# Copyright 2022 NVIDIA Corporation. All Rights Reserved.
#
# Licensed under the Apache License, Version 2.0 (the "License");
# you may not use this file except in compliance with the License.
# You may obtain a copy of the License at
#
# http://www.apache.org/licenses/LICENSE-2.0
#
# Unless required by applicable law or agreed to in writing, software
# distributed under the License is distributed on an "AS IS" BASIS,
# WITHOUT WARRANTIES OR CONDITIONS OF ANY KIND, either express or implied.
# See the License for the specific language governing permissions and
# limitations under the License.
# ==============================================================================
# Each user is responsible for checking the content of datasets and the
# applicable licenses and determining if suitable for the intended use.
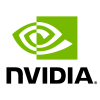
Session-based Recommendation with XLNET
This notebook is created using the latest stable merlin-pytorch container.
In this notebook we introduce the Transformers4Rec library for sequential and session-based recommendation. This notebook uses the PyTorch API. Transformers4Rec integrates with the popular HuggingFace’s Transformers and makes it possible to experiment with a cutting-edge implementation of the latest NLP Transformer architectures.
We demonstrate how to build a session-based recommendation model with the XLNET Transformer architecture. The XLNet architecture was designed to leverage the best of both auto-regressive language modeling and auto-encoding with its Permutation Language Modeling training method. In this example we will use XLNET with masked language modeling (MLM) training method, which showed very promising results in the experiments conducted in our ACM RecSys’21 paper.
In the previous notebook we went through our ETL pipeline with the NVTabular library, and created sequential features to be used in training a session-based recommendation model. In this notebook we will learn:
Accelerating data loading of parquet files with multiple features on PyTorch using NVTabular library
Training and evaluating a Transformer-based (XLNET-MLM) session-based recommendation model with multiple features
Build a DL model with Transformers4Rec library
Transformers4Rec supports multiple input features and provides configurable building blocks that can be easily combined for custom architectures:
TabularSequenceFeatures class that reads from schema and creates an input block. This input module combines different types of features (continuous, categorical & text) to a sequence.
MaskSequence to define masking schema and prepare the masked inputs and labels for the selected LM task.
TransformerBlock class that supports HuggingFace Transformers for session-based and sequential-based recommendation models.
SequentialBlock creates the body by mimicking torch.nn.sequential class. It is designed to define our model as a sequence of layers.
Head where we define the prediction task of the model.
NextItemPredictionTask is the class to support next item prediction task.
Trainer extends the
Trainer
class from HF transformers and manages the model training and evaluation.
You can check the full documentation of Transformers4Rec if needed.
Figure 1 illustrates Transformers4Rec meta-architecture and how each module/block interacts with each other.
Import required libraries
import os
os.environ["CUDA_VISIBLE_DEVICES"]="0"
import glob
import torch
from transformers4rec import torch as tr
from transformers4rec.torch.ranking_metric import NDCGAt, AvgPrecisionAt, RecallAt
from transformers4rec.torch.utils.examples_utils import wipe_memory
/usr/local/lib/python3.8/dist-packages/tqdm/auto.py:22: TqdmWarning: IProgress not found. Please update jupyter and ipywidgets. See https://ipywidgets.readthedocs.io/en/stable/user_install.html
from .autonotebook import tqdm as notebook_tqdm
/usr/lib/python3/dist-packages/requests/__init__.py:89: RequestsDependencyWarning: urllib3 (1.26.12) or chardet (3.0.4) doesn't match a supported version!
warnings.warn("urllib3 ({}) or chardet ({}) doesn't match a supported "
/usr/local/lib/python3.8/dist-packages/torchmetrics/utilities/prints.py:36: UserWarning: Torchmetrics v0.9 introduced a new argument class property called `full_state_update` that has
not been set for this class (NDCGAt). The property determines if `update` by
default needs access to the full metric state. If this is not the case, significant speedups can be
achieved and we recommend setting this to `False`.
We provide an checking function
`from torchmetrics.utilities import check_forward_full_state_property`
that can be used to check if the `full_state_update=True` (old and potential slower behaviour,
default for now) or if `full_state_update=False` can be used safely.
warnings.warn(*args, **kwargs)
/usr/local/lib/python3.8/dist-packages/torchmetrics/utilities/prints.py:36: UserWarning: Torchmetrics v0.9 introduced a new argument class property called `full_state_update` that has
not been set for this class (DCGAt). The property determines if `update` by
default needs access to the full metric state. If this is not the case, significant speedups can be
achieved and we recommend setting this to `False`.
