GNU_SOURCE -D_REENTRANT -Iinclude -Iinclude -o src/extent.o src/extent.c
gcc -std=gnu99 -Wall -pipe -g3 -O3 -funroll-loops -c -D_GNU_SOURCE -D_REENTRANT -Iinclude -Iinclude -o src/extent_dss.o src/extent_dss.c
gcc -std=gnu99 -Wall -pipe -g3 -O3 -funroll-loops -c -D_GNU_SOURCE -D_REENTRANT -Iinclude -Iinclude -o src/extent_mmap.o src/extent_mmap.c
gcc -std=gnu99 -Wall -pipe -g3 -O3 -funroll-loops -c -D_GNU_SOURCE -D_REENTRANT -Iinclude -Iinclude -o src/hash.o src/hash.c
gcc -std=gnu99 -Wall -pipe -g3 -O3 -funroll-loops -c -D_GNU_SOURCE -D_REENTRANT -Iinclude -Iinclude -o src/hook.o src/hook.c
gcc -std=gnu99 -Wall -pipe -g3 -O3 -funroll-loops -c -D_GNU_SOURCE -D_REENTRANT -Iinclude -Iinclude -o src/large.o src/large.c
gcc -std=gnu99 -Wall -pipe -g3 -O3 -funroll-loops -c -D_GNU_SOURCE -D_REENTRANT -Iinclude -Iinclude -o src/log.o src/log.c
gcc -std=gnu99 -Wall -pipe -g3 -O3 -funroll-loops -c -D_GNU_SOURCE -D_REENTRANT -Iinclude -Iinclude -o src/malloc_io.o src/malloc_io.c
gcc -std=gnu99 -Wall -pipe -g3 -O3 -funroll-loops -c -D_GNU_SOURCE -D_REENTRANT -Iinclude -Iinclude -o src/mutex.o src/mutex.c
gcc -std=gnu99 -Wall -pipe -g3 -O3 -funroll-loops -c -D_GNU_SOURCE -D_REENTRANT -Iinclude -Iinclude -o src/mutex_pool.o src/mutex_pool.c
gcc -std=gnu99 -Wall -pipe -g3 -O3 -funroll-loops -c -D_GNU_SOURCE -D_REENTRANT -Iinclude -Iinclude -o src/nstime.o src/nstime.c
gcc -std=gnu99 -Wall -pipe -g3 -O3 -funroll-loops -c -D_GNU_SOURCE -D_REENTRANT -Iinclude -Iinclude -o src/pages.o src/pages.c
gcc -std=gnu99 -Wall -pipe -g3 -O3 -funroll-loops -c -D_GNU_SOURCE -D_REENTRANT -Iinclude -Iinclude -o src/prng.o src/prng.c
gcc -std=gnu99 -Wall -pipe -g3 -O3 -funroll-loops -c -D_GNU_SOURCE -D_REENTRANT -Iinclude -Iinclude -o src/prof.o src/prof.c
gcc -std=gnu99 -Wall -pipe -g3 -O3 -funroll-loops -c -D_GNU_SOURCE -D_REENTRANT -Iinclude -Iinclude -o src/rtree.o src/rtree.c
gcc -std=gnu99 -Wall -pipe -g3 -O3 -funroll-loops -c -D_GNU_SOURCE -D_REENTRANT -Iinclude -Iinclude -o src/safety_check.o src/safety_check.c
gcc -std=gnu99 -Wall -pipe -g3 -O3 -funroll-loops -c -D_GNU_SOURCE -D_REENTRANT -Iinclude -Iinclude -o src/stats.o src/stats.c
gcc -std=gnu99 -Wall -pipe -g3 -O3 -funroll-loops -c -D_GNU_SOURCE -D_REENTRANT -Iinclude -Iinclude -o src/sc.o src/sc.c
gcc -std=gnu99 -Wall -pipe -g3 -O3 -funroll-loops -c -D_GNU_SOURCE -D_REENTRANT -Iinclude -Iinclude -o src/sz.o src/sz.c
gcc -std=gnu99 -Wall -pipe -g3 -O3 -funroll-loops -c -D_GNU_SOURCE -D_REENTRANT -Iinclude -Iinclude -o src/tcache.o src/tcache.c
gcc -std=gnu99 -Wall -pipe -g3 -O3 -funroll-loops -c -D_GNU_SOURCE -D_REENTRANT -Iinclude -Iinclude -o src/test_hooks.o src/test_hooks.c
gcc -std=gnu99 -Wall -pipe -g3 -O3 -funroll-loops -c -D_GNU_SOURCE -D_REENTRANT -Iinclude -Iinclude -o src/ticker.o src/ticker.c
gcc -std=gnu99 -Wall -pipe -g3 -O3 -funroll-loops -c -D_GNU_SOURCE -D_REENTRANT -Iinclude -Iinclude -o src/tsd.o src/tsd.c
gcc -std=gnu99 -Wall -pipe -g3 -O3 -funroll-loops -c -D_GNU_SOURCE -D_REENTRANT -Iinclude -Iinclude -o src/witness.o src/witness.c
g++ -Wall -Wextra -g3 -fvisibility=hidden -O3 -c -D_GNU_SOURCE -D_REENTRANT -Iinclude -Iinclude -o src/jemalloc_cpp.o src/jemalloc_cpp.cpp
