Features in SparseOperationKit
Model-Parallelism GPU Embedding Layer
SOK provides GPU embedding layers that take advantage of model-parallelism. No further data transformation from model-parallelism to data-parallism is required.
SOK implements several different GPU embedding layers. The layers use different algorithms to provide maximum performance in different application scenarios. SOK supports single machine and multi-machine cluster deployment.
Sparse Embedding Layer
The sparse embedding layer is equivalent to tf.nn.embedding_lookup_sparse
, except the sparse embedding layers in SOK operates in a MP manner. The sparse embedding layer supports the Mean
and Sum
combiners.
Distributed Sparse Embedding
The distributed sparse embedding scatters keys across GPUSs by computing gpu_id = key % number_of_gpus
. For example, if there are 8 GPUs, then key=1000
will be deployed to GPU-0, key=1001
will be deployed to GPU-1. The following picture depicts its forward propagation process.
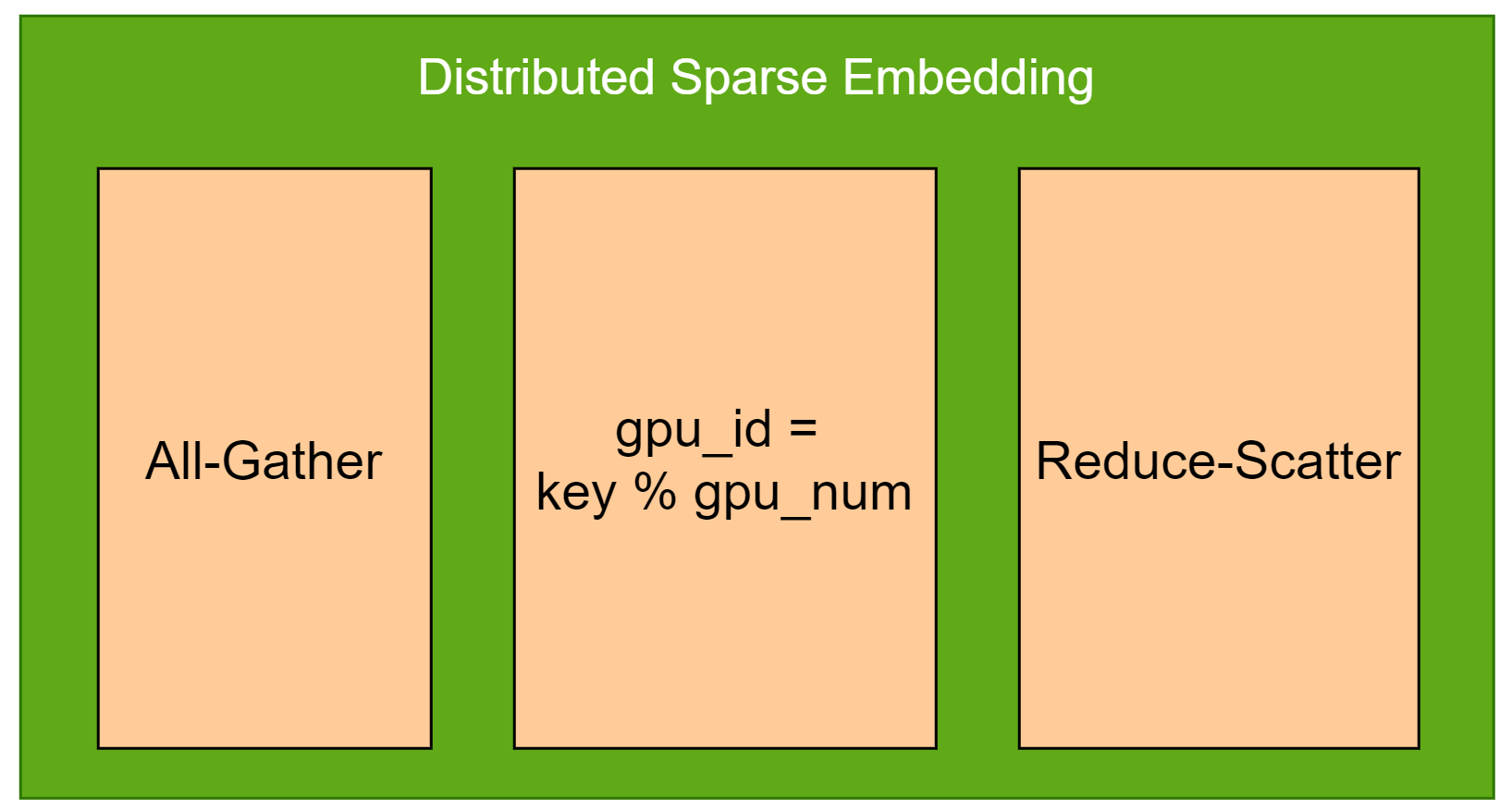
To reduce the overhead when looking up multiple embedding tables with identical embedding vector sizes, the distributed sparse embedding combines them as one huge embedding table. Each sub-embedding-table is called slot, which is also known as feature-field. To avoid ambiguity, the input keys for across embedding tables should be represented using a unified encoding.
