# Copyright 2021 NVIDIA Corporation. All Rights Reserved.
#
# Licensed under the Apache License, Version 2.0 (the "License");
# you may not use this file except in compliance with the License.
# You may obtain a copy of the License at
#
# http://www.apache.org/licenses/LICENSE-2.0
#
# Unless required by applicable law or agreed to in writing, software
# distributed under the License is distributed on an "AS IS" BASIS,
# WITHOUT WARRANTIES OR CONDITIONS OF ANY KIND, either express or implied.
# See the License for the specific language governing permissions and
# limitations under the License.
# ==============================================================================
# Each user is responsible for checking the content of datasets and the
# applicable licenses and determining if suitable for the intended use.
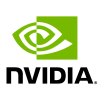
HugeCTR End-end Example with NVTabular
Overview
In this sample notebook, we are going to:
Preprocess data using NVTabular
Training model with HugeCTR
Do offline inference using HugeCTR HPS
Setup
To setup the environment, refer to HugeCTR Example Notebooks and follow the instructions there before running the following.
Data Preparation
import os
import shutil
!mkdir -p /hugectr_e2e
!mkdir -p /hugectr_e2e/criteo/train
!mkdir -p /hugectr_e2e/criteo/val
!mkdir -p /hugectr_e2e/model
BASE_DIR = os.environ.get("BASE_DIR", "/hugectr_e2e")
DATA_DIR = os.environ.get("DATA_DIR", BASE_DIR + "/criteo")
TRAIN_DIR = os.environ.get("TRAIN_DIR", DATA_DIR +"/train")
VAL_DIR = os.environ.get("VAL_DIR", DATA_DIR +"/val")
MODEL_DIR = os.environ.get("MODEL_DIR", BASE_DIR + "/model")
Download the Criteo data for 1 day:
#!wget -P $DATA_DIR https://storage.googleapis.com/criteo-cail-datasets/day_0.gz #decomment this line to download, otherwise soft link the data.
#!gzip -d -c $DATA_DIR/day_0.gz > $DATA_DIR/day_0
INPUT_DATA = os.environ.get("INPUT_DATA", DATA_DIR + "/day_0")
!ln -s $INPUT_DATA $DATA_DIR/day_0
ln: failed to create symbolic link '/hugectr_e2e/criteo/day_0': File exists
Unzip and split data
!head -n 10000000 $DATA_DIR/day_0 > $DATA_DIR/train/train.txt
!tail -n 2000000 $DATA_DIR/day_0 > $DATA_DIR/val/test.txt
Data Preprocessing using NVTabular
import warnings
warnings.filterwarnings("ignore")
warnings.simplefilter("ignore", UserWarning)
import os
import sys
import argparse
import glob
import time
import numpy as np
import shutil
import numba
import dask_cudf
from dask_cuda import LocalCUDACluster
from dask.distributed import Client
import nvtabular as nvt
from merlin.core.compat import device_mem_size, pynvml_mem_size
from nvtabular.ops import (
Categorify,
Clip,
FillMissing,
Normalize,
get_embedding_sizes,
)
import logging
logging.basicConfig(format="%(asctime)s %(message)s")
logging.root.setLevel(logging.NOTSET)
logging.getLogger("numba").setLevel(logging.WARNING)
logging.getLogger("asyncio").setLevel(logging.WARNING)
# define dataset schema
CATEGORICAL_COLUMNS=["C" + str(x) for x in range(1, 27)]
CONTINUOUS_COLUMNS=["I" + str(x) for x in range(1, 14)]
LABEL_COLUMNS = ['label']
COLUMNS = LABEL_COLUMNS + CONTINUOUS_COLUMNS + CATEGORICAL_COLUMNS
#/samples/criteo mode doesn't have dense features
criteo_COLUMN=LABEL_COLUMNS + CATEGORICAL_COLUMNS
#For new feature cross columns
CROSS_COLUMNS = ["C1_C2", "C3_C4"]
NUM_INTEGER_COLUMNS = 13
NUM_CATEGORICAL_COLUMNS = 26
NUM_TOTAL_COLUMNS = 1 + NUM_INTEGER_COLUMNS + NUM_CATEGORICAL_COLUMNS
# Dask dashboard
dashboard_port = "8787"
# Deploy a Single-Machine Multi-GPU Cluster
protocol = "tcp" # "tcp" or "ucx"
if numba.cuda.is_available():
NUM_GPUS = list(range(len(numba.cuda.gpus)))
else:
NUM_GPUS = []
visible_devices = ",".join([str(n) for n in NUM_GPUS]) # Delect devices to place workers
device_limit_frac = 0.7 # Spill GPU-Worker memory to host at this limit.