ar crus lib/libjemalloc.a src/jemalloc.o src/arena.o src/background_thread.o src/base.o src/bin.o src/bitmap.o src/ckh.o src/ctl.o src/div.o src/extent.o src/extent_dss.o src/extent_mmap.o src/hash.o src/hook.o src/large.o src/log.o src/malloc_io.o src/mutex.o src/mutex_pool.o src/nstime.o src/pages.o src/prng.o src/prof.o src/rtree.o src/safety_check.o src/stats.o src/sc.o src/sz.o src/tcache.o src/test_hooks.o src/ticker.o src/tsd.o src/witness.o src/jemalloc_cpp.o
ar: `u' modifier ignored since `D' is the default (see `U')
make[3]: Leaving directory '/scratch/proj/hugectr/notebooks/redis-7.0.5/deps/jemalloc'
make[2]: Leaving directory '/scratch/proj/hugectr/notebooks/redis-7.0.5/deps'
CC adlist.o
CC quicklist.o
CC ae.o
CC anet.o
CC dict.o
CC server.o
CC sds.o
CC zmalloc.o
CC lzf_c.o
CC lzf_d.o
CC pqsort.o
CC zipmap.o
CC sha1.o
CC ziplist.o
CC release.o
CC networking.o
CC util.o
CC object.o
CC db.o
CC replication.o
CC rdb.o
CC t_string.o
CC t_list.o
CC t_set.o
CC t_zset.o
CC t_hash.o
CC config.o
CC aof.o
CC pubsub.o
CC multi.o
CC debug.o
CC sort.o
CC intset.o
CC syncio.o
CC cluster.o
CC crc16.o
CC endianconv.o
CC slowlog.o
CC eval.o
CC bio.o
CC rio.o
CC rand.o
CC memtest.o
CC syscheck.o
CC crcspeed.o
CC crc64.o
CC bitops.o
CC sentinel.o
CC notify.o
CC setproctitle.o
CC blocked.o
CC hyperloglog.o
CC latency.o
CC sparkline.o
CC redis-check-rdb.o
CC redis-check-aof.o
CC geo.o
CC lazyfree.o
CC module.o
CC evict.o
CC expire.o
CC geohash.o
CC geohash_helper.o
CC childinfo.o
CC defrag.o
CC siphash.o
CC rax.o
CC t_stream.o
CC listpack.o
CC localtime.o
CC lolwut.o
CC lolwut5.o
CC lolwut6.o
CC acl.o
CC tracking.o
CC connection.o
CC tls.o
CC sha256.o
CC timeout.o
CC setcpuaffinity.o
CC monotonic.o
CC mt19937-64.o
CC resp_parser.o
CC call_reply.o
CC script_lua.o
CC script.o
CC functions.o
CC function_lua.o
CC commands.o
LINK redis-server
INSTALL redis-sentinel
CC redis-cli.o
CC redisassert.o
CC cli_common.o
LINK redis-cli
CC redis-benchmark.o
LINK redis-benchmark
INSTALL redis-check-rdb
INSTALL redis-check-aof
Hint: It's a good idea to run 'make test' ;)
make[1]: Leaving directory '/scratch/proj/hugectr/notebooks/redis-7.0.5/src'
If you see the message Hint: It's a good idea to run 'make test' ;)
followed by make[1]: Leaving directory ...
, the compilation should have completed successfully.
Step 2: Configure a mock Redis cluster
WARNING: The following commands will erase the all contents in the following directories: redis-server-1
, redis-server-2
and redis-server-3
.
!mkdir -p redis-server-1 redis-server-2 redis-server-3
!rm -f redis-server-1/* redis-server-2/* redis-server-3/*
!ln -sf $PWD/redis-7.0.5/src/redis-server redis-server-1/redis-server
!ln -sf $PWD/redis-7.0.5/src/redis-server redis-server-2/redis-server
!ln -sf $PWD/redis-7.0.5/src/redis-server redis-server-3/redis-server
%%writefile redis-server-1/redis.conf
daemonize yes
port 7000
cluster-enabled yes
cluster-config-file nodes.conf
appendonly no
save ""
Writing redis-server-1/redis.conf
%%writefile redis-server-2/redis.conf
daemonize yes
port 7001
cluster-enabled yes
cluster-config-file nodes.conf
appendonly no
save ""
Writing redis-server-2/redis.conf
%%writefile redis-server-3/redis.conf
daemonize yes
port 7002
cluster-enabled yes
cluster-config-file nodes.conf
appendonly no
save ""
Writing redis-server-3/redis.conf
Step 3: Form Redis cluster
WARNING: The following command will shutdown any processes called redis-cluster
in the current system!