When conducting reduction of embedding vectors intra slots (feature-fields), SOK will use the collective operation Reduce-Scatter
. All-Gather
is used for the accumulation of gradient during backward propagation.
Dense Embedding Layer
SOK’s dense embedding layer is equivalent to tf.nn.embedding_lookup
, except that it works in a MP manner.
All2All Dense Embedding
The all-2-all dense embedding will distribute each key based on gpu_id = key % gpu_num
. For example, if there are 8 GPUs, then key=1000
will be deployed to GPU-0, key=1001
will be deployed to GPU-1. The following picture illustrates the forward propagation process.
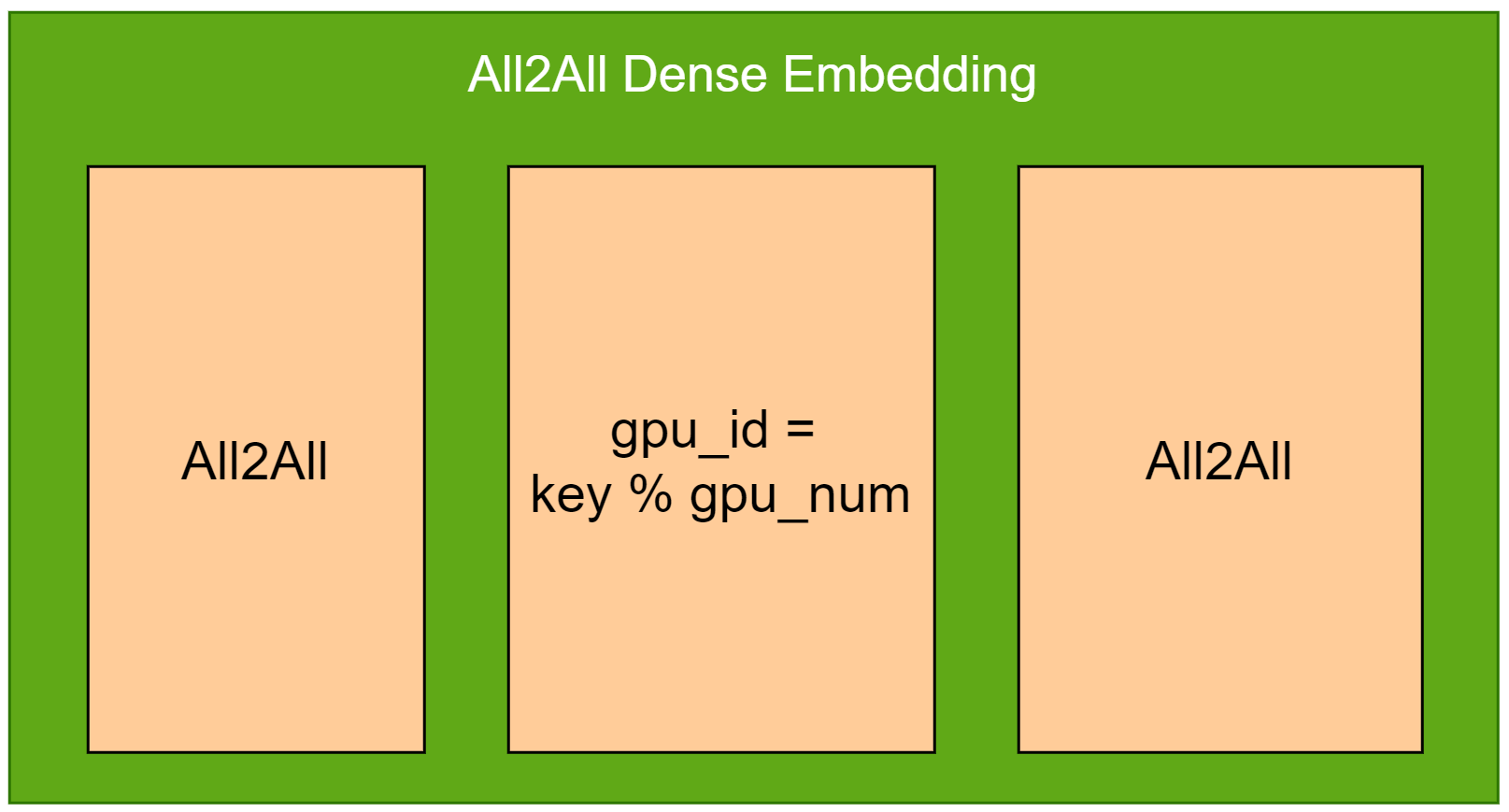
To reduce the overhead when looking up multiple embedding tables with identical embedding vector sizes, the all-2-all dense embedding combines them as one huge embedding table. Each sub-embedding-table is called slot, which is also known as feature-field. To avoid ambiguity, the input keys for across embedding tables should be represented using a unified encoding.
During forward propagation, an All2All
communication primitive is first used to exchange keys among all GPUs. Then, another All2All
is used to exchange embedding vectors among all GPUs. During backward propagation, All2All
is used to exchange top gradients among all GPUs.