device_pool_frac = 0.8
part_mem_frac = 0.15
# Use total device size to calculate args.device_limit_frac
device_size = device_mem_size(kind="total")
device_limit = int(device_limit_frac * device_size)
device_pool_size = int(device_pool_frac * device_size)
part_size = int(part_mem_frac * device_size)
# Check if any device memory is already occupied
for dev in visible_devices.split(","):
fmem = pynvml_mem_size(kind="free", index=int(dev))
used = (device_size - fmem) / 1e9
if used > 1.0:
warnings.warn(f"BEWARE - {used} GB is already occupied on device {int(dev)}!")
cluster = None # (Optional) Specify existing scheduler port
if cluster is None:
cluster = LocalCUDACluster(
protocol=protocol,
n_workers=len(visible_devices.split(",")),
CUDA_VISIBLE_DEVICES=visible_devices,
device_memory_limit=device_limit,
dashboard_address=":" + dashboard_port,
rmm_pool_size=(device_pool_size // 256) * 256
)
# Create the distributed client
client = Client(cluster)
client
2023-05-26 03:09:04,061 - distributed.preloading - INFO - Creating preload: dask_cuda.initialize
2023-05-26 03:09:04,061 - distributed.preloading - INFO - Import preload module: dask_cuda.initialize
2023-05-26 03:09:04,062 - distributed.preloading - INFO - Creating preload: dask_cuda.initialize
2023-05-26 03:09:04,062 - distributed.preloading - INFO - Import preload module: dask_cuda.initialize
2023-05-26 03:09:04,063 - distributed.preloading - INFO - Creating preload: dask_cuda.initialize
2023-05-26 03:09:04,063 - distributed.preloading - INFO - Import preload module: dask_cuda.initialize
2023-05-26 03:09:04,064 - distributed.preloading - INFO - Creating preload: dask_cuda.initialize
2023-05-26 03:09:04,064 - distributed.preloading - INFO - Import preload module: dask_cuda.initialize
2023-05-26 03:09:04,072 - distributed.preloading - INFO - Creating preload: dask_cuda.initialize
2023-05-26 03:09:04,072 - distributed.preloading - INFO - Import preload module: dask_cuda.initialize
2023-05-26 03:09:04,085 - distributed.preloading - INFO - Creating preload: dask_cuda.initialize
2023-05-26 03:09:04,085 - distributed.preloading - INFO - Import preload module: dask_cuda.initialize
2023-05-26 03:09:04,087 - distributed.preloading - INFO - Creating preload: dask_cuda.initialize
2023-05-26 03:09:04,087 - distributed.preloading - INFO - Import preload module: dask_cuda.initialize
2023-05-26 03:09:04,093 - distributed.preloading - INFO - Creating preload: dask_cuda.initialize
2023-05-26 03:09:04,093 - distributed.preloading - INFO - Import preload module: dask_cuda.initialize
Client
Client-acc90f7f-fb72-11ed-808f-54ab3adac0a5
Connection method: Cluster object | Cluster type: dask_cuda.LocalCUDACluster |
Dashboard: http://127.0.0.1:8787/status |
Cluster Info
LocalCUDACluster
c0d46f34
Dashboard: http://127.0.0.1:8787/status | Workers: 8 |
Total threads: 8 | Total memory: 503.79 GiB |
Status: running | Using processes: True |
Scheduler Info
Scheduler
Scheduler-789d4132-7d07-451f-aced-1867dfa9d7b3
Comm: tcp://127.0.0.1:33423 | Workers: 8 |
Dashboard: http://127.0.0.1:8787/status | Total threads: 8 |
Started: Just now | Total memory: 503.79 GiB |
Workers
Worker: 0
Comm: tcp://127.0.0.1:40925 | Total threads: 1 |
Dashboard: http://127.0.0.1:43851/status | Memory: 62.97 GiB |
Nanny: tcp://127.0.0.1:40143 | |
Local directory: /tmp/dask-worker-space/worker-6pb36hck | |