We provide an checking function
`from torchmetrics.utilities import check_forward_full_state_property`
that can be used to check if the `full_state_update=True` (old and potential slower behaviour,
default for now) or if `full_state_update=False` can be used safely.
warnings.warn(*args, **kwargs)
/usr/local/lib/python3.8/dist-packages/torchmetrics/utilities/prints.py:36: UserWarning: Torchmetrics v0.9 introduced a new argument class property called `full_state_update` that has
not been set for this class (AvgPrecisionAt). The property determines if `update` by
default needs access to the full metric state. If this is not the case, significant speedups can be
achieved and we recommend setting this to `False`.
We provide an checking function
`from torchmetrics.utilities import check_forward_full_state_property`
that can be used to check if the `full_state_update=True` (old and potential slower behaviour,
default for now) or if `full_state_update=False` can be used safely.
warnings.warn(*args, **kwargs)
/usr/local/lib/python3.8/dist-packages/torchmetrics/utilities/prints.py:36: UserWarning: Torchmetrics v0.9 introduced a new argument class property called `full_state_update` that has
not been set for this class (PrecisionAt). The property determines if `update` by
default needs access to the full metric state. If this is not the case, significant speedups can be
achieved and we recommend setting this to `False`.
We provide an checking function
`from torchmetrics.utilities import check_forward_full_state_property`
that can be used to check if the `full_state_update=True` (old and potential slower behaviour,
default for now) or if `full_state_update=False` can be used safely.
warnings.warn(*args, **kwargs)
/usr/local/lib/python3.8/dist-packages/torchmetrics/utilities/prints.py:36: UserWarning: Torchmetrics v0.9 introduced a new argument class property called `full_state_update` that has
not been set for this class (RecallAt). The property determines if `update` by
default needs access to the full metric state. If this is not the case, significant speedups can be
achieved and we recommend setting this to `False`.
We provide an checking function
`from torchmetrics.utilities import check_forward_full_state_property`
that can be used to check if the `full_state_update=True` (old and potential slower behaviour,
default for now) or if `full_state_update=False` can be used safely.
warnings.warn(*args, **kwargs)
Transformers4Rec library relies on a schema object to automatically build all necessary layers to represent, normalize and aggregate input features. As you can see below, schema.pb
is a protobuf file that contains metadata including statistics about features such as cardinality, min and max values and also tags features based on their characteristics and dtypes (e.g., categorical, continuous, list, integer).
Set the schema object
We create the schema object by reading the schema.pbtxt
file generated by NVTabular pipeline in the previous, 01-ETL-with-NVTabular
, notebook.
from merlin_standard_lib import Schema
SCHEMA_PATH = os.environ.get("INPUT_SCHEMA_PATH", "/workspace/data/processed_nvt/schema.pbtxt")
schema = Schema().from_proto_text(SCHEMA_PATH)
!head -20 $SCHEMA_PATH
feature {
name: "session_id"
type: INT
int_domain {
name: "session_id"
max: 19863
is_categorical: true
}
annotation {
tag: "categorical"
extra_metadata {
type_url: "type.googleapis.com/google.protobuf.Struct"
value: "\n\021\n\013num_buckets\022\002\010\000\n\033\n\016freq_threshold\022\t\021\000\000\000\000\000\000\000\000\n\025\n\010max_size\022\t\021\000\000\000\000\000\000\000\000\n\030\n\013start_index\022\t\021\000\000\000\000\000\000\000\000\n5\n\010cat_path\022)\032\'.//categories/unique.session_id.parquet\nG\n\017embedding_sizes\0224*2\n\030\n\013cardinality\022\t\021\000\000\000\000\000f\323@\n\026\n\tdimension\022\t\021\000\000\000\000\000\200y@\n\034\n\017dtype_item_size\022\t\021\000\000\000\000\000\000P@\n\r\n\007is_list\022\002 \000\n\017\n\tis_ragged\022\002 \000"
}
}
}
feature {
name: "day-first"
type: INT
annotation {
# You can select a subset of features for training
schema = schema.select_by_name(['item_id-list',
'category-list',
'weekday_sin-list',
'age_days-list'])
Define the sequential input module
Below we define our input
block using the TabularSequenceFeatures
class. The from_schema()
method processes the schema and creates the necessary layers to represent features and aggregate them. It keeps only features tagged as categorical
and continuous
and supports data aggregation methods like concat
and elementwise-sum
. It also supports data augmentation techniques like stochastic swap noise. It outputs an interaction representation after combining all features and also the input mask according to the training task (more on this later).