# Shutdown existing cluster (if any).
!pkill redis-server
# Reset configuration and start 3 Redis servers.
!cd redis-server-1 && rm -f nodes.conf && ./redis-server redis.conf
!cd redis-server-2 && rm -f nodes.conf && ./redis-server redis.conf
!cd redis-server-3 && rm -f nodes.conf && ./redis-server redis.conf
# Form the cluster.
!redis-7.0.5/src/redis-cli \
--cluster create 127.0.0.1:7000 127.0.0.1:7001 127.0.0.1:7002 \
--cluster-yes
>>> Performing hash slots allocation on 3 nodes...
Master[0] -> Slots 0 - 5460
Master[1] -> Slots 5461 - 10922
Master[2] -> Slots 10923 - 16383
M: 746a01efe6afd6d0709859054e9845877e9d0571 127.0.0.1:7000
slots:[0-5460] (5461 slots) master
M: 8fdba8dc3f666d570291cd83ff14259e1513a904 127.0.0.1:7001
slots:[5461-10922] (5462 slots) master
M: 43222fc0adff160382ad5d868e0e270327df6c15 127.0.0.1:7002
slots:[10923-16383] (5461 slots) master
>>> Nodes configuration updated
>>> Assign a different config epoch to each node
>>> Sending CLUSTER MEET messages to join the cluster
Waiting for the cluster to join
.
>>> Performing Cluster Check (using node 127.0.0.1:7000)
M: 746a01efe6afd6d0709859054e9845877e9d0571 127.0.0.1:7000
slots:[0-5460] (5461 slots) master
M: 43222fc0adff160382ad5d868e0e270327df6c15 127.0.0.1:7002
slots:[10923-16383] (5461 slots) master
M: 8fdba8dc3f666d570291cd83ff14259e1513a904 127.0.0.1:7001
slots:[5461-10922] (5462 slots) master
[OK] All nodes agree about slots configuration.
>>> Check for open slots...
>>> Check slots coverage...
[OK] All 16384 slots covered.
Step 4: Run HugeCTR
import os
import time
import multiprocessing as mp
import pandas as pd
import numpy as np
import onnxruntime as ort
from hugectr import DatabaseType_t
from hugectr.inference import HPS, ParameterServerConfig, InferenceParams, VolatileDatabaseParams
slot_size_array = [10000, 10000, 10000, 10000]
key_offset = np.insert(np.cumsum(slot_size_array), 0, 0)[:-1]
batch_size = 2048
print('Launching...')
# 1. Configure the HPS hyperparameters.
ps_config = ParameterServerConfig(
emb_table_name = {'hps_demo': ['sparse_embedding1', 'sparse_embedding2']},
embedding_vec_size = {'hps_demo': [16, 32]},
max_feature_num_per_sample_per_emb_table = {'hps_demo': [2, 2]},
inference_params_array = [
InferenceParams(
model_name = 'hps_demo',
max_batchsize = batch_size,
hit_rate_threshold = 1.0,
dense_model_file = '',
sparse_model_files = ['hps_demo0_sparse_1000.model', 'hps_demo1_sparse_1000.model'],
deployed_devices = [0],
use_gpu_embedding_cache = True,
cache_size_percentage = 0.5,
i64_input_key = True)
],
volatile_db = VolatileDatabaseParams(
DatabaseType_t.redis_cluster,
address = '127.0.0.1:7000',
num_partitions = 15,
num_node_connections = 5,
refresh_time_after_fetch = True,
))
# 2. Initialize the HPS object.
hps = HPS(ps_config)
print('HPS initialized')
# 3. Load query data.
df = pd.read_parquet('data_parquet/val/gen_0.parquet')
dense_input_columns = df.columns[1:11]
cat_input1_columns = df.columns[11:13]
cat_input2_columns = df.columns[13:15]
dense_input = df[dense_input_columns].loc[0:batch_size-1].to_numpy(dtype=np.float32)
cat_input1 = (df[cat_input1_columns].loc[0:batch_size-1].to_numpy(dtype=np.int64) + key_offset[0:2]).reshape((batch_size, 2, 1))
cat_input2 = (df[cat_input2_columns].loc[0:batch_size-1].to_numpy(dtype=np.int64) + key_offset[2:4]).reshape((batch_size, 2, 1))