GPU: Tesla V100-SXM2-32GB | GPU memory: 31.75 GiB |
Worker: 1
Comm: tcp://127.0.0.1:40769 | Total threads: 1 |
Dashboard: http://127.0.0.1:44353/status | Memory: 62.97 GiB |
Nanny: tcp://127.0.0.1:43979 | |
Local directory: /tmp/dask-worker-space/worker-rv8itza6 | |
GPU: Tesla V100-SXM2-32GB | GPU memory: 31.75 GiB |
Worker: 2
Comm: tcp://127.0.0.1:45255 | Total threads: 1 |
Dashboard: http://127.0.0.1:37165/status | Memory: 62.97 GiB |
Nanny: tcp://127.0.0.1:46241 | |
Local directory: /tmp/dask-worker-space/worker-co2ru8ea | |
GPU: Tesla V100-SXM2-32GB | GPU memory: 31.75 GiB |
Worker: 3
Comm: tcp://127.0.0.1:40555 | Total threads: 1 |
Dashboard: http://127.0.0.1:37399/status | Memory: 62.97 GiB |
Nanny: tcp://127.0.0.1:36117 | |
Local directory: /tmp/dask-worker-space/worker-04qjh_rt | |
GPU: Tesla V100-SXM2-32GB | GPU memory: 31.75 GiB |
Worker: 4
Comm: tcp://127.0.0.1:39951 | Total threads: 1 |
Dashboard: http://127.0.0.1:42631/status | Memory: 62.97 GiB |
Nanny: tcp://127.0.0.1:46323 | |
Local directory: /tmp/dask-worker-space/worker-9zzs6cz6 | |
GPU: Tesla V100-SXM2-32GB | GPU memory: 31.75 GiB |
Worker: 5
Comm: tcp://127.0.0.1:40815 | Total threads: 1 |
Dashboard: http://127.0.0.1:37909/status | Memory: 62.97 GiB |
Nanny: tcp://127.0.0.1:40533 | |
Local directory: /tmp/dask-worker-space/worker-hq437puc | |
GPU: Tesla V100-SXM2-32GB | GPU memory: 31.75 GiB |
Worker: 6
Comm: tcp://127.0.0.1:42963 | Total threads: 1 |
Dashboard: http://127.0.0.1:41947/status | Memory: 62.97 GiB |
Nanny: tcp://127.0.0.1:42201 | |
Local directory: /tmp/dask-worker-space/worker-kuwua5fi | |
GPU: Tesla V100-SXM2-32GB | GPU memory: 31.75 GiB |
Worker: 7
Comm: tcp://127.0.0.1:39607 | Total threads: 1 |
Dashboard: http://127.0.0.1:45371/status | Memory: 62.97 GiB |
Nanny: tcp://127.0.0.1:39667 | |
Local directory: /tmp/dask-worker-space/worker-ouyeimq6 | |
GPU: Tesla V100-SXM2-32GB | GPU memory: 31.75 GiB |
train_output = os.path.join(DATA_DIR, "train")
print("Training output data: "+train_output)
val_output = os.path.join(DATA_DIR, "val")
print("Validation output data: "+val_output)
train_input = os.path.join(DATA_DIR, "train/train.txt")
print("Training dataset: "+train_input)
val_input = os.path.join(DATA_DIR, "val/test.txt")
PREPROCESS_DIR_temp_train = os.path.join(DATA_DIR, 'train/temp-parquet-after-conversion')
PREPROCESS_DIR_temp_val = os.path.join(DATA_DIR, "val/temp-parquet-after-conversion")
if not os.path.exists(PREPROCESS_DIR_temp_train):
os.makedirs(PREPROCESS_DIR_temp_train)
if not os.path.exists(PREPROCESS_DIR_temp_val):
os.makedirs(PREPROCESS_DIR_temp_val)
PREPROCESS_DIR_temp = [PREPROCESS_DIR_temp_train, PREPROCESS_DIR_temp_val]
# Make sure we have a clean parquet space for cudf conversion
for one_path in PREPROCESS_DIR_temp:
if os.path.exists(one_path):
shutil.rmtree(one_path)
os.mkdir(one_path)
#calculate the total processing time
runtime = time.time()
## train/valid txt to parquet
train_valid_paths = [(train_input,PREPROCESS_DIR_temp_train),(val_input,PREPROCESS_DIR_temp_val)]
for input, temp_output in train_valid_paths:
ddf = dask_cudf.read_csv(input,sep='\t',names=LABEL_COLUMNS + CONTINUOUS_COLUMNS + CATEGORICAL_COLUMNS)
if CROSS_COLUMNS:
for pair in CROSS_COLUMNS:
feature_pair = pair.split("_")
ddf[pair] = ddf[feature_pair[0]] + ddf[feature_pair[1]]
ddf["label"] = ddf['label'].astype('float32')
ddf[CONTINUOUS_COLUMNS] = ddf[CONTINUOUS_COLUMNS].astype('float32')
