# Copyright 2021 NVIDIA Corporation. All Rights Reserved.
#
# Licensed under the Apache License, Version 2.0 (the "License");
# you may not use this file except in compliance with the License.
# You may obtain a copy of the License at
#
# http://www.apache.org/licenses/LICENSE-2.0
#
# Unless required by applicable law or agreed to in writing, software
# distributed under the License is distributed on an "AS IS" BASIS,
# WITHOUT WARRANTIES OR CONDITIONS OF ANY KIND, either express or implied.
# See the License for the specific language governing permissions and
# limitations under the License.
# ==============================================================================
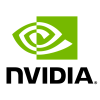
Scaling Criteo: Training with Merlin Models TensorFlow#
This notebook is created using the latest stable merlin-tensorflow container.
Overview#
The Criteo 1TB Click Logs dataset is a popular dataset in the recommender system community as it is one of the largest, public available dataset. It contains ~1.3 TB of uncompressed click logs containing over four billion samples spanning 24 days.
We will train Facebook’s deep learning recommendation model (DLRM) architecture with Merlin Models. We will assume you are familiar with Merlin Models’ API and features. Otherwise, we recommend to start with the Merlin Models examples.
Learning objectives#
Train a DLRM architecture with Merlin Models on a large dataset
Training a DLRM model#
Let’s start with importing the libraries that we’ll use in this notebook.
import os
os.environ["TF_GPU_ALLOCATOR"] = "cuda_malloc_async"
import glob
import merlin.models.tf as mm
from merlin.io.dataset import Dataset
from merlin.schema import Tags
import tensorflow as tf
Define the path to directories which contains the processed data.
input_path = os.environ.get("INPUT_DATA_DIR", "/raid/data/criteo/test_dask/output/")
# path to processed data
PATH_TO_TRAIN_DATA = sorted(glob.glob(os.path.join(input_path, "train", "*.parquet")))
PATH_TO_VALID_DATA = sorted(glob.glob(os.path.join(input_path, "valid", "*.parquet")))
PATH_TO_TRAIN_DATA, PATH_TO_VALID_DATA
We define some hyperparameters for the model architecture.
BATCH_SIZE = int(os.environ.get("BATCH_SIZE", 64 * 1024))
EMBEDDING_SIZE = 8
EPOCHS = 1
LR = 0.01
OPTIMIZER = tf.keras.optimizers.SGD(learning_rate=LR)
We will use Merlin Dataset object to initialize the dataloaders. It provides a dataset schema to initialize the model architectures. The Merlin Models examples will explain more details.
train = Dataset(PATH_TO_TRAIN_DATA, part_mem_fraction=0.08)
valid = Dataset(PATH_TO_VALID_DATA, part_mem_fraction=0.08)
We initialize the DLRM architecture with Merlin Models.
model = mm.DLRMModel(
train.schema,
embedding_dim=EMBEDDING_SIZE,
bottom_block=mm.MLPBlock([128, EMBEDDING_SIZE]),
top_block=mm.MLPBlock([128, 64, 32]),
prediction_tasks=mm.BinaryClassificationTask(
train.schema.select_by_tag(Tags.TARGET).column_names[0]
)
)
We compile and train our model.
%%time
model.compile(optimizer=OPTIMIZER, run_eagerly=False)
model.fit(train,
validation_data=valid,
batch_size=BATCH_SIZE,
epochs=EPOCHS
)
Evaluate the model#
Finally, we can evaluate our model on the validation dataset.
eval_metrics = model.evaluate(valid, batch_size=BATCH_SIZE, return_dict=True)
eval_metrics
Save the model#
We save the model to disk.
model.save(os.path.join(input_path, "dlrm"))
Summary#
We trained Facebook’s popular DLRM architecture with only ~5 commands on the large criteo dataset.
Next steps#
The next step is to deploy the NVTabular workflow and DLRM model to production.
If you are interested more in different architecture and training models with Merlin Models, we recommend to check out our Merlin Models examples