# 4. Make inference from the HPS object and the ONNX inference session of `hps_demo_without_embedding.onnx`.
embedding1 = hps.lookup(cat_input1.flatten(), 'hps_demo', 0).reshape(batch_size, 2, 16)
embedding2 = hps.lookup(cat_input2.flatten(), 'hps_demo', 1).reshape(batch_size, 2, 32)
sess = ort.InferenceSession('hps_demo_without_embedding.onnx')
res = sess.run(output_names=[sess.get_outputs()[0].name],
input_feed={sess.get_inputs()[0].name: dense_input,
sess.get_inputs()[1].name: embedding1,
sess.get_inputs()[2].name: embedding2})
pred = res[0].flatten()
# 5. Check the correctness by comparing with dumped evaluation results.
ground_truth = np.loadtxt('hps_demo_pred_1000')
print('-------------------------------------------------------------------------------')
print(' HPS demo without embedding ')
print('-------------------------------------------------------------------------------')
print(f'Ground truth: {ground_truth.shape} = {ground_truth}')
print('-------------------------------------------------------------------------------')
print(f'Prediction without embedding: {pred.shape} = {pred}')
diff = pred - ground_truth
mse = np.mean(diff * diff)
print(f'MSE between prediction and ground_truth: {mse}')
# 6. Make inference with the ONNX inference session of `hps_demo_with_embedding.onnx` (double check).
sess_ref = ort.InferenceSession('hps_demo_with_embedding.onnx')
res_ref = sess_ref.run(output_names=[sess_ref.get_outputs()[0].name],
input_feed={sess_ref.get_inputs()[0].name: dense_input,
sess_ref.get_inputs()[1].name: cat_input1,
sess_ref.get_inputs()[2].name: cat_input2})
pred_ref = res_ref[0].flatten()
print('-------------------------------------------------------------------------------')
print(' HPS demo with embedding ')
print('-------------------------------------------------------------------------------')
print(f'Ground truth: {ground_truth.shape} = {ground_truth}')
print('-------------------------------------------------------------------------------')
print(f'Prediction with embedding: {pred_ref.shape} = {pred_ref}')
diff_ref = pred_ref.flatten() - ground_truth
mse_ref = np.mean(diff_ref * diff_ref)
print(f'MSE between prediction and ground_truth: {mse_ref}')
Launching...
HPS initialized[HCTR][12:16:50.287][WARNING][RK0][main]: default_value_for_each_table.size() is not equal to the number of embedding tables
====================================================HPS Create====================================================
[HCTR][12:16:50.289][INFO][RK0][main]: Creating RedisCluster backend...
[HCTR][12:16:50.290][INFO][RK0][main]: RedisCluster: Connecting via 127.0.0.1:7000...
[HCTR][12:16:50.290][INFO][RK0][main]: Volatile DB: initial cache rate = 1
[HCTR][12:16:50.290][INFO][RK0][main]: Volatile DB: cache missed embeddings = 0
[HCTR][12:16:50.290][INFO][RK0][main]: Using Local file system backend.
[HCTR][12:16:50.355][INFO][RK0][main]: Table: hps_et.hps_demo.sparse_embedding1; cached 18424 / 18424 embeddings in volatile database (RedisCluster); load: 18424 / 18446744073709551615 (0.00%).
[HCTR][12:16:50.356][INFO][RK0][main]: Using Local file system backend.
[HCTR][12:16:50.414][INFO][RK0][main]: Table: hps_et.hps_demo.sparse_embedding2; cached 18468 / 18468 embeddings in volatile database (RedisCluster); load: 18468 / 18446744073709551615 (0.00%).
[HCTR][12:16:50.417][INFO][RK0][main]: Creating embedding cache in device 0.
[HCTR][12:16:50.432][INFO][RK0][main]: Model name: hps_demo
[HCTR][12:16:50.432][INFO][RK0][main]: Number of embedding tables: 2
[HCTR][12:16:50.432][INFO][RK0][main]: Use GPU embedding cache: True, cache size percentage: 0.500000
[HCTR][12:16:50.432][INFO][RK0][main]: Use I64 input key: True
[HCTR][12:16:50.432][INFO][RK0][main]: Configured cache hit rate threshold: 1.000000
[HCTR][12:16:50.432][INFO][RK0][main]: The size of thread pool: 256
[HCTR][12:16:50.432][INFO][RK0][main]: The size of worker memory pool: 2
[HCTR][12:16:50.432][INFO][RK0][main]: The size of refresh memory pool: 1
[HCTR][12:16:50.432][INFO][RK0][main]: The refresh percentage : 0.000000
[HCTR][12:16:51.541][INFO][RK0][main]: Creating lookup session for hps_demo on device: 0
-------------------------------------------------------------------------------
HPS demo without embedding
-------------------------------------------------------------------------------
Ground truth: (2048,) = [0.492878 0.491375 0.451757 ... 0.539345 0.503146 0.528778]
-------------------------------------------------------------------------------
Prediction without embedding: (2048,) = [0.48749068 0.4513032 0.5174793 ... 0.5130673 0.50176597 0.56402916]
MSE between prediction and ground_truth: 0.0035816529478249915
-------------------------------------------------------------------------------
HPS demo with embedding
-------------------------------------------------------------------------------
Ground truth: (2048,) = [0.492878 0.491375 0.451757 ... 0.539345 0.503146 0.528778]
-------------------------------------------------------------------------------
Prediction with embedding: (2048,) = [0.48749068 0.4513032 0.5174793 ... 0.5130673 0.50176597 0.56402916]
MSE between prediction and ground_truth: 0.0035816529478249915
2022-12-08 12:16:51.648718677 [W:onnxruntime:, graph.cc:3559 CleanUnusedInitializersAndNodeArgs] Removing initializer 'key_to_indice_hash_all_tables'. It is not used by any node and should be removed from the model.