# Save it as parquet format for better memory usage
ddf.to_parquet(temp_output,header=True)
##-----------------------------------##
COLUMNS = LABEL_COLUMNS + CONTINUOUS_COLUMNS + CROSS_COLUMNS + CATEGORICAL_COLUMNS
train_paths = glob.glob(os.path.join(PREPROCESS_DIR_temp_train, "*.parquet"))
valid_paths = glob.glob(os.path.join(PREPROCESS_DIR_temp_val, "*.parquet"))
categorify_op = Categorify()
cat_features = CATEGORICAL_COLUMNS >> categorify_op
cont_features = CONTINUOUS_COLUMNS >> FillMissing() >> Clip(min_value=0) >> Normalize()
cross_cat_op = Categorify()
features = LABEL_COLUMNS
features += cont_features
if CROSS_COLUMNS:
for pair in CROSS_COLUMNS:
features += [pair] >> cross_cat_op
features += cat_features
workflow = nvt.Workflow(features, client=client)
logging.info("Preprocessing")
output_format = 'parquet'
# just for /samples/criteo model
train_ds_iterator = nvt.Dataset(train_paths, engine='parquet')
valid_ds_iterator = nvt.Dataset(valid_paths, engine='parquet')
shuffle = nvt.io.Shuffle.PER_PARTITION
logging.info('Train Datasets Preprocessing.....')
dict_dtypes = {}
for col in CATEGORICAL_COLUMNS:
dict_dtypes[col] = np.int64
for col in CONTINUOUS_COLUMNS:
dict_dtypes[col] = np.float32
for col in CROSS_COLUMNS:
dict_dtypes[col] = np.int64
for col in LABEL_COLUMNS:
dict_dtypes[col] = np.float32
conts = CONTINUOUS_COLUMNS
workflow.fit(train_ds_iterator)
if output_format == 'hugectr':
workflow.transform(train_ds_iterator).to_hugectr(
cats=CATEGORICAL_COLUMNS + CROSS_COLUMNS,
conts=conts,
labels=LABEL_COLUMNS,
output_path=train_output,
shuffle=shuffle)
else:
workflow.transform(train_ds_iterator).to_parquet(
output_path=train_output,
dtypes=dict_dtypes,
cats=CATEGORICAL_COLUMNS + CROSS_COLUMNS,
conts=conts,
labels=LABEL_COLUMNS,
shuffle=shuffle)
###Getting slot size###
#--------------------##
embeddings_dict_cat = categorify_op.get_embedding_sizes(CATEGORICAL_COLUMNS)
embeddings_dict_cross = cross_cat_op.get_embedding_sizes(CROSS_COLUMNS)
embeddings = [embeddings_dict_cat[c][0] for c in CATEGORICAL_COLUMNS] + [embeddings_dict_cross[c][0] for c in CROSS_COLUMNS]
print(embeddings)
##--------------------##
logging.info('Valid Datasets Preprocessing.....')
if output_format == 'hugectr':
workflow.transform(valid_ds_iterator).to_hugectr(
cats=CATEGORICAL_COLUMNS + CROSS_COLUMNS,
conts=conts,
labels=LABEL_COLUMNS,
output_path=val_output,
shuffle=shuffle)
else:
workflow.transform(valid_ds_iterator).to_parquet(
output_path=val_output,
dtypes=dict_dtypes,
cats=CATEGORICAL_COLUMNS + CROSS_COLUMNS,
conts=conts,
labels=LABEL_COLUMNS,
shuffle=shuffle)
embeddings_dict_cat = categorify_op.get_embedding_sizes(CATEGORICAL_COLUMNS)
embeddings_dict_cross = cross_cat_op.get_embedding_sizes(CROSS_COLUMNS)
embeddings = [embeddings_dict_cat[c][0] for c in CATEGORICAL_COLUMNS] + [embeddings_dict_cross[c][0] for c in CROSS_COLUMNS]
print(embeddings)
##--------------------##
## Shutdown clusters
client.shutdown()
runtime = time.time() - runtime
print("\nDask-NVTabular Criteo Preprocessing Done!")
print(f"Runtime[s] | {runtime}")
print("======================================\n")
Training output data: /hugectr_e2e/criteo/train
Validation output data: /hugectr_e2e/criteo/val
Training dataset: /hugectr_e2e/criteo/train/train.txt
2023-05-26 03:09:49,967 Preprocessing
2023-05-26 03:09:50,513 Train Datasets Preprocessing.....