The max_sequence_length
argument defines the maximum sequence length of our sequential input, and if continuous_projection
argument is set, all numerical features are concatenated and projected by an MLP block so that continuous features are represented by a vector of size defined by user, which is 64
in this example.
inputs = tr.TabularSequenceFeatures.from_schema(
schema,
max_sequence_length=20,
continuous_projection=64,
masking="mlm",
d_output=100,
)
The output of the TabularSequenceFeatures
module is the sequence of interactions embedding vectors defined in the following steps:
Create sequence inputs: If the schema contains non sequential features, expand each feature to a sequence by repeating the value as many times as the
max_sequence_length
value.
Get a representation vector of categorical features: Project each sequential categorical feature using the related embedding table. The resulting tensor is of shape (bs, max_sequence_length, embed_dim).
Project scalar values if
continuous_projection
is set : Apply an MLP layer with hidden size equal tocontinuous_projection
vector size value. The resulting tensor is of shape (batch_size, max_sequence_length, continuous_projection).
Aggregate the list of features vectors to represent each interaction in the sequence with one vector: For example,
concat
will concat all vectors based on the last dimension-1
and the resulting tensor will be of shape (batch_size, max_sequence_length, D) where D is the sum over all embedding dimensions and the value of continuous_projection.
If masking schema is set (needed only for the
NextItemPredictionTask
training), the masked labels are derived from the sequence of raw item-ids and the sequence of interactions embeddings are processed to mask information about the masked positions.
Define the Transformer block
In the next cell, the whole model is build with a few lines of code. Here is a brief explanation of the main classes:
XLNetConfig - We have injected in the HF transformers config classes like
XLNetConfig
thebuild()
method that provides default configuration to Transformer architectures for session-based recommendation. Here we use it to instantiate and configure an XLNET architecture.TransformerBlock class integrates with HF Transformers, which are made available as a sequence processing module for session-based and sequential-based recommendation models.
NextItemPredictionTask supports the next-item prediction task. We also support other predictions tasks, like classification and regression for the whole sequence.
# Define XLNetConfig class and set default parameters for HF XLNet config
transformer_config = tr.XLNetConfig.build(
d_model=64, n_head=4, n_layer=2, total_seq_length=20
)
# Define the model block including: inputs, masking, projection and transformer block.
body = tr.SequentialBlock(
inputs, tr.MLPBlock([64]), tr.TransformerBlock(transformer_config, masking=inputs.masking)
)
# Define the evaluation top-N metrics and the cut-offs
metrics = [NDCGAt(top_ks=[20, 40], labels_onehot=True),
RecallAt(top_ks=[20, 40], labels_onehot=True)]
# Define a head related to next item prediction task
head = tr.Head(
body,
tr.NextItemPredictionTask(weight_tying=True,
metrics=metrics),
inputs=inputs,
)
# Get the end-to-end Model class
model = tr.Model(head)
/usr/local/lib/python3.8/dist-packages/torchmetrics/utilities/prints.py:36: UserWarning: Torchmetrics v0.9 introduced a new argument class property called `full_state_update` that has
not been set for this class (NDCGAt). The property determines if `update` by
default needs access to the full metric state. If this is not the case, significant speedups can be
achieved and we recommend setting this to `False`.
We provide an checking function
`from torchmetrics.utilities import check_forward_full_state_property`
that can be used to check if the `full_state_update=True` (old and potential slower behaviour,
default for now) or if `full_state_update=False` can be used safely.
warnings.warn(*args, **kwargs)
/usr/local/lib/python3.8/dist-packages/torchmetrics/utilities/prints.py:36: UserWarning: Torchmetrics v0.9 introduced a new argument class property called `full_state_update` that has
not been set for this class (DCGAt). The property determines if `update` by
default needs access to the full metric state. If this is not the case, significant speedups can be
achieved and we recommend setting this to `False`.