Step 5: Shutdown Redis cluster
!pkill redis-server
Redis Cluster deployment (with TLS/SSL)
When using Redis as backing storage, HugeCTR can use make use of TLS/SSL to encrypt data transfers. In the following steps we setupt a small Redis cluster and enable SSL for it.
Step 1: Build a TLS/SSL capable distribution of Redis
!wget https://github.com/redis/redis/archive/7.0.5.tar.gz
!tar -xf 7.0.5.tar.gz && rm -f 7.0.5.tar.gz
![ -f redis-7.0.5 ] && rm -rf redis-7.0.5
!cd redis-7.0.5 && make BUILD_TLS=yes
--2022-12-08 12:17:08-- https://github.com/redis/redis/archive/7.0.5.tar.gz
Resolving github.com (github.com)... 192.30.255.112
Connecting to github.com (github.com)|192.30.255.112|:443... connected.
HTTP request sent, awaiting response... 302 Found
Location: https://codeload.github.com/redis/redis/tar.gz/refs/tags/7.0.5 [following]
--2022-12-08 12:17:08-- https://codeload.github.com/redis/redis/tar.gz/refs/tags/7.0.5
Resolving codeload.github.com (codeload.github.com)... 192.30.255.120
Connecting to codeload.github.com (codeload.github.com)|192.30.255.120|:443... connected.
HTTP request sent, awaiting response... 200 OK
Length: 2998759 (2.9M) [application/x-gzip]
Saving to: ‘7.0.5.tar.gz’
7.0.5.tar.gz 100%[===================>] 2.86M 15.2MB/s in 0.2s
2022-12-08 12:17:08 (15.2 MB/s) - ‘7.0.5.tar.gz’ saved [2998759/2998759]
cd src && make all
make[1]: Entering directory '/scratch/proj/hugectr/notebooks/redis-7.0.5/src'
./mkreleasehdr.sh: 1: echo: echo: I/O error
CC Makefile.dep
./mkreleasehdr.sh: 1: echo: echo: I/O error
CC release.o
LINK redis-server
INSTALL redis-sentinel
LINK redis-cli
LINK redis-benchmark
INSTALL redis-check-rdb
INSTALL redis-check-aof
Hint: It's a good idea to run 'make test' ;)
make[1]: Leaving directory '/scratch/proj/hugectr/notebooks/redis-7.0.5/src'
If you see the message Hint: It's a good idea to run 'make test' ;)
followed by make[1]: Leaving directory ...
, the compilation should have completed successfully.
Step 2: Configure a mock Redis cluster
Setup TLS/SSL certificates. Can skip if encyryption is not needed.
WARNING: The following commands will erase the all contents in the following directories: test_certs
, redis-server-1
, redis-server-2
and redis-server-3
.
!mkdir -p test_certs
!rm -f test_certs/*
with open("test_certs/openssl.conf", "w") as f:
f.write("""[ redis_server ]
keyUsage = digitalSignature, keyEncipherment
[ hugectr_client ]
keyUsage = digitalSignature, keyEncipherment
nsCertType = client""")
# Create private keys for CA, Redis server and HugeCTR client.
!openssl genrsa -out test_certs/ca-private.pem 4096
!openssl genrsa -out test_certs/redis-private.pem 4096
!openssl genrsa -out test_certs/hugectr-private.pem 4096
# Create public keys for CA, Redis server and HugeCTR client.
#!openssl rsa -pubout -in test_certs/ca-private.pem -out test_certs/ca-public.pem
#!openssl rsa -pubout -in test_certs/redis-private.pem -out test_certs/redis-public.pem
#!openssl rsa -pubout -in test_certs/hugectr-private.pem -out test_certs/hugectr-public.pem
# Form dummy CA.
!openssl req -new -nodes -sha256 -x509 -subj '/O=NVIDIA Merlin/CN=Certificate Authority' -days 365 \
-key test_certs/ca-private.pem \
-out test_certs/ca.crt
# Generate certificate for Redis server.
!openssl req -new -sha256 -subj "/O=NVIDIA Merlin/CN=Redis Server" \
-key test_certs/redis-private.pem | \
openssl x509 -req -sha256 \
-CA test_certs/ca.crt \
-CAkey test_certs/ca-private.pem \
-CAserial test_certs/redis.ser \
-CAcreateserial \
-days 365 \
-extfile test_certs/openssl.conf -extensions redis_server \
-out test_certs/redis.crt
# Generate certificate for HugeCTR client.
!openssl req -new -sha256 -subj "/O=NVIDIA Merlin/CN=HugeCTR Redis Client" \
-key test_certs/hugectr-private.pem | \
openssl x509 \
-req -sha256 \
-CA test_certs/ca.crt \
-CAkey test_certs/ca-private.pem \
-CAserial test_certs/hugectr.ser \
-CAcreateserial \
-days 365 \
-extfile test_certs/openssl.conf -extensions hugectr_client \
-out test_certs/hugectr.crt
Generating RSA private key, 4096 bit long modulus (2 primes)
.....................................................................................................................................................................................................................................................................................................................++++
........................................................................................................................................................................................................................................................................................................................................................................................++++
e is 65537 (0x010001)
Generating RSA private key, 4096 bit long modulus (2 primes)
.................++++
...++++
e is 65537 (0x010001)
Generating RSA private key, 4096 bit long modulus (2 primes)
..............++++
...................................................++++
e is 65537 (0x010001)
Signature ok
subject=O = NVIDIA Merlin, CN = Redis Server
Getting CA Private Key
Signature ok
subject=O = NVIDIA Merlin, CN = HugeCTR Redis Client
Getting CA Private Key
!mkdir -p redis-server-1 redis-server-2 redis-server-3
!rm -f redis-server-1/* redis-server-2/* redis-server-3/*
!ln -sf $PWD/redis-7.0.5/src/redis-server redis-server-1/redis-server
!ln -sf $PWD/redis-7.0.5/src/redis-server redis-server-2/redis-server
!ln -sf $PWD/redis-7.0.5/src/redis-server redis-server-3/redis-server
!ln -sf $PWD/test_certs/ca.crt redis-server-1/ca.crt
!ln -sf $PWD/test_certs/ca.crt redis-server-2/ca.crt
!ln -sf $PWD/test_certs/ca.crt redis-server-3/ca.crt
!ln -sf $PWD/test_certs/redis-private.pem redis-server-1/private.pem
!ln -sf $PWD/test_certs/redis-private.pem redis-server-2/private.pem
!ln -sf $PWD/test_certs/redis-private.pem redis-server-3/private.pem
!ln -sf $PWD/test_certs/redis.crt redis-server-1/redis.crt
!ln -sf $PWD/test_certs/redis.crt redis-server-2/redis.crt
!ln -sf $PWD/test_certs/redis.crt redis-server-3/redis.crt
%%writefile redis-server-1/redis.conf
daemonize yes
port 0
cluster-enabled yes
cluster-config-file nodes.conf
tls-port 7000
tls-ca-cert-file ca.crt
tls-cert-file redis.crt
tls-key-file private.pem
tls-cluster yes
appendonly no
save ""
Writing redis-server-1/redis.conf
%%writefile redis-server-2/redis.conf
daemonize yes
port 0
cluster-enabled yes
cluster-config-file nodes.conf
tls-port 7001
tls-ca-cert-file ca.crt
tls-cert-file redis.crt
tls-key-file private.pem
tls-cluster yes
appendonly no
save ""
Writing redis-server-2/redis.conf
%%writefile redis-server-3/redis.conf
daemonize yes
port 0
cluster-enabled yes
cluster-config-file nodes.conf
tls-port 7002
tls-ca-cert-file ca.crt
tls-cert-file redis.crt
tls-key-file private.pem
tls-cluster yes
appendonly no
save ""
Writing redis-server-3/redis.conf
Step 3: Form Redis cluster
WARNING: The following command will shutdown any processes called redis-cluster
in the current system!
# Shutdown existing cluster (if any).
!pkill redis-server
# Reset configuration and start 3 Redis servers.
!cd redis-server-1 && rm -f nodes.conf && ./redis-server redis.conf
!cd redis-server-2 && rm -f nodes.conf && ./redis-server redis.conf
!cd redis-server-3 && rm -f nodes.conf && ./redis-server redis.conf
# Form the cluster.
!redis-7.0.5/src/redis-cli \
--cluster create 127.0.0.1:7000 127.0.0.1:7001 127.0.0.1:7002 \
--cluster-yes \
--tls \
--cacert test_certs/ca.crt \
--cert test_certs/hugectr.crt \
--key test_certs/hugectr-private.pem
>>> Performing hash slots allocation on 3 nodes...