We provide an checking function
`from torchmetrics.utilities import check_forward_full_state_property`
that can be used to check if the `full_state_update=True` (old and potential slower behaviour,
default for now) or if `full_state_update=False` can be used safely.
warnings.warn(*args, **kwargs)
/usr/local/lib/python3.8/dist-packages/torchmetrics/utilities/prints.py:36: UserWarning: Torchmetrics v0.9 introduced a new argument class property called `full_state_update` that has
not been set for this class (RecallAt). The property determines if `update` by
default needs access to the full metric state. If this is not the case, significant speedups can be
achieved and we recommend setting this to `False`.
We provide an checking function
`from torchmetrics.utilities import check_forward_full_state_property`
that can be used to check if the `full_state_update=True` (old and potential slower behaviour,
default for now) or if `full_state_update=False` can be used safely.
warnings.warn(*args, **kwargs)
Note that we can easily define an RNN-based model inside the SequentialBlock
instead of a Transformer-based model. You can explore this tutorial for a GRU-based model example.
Train the model
We use the Merlin Dataloader’s PyTorch Dataloader for optimized loading of multiple features from input parquet files. You can learn more about this data loader here.
Set Training arguments
per_device_train_batch_size = int(os.environ.get(
"per_device_train_batch_size",
'128'
))
per_device_eval_batch_size = int(os.environ.get(
"per_device_eval_batch_size",
'32'
))
from transformers4rec.config.trainer import T4RecTrainingArguments
from transformers4rec.torch import Trainer
# Set hyperparameters for training
train_args = T4RecTrainingArguments(data_loader_engine='merlin',
dataloader_drop_last = True,
gradient_accumulation_steps = 1,
per_device_train_batch_size = per_device_train_batch_size,
per_device_eval_batch_size = per_device_eval_batch_size,
output_dir = "./tmp",
learning_rate=0.0005,
lr_scheduler_type='cosine',
learning_rate_num_cosine_cycles_by_epoch=1.5,
num_train_epochs=5,
max_sequence_length=20,
report_to = [],
logging_steps=50,
no_cuda=False)
Note that we add an argument data_loader_engine='merlin'
to automatically load the features needed for training using the schema. The default value is merlin
for optimized GPU-based data-loading. Optionally a PyarrowDataLoader
(pyarrow) can also be used as a basic option, but it is slower and works only for small datasets, as the full data is loaded to CPU memory.
Daily Fine-Tuning: Training over a time window
Here we do daily fine-tuning meaning that we use the first day to train and second day to evaluate, then we use the second day data to train the model by resuming from the first step, and evaluate on the third day, so on and so forth.
We have extended the HuggingFace transformers Trainer
class (PyTorch only) to support evaluation of RecSys metrics. In this example, the evaluation of the session-based recommendation model is performed using traditional Top-N ranking metrics such as Normalized Discounted Cumulative Gain (NDCG@20) and Hit Rate (HR@20). NDCG accounts for rank of the relevant item in the recommendation list and is a more fine-grained metric than HR, which only verifies whether the relevant item is among the top-n items. HR@n is equivalent to Recall@n when there is only one relevant item in the recommendation list.
# Instantiate the T4Rec Trainer, which manages training and evaluation for the PyTorch API
trainer = Trainer(
model=model,
args=train_args,
schema=schema,
compute_metrics=True,
)
Define the output folder of the processed parquet files:
INPUT_DATA_DIR = os.environ.get("INPUT_DATA_DIR", "/workspace/data")
OUTPUT_DIR = os.environ.get("OUTPUT_DIR", f"{INPUT_DATA_DIR}/sessions_by_day")
start_window_index = int(os.environ.get(
"start_window_index",
'1'
))
final_window_index = int(os.environ.get(
"final_window_index",
'8'
))
start_time_window_index = start_window_index
final_time_window_index = final_window_index
#Iterating over days of one week
for time_index in range(start_time_window_index, final_time_window_index):
# Set data
time_index_train = time_index
time_index_eval = time_index + 1
train_paths = glob.glob(os.path.join(OUTPUT_DIR, f"{time_index_train}/train.parquet"))
eval_paths = glob.glob(os.path.join(OUTPUT_DIR, f"{time_index_eval}/valid.parquet"))
print(train_paths)
# Train on day related to time_index
print('*'*20)
print("Launch training for day %s are:" %time_index)
print('*'*20 + '\n')
trainer.train_dataset_or_path = train_paths
trainer.reset_lr_scheduler()
trainer.train()
trainer.state.global_step +=1
print('finished')
# Evaluate on the following day
trainer.eval_dataset_or_path = eval_paths
train_metrics = trainer.evaluate(metric_key_prefix='eval')
print('*'*20)
print("Eval results for day %s are:\t" %time_index_eval)
print('\n' + '*'*20 + '\n')
for key in sorted(train_metrics.keys()):
print(" %s = %s" % (key, str(train_metrics[key])))
wipe_memory()
***** Running training *****
Num examples = 1664
Num Epochs = 5
Instantaneous batch size per device = 128
Total train batch size (w. parallel, distributed & accumulation) = 128
Gradient Accumulation steps = 1
Total optimization steps = 65
['/workspace/data/sessions_by_day/1/train.parquet']
********************
Launch training for day 1 are:
********************
Step | Training Loss |
---|---|
50 | 5.826500 |
Training completed. Do not forget to share your model on huggingface.co/models =)
finished
********************
Eval results for day 2 are:
********************
eval_/loss = 5.089233875274658
eval_/next-item/ndcg_at_20 = 0.1664465367794037
eval_/next-item/ndcg_at_40 = 0.2163003385066986
eval_/next-item/recall_at_20 = 0.4583333432674408
eval_/next-item/recall_at_40 = 0.703125
eval_runtime = 0.1437
eval_samples_per_second = 1335.721
eval_steps_per_second = 41.741
***** Running training *****
Num examples = 1536
Num Epochs = 5
Instantaneous batch size per device = 128
Total train batch size (w. parallel, distributed & accumulation) = 128
Gradient Accumulation steps = 1
Total optimization steps = 60
['/workspace/data/sessions_by_day/2/train.parquet']
********************
Launch training for day 2 are:
********************
Step | Training Loss |
---|---|
50 | 4.900800 |
Training completed. Do not forget to share your model on huggingface.co/models =)
finished
********************
Eval results for day 3 are:
********************
eval_/loss = 4.635087966918945
eval_/next-item/ndcg_at_20 = 0.19952264428138733
eval_/next-item/ndcg_at_40 = 0.24891459941864014
eval_/next-item/recall_at_20 = 0.5052083730697632
eval_/next-item/recall_at_40 = 0.7447916865348816
eval_runtime = 0.1381
eval_samples_per_second = 1389.895
eval_steps_per_second = 43.434
['/workspace/data/sessions_by_day/3/train.parquet']
********************
Launch training for day 3 are:
********************
***** Running training *****
Num examples = 1664
Num Epochs = 5
Instantaneous batch size per device = 128
Total train batch size (w. parallel, distributed & accumulation) = 128
Gradient Accumulation steps = 1
Total optimization steps = 65
Step | Training Loss |
---|---|
50 | 4.616300 |
Training completed. Do not forget to share your model on huggingface.co/models =)
finished
********************
Eval results for day 4 are:
********************
eval_/loss = 4.498825550079346
eval_/next-item/ndcg_at_20 = 0.1833435297012329
eval_/next-item/ndcg_at_40 = 0.22633466124534607
eval_/next-item/recall_at_20 = 0.53125
eval_/next-item/recall_at_40 = 0.7395833730697632
eval_runtime = 0.1391
eval_samples_per_second = 1380.644
eval_steps_per_second = 43.145
['/workspace/data/sessions_by_day/4/train.parquet']
********************
Launch training for day 4 are:
********************
***** Running training *****
Num examples = 1664
Num Epochs = 5
Instantaneous batch size per device = 128
Total train batch size (w. parallel, distributed & accumulation) = 128
Gradient Accumulation steps = 1
Total optimization steps = 65
Step | Training Loss |
---|---|
50 | 4.538300 |
Training completed. Do not forget to share your model on huggingface.co/models =)
finished
********************
Eval results for day 5 are:
********************
eval_/loss = 4.3865251541137695
eval_/next-item/ndcg_at_20 = 0.19835762679576874
eval_/next-item/ndcg_at_40 = 0.2511940002441406
eval_/next-item/recall_at_20 = 0.5364583730697632
eval_/next-item/recall_at_40 = 0.7916666865348816
eval_runtime = 0.1283