Master[0] -> Slots 0 - 5460
Master[1] -> Slots 5461 - 10922
Master[2] -> Slots 10923 - 16383
M: 4bc8ff66e5946deda948e627add42d04fe2e775d 127.0.0.1:7000
slots:[0-5460] (5461 slots) master
M: 9c84f3f2603d38982a16e6fea17d7a2f31299e89 127.0.0.1:7001
slots:[5461-10922] (5462 slots) master
M: c9b58fc19d25ae1ebaa0705a0f69ba40ddf7497d 127.0.0.1:7002
slots:[10923-16383] (5461 slots) master
>>> Nodes configuration updated
>>> Assign a different config epoch to each node
>>> Sending CLUSTER MEET messages to join the cluster
Waiting for the cluster to join
...
>>> Performing Cluster Check (using node 127.0.0.1:7000)
M: 4bc8ff66e5946deda948e627add42d04fe2e775d 127.0.0.1:7000
slots:[0-5460] (5461 slots) master
M: 9c84f3f2603d38982a16e6fea17d7a2f31299e89 127.0.0.1:7001
slots:[5461-10922] (5462 slots) master
M: c9b58fc19d25ae1ebaa0705a0f69ba40ddf7497d 127.0.0.1:7002
slots:[10923-16383] (5461 slots) master
[OK] All nodes agree about slots configuration.
>>> Check for open slots...
>>> Check slots coverage...
[OK] All 16384 slots covered.
Step 4: Run HugeCTR
import os
import time
import multiprocessing as mp
import pandas as pd
import numpy as np
import onnxruntime as ort
from hugectr import DatabaseType_t
from hugectr.inference import HPS, ParameterServerConfig, InferenceParams, VolatileDatabaseParams
slot_size_array = [10000, 10000, 10000, 10000]
key_offset = np.insert(np.cumsum(slot_size_array), 0, 0)[:-1]
batch_size = 2048
print('Launching...')
# 1. Configure the HPS hyperparameters.
ps_config = ParameterServerConfig(
emb_table_name = {'hps_demo': ['sparse_embedding1', 'sparse_embedding2']},
embedding_vec_size = {'hps_demo': [16, 32]},
max_feature_num_per_sample_per_emb_table = {'hps_demo': [2, 2]},
inference_params_array = [
InferenceParams(
model_name = 'hps_demo',
max_batchsize = batch_size,
hit_rate_threshold = 1.0,
dense_model_file = '',
sparse_model_files = ['hps_demo0_sparse_1000.model', 'hps_demo1_sparse_1000.model'],
deployed_devices = [0],
use_gpu_embedding_cache = True,
cache_size_percentage = 0.5,
i64_input_key = True)
],
volatile_db = VolatileDatabaseParams(
DatabaseType_t.redis_cluster,
address = '127.0.0.1:7000',
num_partitions = 15,
num_node_connections = 5,
enable_tls = True,
tls_ca_certificate = 'test_certs/ca.crt',
tls_client_certificate = 'test_certs/hugectr.crt',
tls_client_key = 'test_certs/hugectr-private.pem',
tls_server_name_identification = 'redis.localhost',
refresh_time_after_fetch = True,
))
# 2. Initialize the HPS object.
hps = HPS(ps_config)
print('HPS initialized')
# 3. Load query data.
df = pd.read_parquet('data_parquet/val/gen_0.parquet')
dense_input_columns = df.columns[1:11]
cat_input1_columns = df.columns[11:13]
cat_input2_columns = df.columns[13:15]
dense_input = df[dense_input_columns].loc[0:batch_size-1].to_numpy(dtype=np.float32)
cat_input1 = (df[cat_input1_columns].loc[0:batch_size-1].to_numpy(dtype=np.int64) + key_offset[0:2]).reshape((batch_size, 2, 1))
cat_input2 = (df[cat_input2_columns].loc[0:batch_size-1].to_numpy(dtype=np.int64) + key_offset[2:4]).reshape((batch_size, 2, 1))
# 4. Make inference from the HPS object and the ONNX inference session of `hps_demo_without_embedding.onnx`.
embedding1 = hps.lookup(cat_input1.flatten(), 'hps_demo', 0).reshape(batch_size, 2, 16)
embedding2 = hps.lookup(cat_input2.flatten(), 'hps_demo', 1).reshape(batch_size, 2, 32)
sess = ort.InferenceSession('hps_demo_without_embedding.onnx')
res = sess.run(output_names=[sess.get_outputs()[0].name],
input_feed={sess.get_inputs()[0].name: dense_input,
sess.get_inputs()[1].name: embedding1,
sess.get_inputs()[2].name: embedding2})
pred = res[0].flatten()
# 5. Check the correctness by comparing with dumped evaluation results.
ground_truth = np.loadtxt('hps_demo_pred_1000')
print('-------------------------------------------------------------------------------')
print(' HPS demo without embedding ')
print('-------------------------------------------------------------------------------')
print(f'Ground truth: {ground_truth.shape} = {ground_truth}')
print('-------------------------------------------------------------------------------')
print(f'Prediction without embedding: {pred.shape} = {pred}')
diff = pred - ground_truth
mse = np.mean(diff * diff)
print(f'MSE between prediction and ground_truth: {mse}')
# 6. Make inference with the ONNX inference session of `hps_demo_with_embedding.onnx` (double check).
sess_ref = ort.InferenceSession('hps_demo_with_embedding.onnx')
res_ref = sess_ref.run(output_names=[sess_ref.get_outputs()[0].name],
input_feed={sess_ref.get_inputs()[0].name: dense_input,
sess_ref.get_inputs()[1].name: cat_input1,
sess_ref.get_inputs()[2].name: cat_input2})
pred_ref = res_ref[0].flatten()
print('-------------------------------------------------------------------------------')
print(' HPS demo with embedding ')
print('-------------------------------------------------------------------------------')
print(f'Ground truth: {ground_truth.shape} = {ground_truth}')
print('-------------------------------------------------------------------------------')
print(f'Prediction with embedding: {pred_ref.shape} = {pred_ref}')
diff_ref = pred_ref.flatten() - ground_truth
mse_ref = np.mean(diff_ref * diff_ref)
print(f'MSE between prediction and ground_truth: {mse_ref}')
Launching...
[HCTR][12:18:30.809][WARNING][RK0][main]: default_value_for_each_table.size() is not equal to the number of embedding tables
====================================================HPS Create====================================================
[HCTR][12:18:30.810][INFO][RK0][main]: Creating RedisCluster backend...
[HCTR][12:18:30.811][INFO][RK0][main]: RedisCluster: Connecting via 127.0.0.1:7000...
[HCTR][12:18:30.839][INFO][RK0][main]: Volatile DB: initial cache rate = 1
[HCTR][12:18:30.839][INFO][RK0][main]: Volatile DB: cache missed embeddings = 0
[HCTR][12:18:30.839][INFO][RK0][main]: Using Local file system backend.
[HCTR][12:18:30.980][INFO][RK0][main]: Table: hps_et.hps_demo.sparse_embedding1; cached 18424 / 18424 embeddings in volatile database (RedisCluster); load: 18424 / 18446744073709551615 (0.00%).
[HCTR][12:18:30.981][INFO][RK0][main]: Using Local file system backend.
[HCTR][12:18:31.051][INFO][RK0][main]: Table: hps_et.hps_demo.sparse_embedding2; cached 18468 / 18468 embeddings in volatile database (RedisCluster); load: 18468 / 18446744073709551615 (0.00%).
[HCTR][12:18:31.053][INFO][RK0][main]: Creating embedding cache in device 0.
[HCTR][12:18:31.069][INFO][RK0][main]: Model name: hps_demo
[HCTR][12:18:31.069][INFO][RK0][main]: Number of embedding tables: 2
[HCTR][12:18:31.069][INFO][RK0][main]: Use GPU embedding cache: True, cache size percentage: 0.500000
[HCTR][12:18:31.069][INFO][RK0][main]: Use I64 input key: True
[HCTR][12:18:31.069][INFO][RK0][main]: Configured cache hit rate threshold: 1.000000
[HCTR][12:18:31.069][INFO][RK0][main]: The size of thread pool: 256
[HCTR][12:18:31.069][INFO][RK0][main]: The size of worker memory pool: 2
[HCTR][12:18:31.069][INFO][RK0][main]: The size of refresh memory pool: 1
[HCTR][12:18:31.069][INFO][RK0][main]: The refresh percentage : 0.000000
[HCTR][12:18:31.072][INFO][RK0][main]: Creating lookup session for hps_demo on device: 0
[HCTR][12:18:31.078][INFO][RK0][main]: RedisCluster: Awaiting background worker to conclude...
[HCTR][12:18:31.078][INFO][RK0][main]: RedisCluster: Disconnecting...
HPS initialized
-------------------------------------------------------------------------------
HPS demo without embedding
-------------------------------------------------------------------------------
Ground truth: (2048,) = [0.492878 0.491375 0.451757 ... 0.539345 0.503146 0.528778]
-------------------------------------------------------------------------------
Prediction without embedding: (2048,) = [0.48749068 0.4513032 0.5174793 ... 0.5130673 0.50176597 0.56402916]
MSE between prediction and ground_truth: 0.0035816529478249915
-------------------------------------------------------------------------------
HPS demo with embedding
-------------------------------------------------------------------------------
Ground truth: (2048,) = [0.492878 0.491375 0.451757 ... 0.539345 0.503146 0.528778]
-------------------------------------------------------------------------------
Prediction with embedding: (2048,) = [0.48749068 0.4513032 0.5174793 ... 0.5130673 0.50176597 0.56402916]
MSE between prediction and ground_truth: 0.0035816529478249915
2022-12-08 12:18:31.115939884 [W:onnxruntime:, graph.cc:3559 CleanUnusedInitializersAndNodeArgs] Removing initializer 'key_to_indice_hash_all_tables'. It is not used by any node and should be removed from the model.
Step 5: Shutdown Redis cluster
!pkill redis-server