eval_samples_per_second = 1496.582
eval_steps_per_second = 46.768
['/workspace/data/sessions_by_day/5/train.parquet']
********************
Launch training for day 5 are:
********************
***** Running training *****
Num examples = 1664
Num Epochs = 5
Instantaneous batch size per device = 128
Total train batch size (w. parallel, distributed & accumulation) = 128
Gradient Accumulation steps = 1
Total optimization steps = 65
Step | Training Loss |
---|---|
50 | 4.499100 |
Training completed. Do not forget to share your model on huggingface.co/models =)
finished
********************
Eval results for day 6 are:
********************
eval_/loss = 4.248472213745117
eval_/next-item/ndcg_at_20 = 0.21316508948802948
eval_/next-item/ndcg_at_40 = 0.2594021260738373
eval_/next-item/recall_at_20 = 0.59375
eval_/next-item/recall_at_40 = 0.8177083730697632
eval_runtime = 0.1378
eval_samples_per_second = 1393.324
eval_steps_per_second = 43.541
['/workspace/data/sessions_by_day/6/train.parquet']
********************
Launch training for day 6 are:
********************
***** Running training *****
Num examples = 1664
Num Epochs = 5
Instantaneous batch size per device = 128
Total train batch size (w. parallel, distributed & accumulation) = 128
Gradient Accumulation steps = 1
Total optimization steps = 65
Step | Training Loss |
---|---|
50 | 4.478100 |
Training completed. Do not forget to share your model on huggingface.co/models =)
finished
********************
Eval results for day 7 are:
********************
eval_/loss = 4.356322765350342
eval_/next-item/ndcg_at_20 = 0.17782533168792725
eval_/next-item/ndcg_at_40 = 0.24227355420589447
eval_/next-item/recall_at_20 = 0.5208333730697632
eval_/next-item/recall_at_40 = 0.8333333730697632
eval_runtime = 0.1394
eval_samples_per_second = 1377.035
eval_steps_per_second = 43.032
['/workspace/data/sessions_by_day/7/train.parquet']
********************
Launch training for day 7 are:
********************
***** Running training *****
Num examples = 1664
Num Epochs = 5
Instantaneous batch size per device = 128
Total train batch size (w. parallel, distributed & accumulation) = 128
Gradient Accumulation steps = 1
Total optimization steps = 65
Step | Training Loss |
---|---|
50 | 4.480100 |
Training completed. Do not forget to share your model on huggingface.co/models =)
finished
********************
Eval results for day 8 are:
********************
eval_/loss = 4.452080249786377
eval_/next-item/ndcg_at_20 = 0.19874617457389832
eval_/next-item/ndcg_at_40 = 0.24815073609352112
eval_/next-item/recall_at_20 = 0.5208333730697632
eval_/next-item/recall_at_40 = 0.7604166865348816
eval_runtime = 0.1314
eval_samples_per_second = 1460.932
eval_steps_per_second = 45.654
Re-compute evaluation metrics of the validation data
eval_data_paths = glob.glob(os.path.join(OUTPUT_DIR, f"{time_index_eval}/valid.parquet"))
# set new data from day 7
eval_metrics = trainer.evaluate(eval_dataset=eval_data_paths, metric_key_prefix='eval')
for key in sorted(eval_metrics.keys()):
print(" %s = %s" % (key, str(eval_metrics[key])))
eval_/loss = 4.452080249786377
eval_/next-item/ndcg_at_20 = 0.19874617457389832
eval_/next-item/ndcg_at_40 = 0.24815073609352112
eval_/next-item/recall_at_20 = 0.5208333730697632
eval_/next-item/recall_at_40 = 0.7604166865348816
eval_runtime = 0.1411
eval_samples_per_second = 1360.439
eval_steps_per_second = 42.514
Save the model
Let’s save the model to be able to load it back at inference step. Using model.save()
, we save the model as a pkl file in the given path.
model_path= os.environ.get("OUTPUT_DIR", f"{INPUT_DATA_DIR}/saved_model")
model.save(model_path)
That’s it! You have just trained your session-based recommendation model using Transformers4Rec. Now you can move on to the next notebook 03-serving-session-based-model-torch-backend
. Please shut down this kernel to free the GPU memory before you start the next one.
Tip: We can easily log and visualize model training and evaluation on Weights & Biases (W&B), TensorBoard, or NVIDIA DLLogger. By default, the HuggingFace transformers Trainer
(which we extend) uses Weights & Biases (W&B) to log training and evaluation metrics, which provides nice visualization results and comparison between different runs.