2023-05-26 03:09:57,544 Valid Datasets Preprocessing.....
[1234907, 19683, 13780, 6867, 18490, 4, 6264, 1235, 50, 854680, 114026, 75736, 11, 2159, 7533, 61, 4, 919, 15, 1307783, 404742, 1105613, 87714, 9032, 77, 34, 1577645, 1093030]
[1234907, 19683, 13780, 6867, 18490, 4, 6264, 1235, 50, 854680, 114026, 75736, 11, 2159, 7533, 61, 4, 919, 15, 1307783, 404742, 1105613, 87714, 9032, 77, 34, 1577645, 1093030]
Dask-NVTabular Criteo Preprocessing Done!
Runtime[s] | 11.187256813049316
======================================
### Record the slot size array
SLOT_SIZE_ARRAY = embeddings
Training a WDL model with HugeCTR
%%writefile './train.py'
import hugectr
import os
import argparse
from mpi4py import MPI
parser = argparse.ArgumentParser(description=("HugeCTR Training"))
parser.add_argument("--data_path", type=str, help="Input dataset path (Required)")
parser.add_argument("--model_path", type=str, help="Directory path to write output (Required)")
args = parser.parse_args()
SLOT_SIZE_ARRAY = [1234907, 19683, 13780, 6867, 18490, 4, 6264, 1235, 50, 854680, 114026, 75736, 11, 2159, 7533, 61, 4, 919, 15, 1307783, 404742, 1105613, 87714, 9032, 77, 34, 1581605, 1093030]
solver = hugectr.CreateSolver(max_eval_batches = 4000,
batchsize_eval = 2720,
batchsize = 2720,
lr = 0.001,
vvgpu = [[0]],
repeat_dataset = True,
i64_input_key = True)
reader = hugectr.DataReaderParams(data_reader_type = hugectr.DataReaderType_t.Parquet,
source = [os.path.join(args.data_path, "train/_file_list.txt")],
eval_source = os.path.join(args.data_path, "val/_file_list.txt"),
check_type = hugectr.Check_t.Non,
slot_size_array = SLOT_SIZE_ARRAY)
optimizer = hugectr.CreateOptimizer(optimizer_type = hugectr.Optimizer_t.Adam,
update_type = hugectr.Update_t.Global,
beta1 = 0.9,
beta2 = 0.999,
epsilon = 0.0000001)
model = hugectr.Model(solver, reader, optimizer)
model.add(hugectr.Input(label_dim = 1, label_name = "label",
dense_dim = 13, dense_name = "dense",
data_reader_sparse_param_array =
[hugectr.DataReaderSparseParam("wide_data", 1, True, 2),
hugectr.DataReaderSparseParam("deep_data", 2, False, 26)]))
model.add(hugectr.SparseEmbedding(embedding_type = hugectr.Embedding_t.DistributedSlotSparseEmbeddingHash,
workspace_size_per_gpu_in_mb = 80,
embedding_vec_size = 1,
combiner = "sum",
sparse_embedding_name = "sparse_embedding2",
bottom_name = "wide_data",
optimizer = optimizer))
model.add(hugectr.SparseEmbedding(embedding_type = hugectr.Embedding_t.DistributedSlotSparseEmbeddingHash,
workspace_size_per_gpu_in_mb = 1350,
embedding_vec_size = 16,
combiner = "sum",
sparse_embedding_name = "sparse_embedding1",
bottom_name = "deep_data",
optimizer = optimizer))
model.add(hugectr.DenseLayer(layer_type = hugectr.Layer_t.Reshape,
bottom_names = ["sparse_embedding1"],
top_names = ["reshape1"],
leading_dim=416))
model.add(hugectr.DenseLayer(layer_type = hugectr.Layer_t.Reshape,
bottom_names = ["sparse_embedding2"],
top_names = ["reshape2"],
leading_dim=2))
model.add(hugectr.DenseLayer(layer_type = hugectr.Layer_t.ReduceSum,
bottom_names = ["reshape2"],
top_names = ["wide_redn"],
axis = 1))
model.add(hugectr.DenseLayer(layer_type = hugectr.Layer_t.Concat,
bottom_names = ["reshape1", "dense"],
top_names = ["concat1"]))
model.add(hugectr.DenseLayer(layer_type = hugectr.Layer_t.InnerProduct,
bottom_names = ["concat1"],
top_names = ["fc1"],
num_output=1024))
model.add(hugectr.DenseLayer(layer_type = hugectr.Layer_t.ReLU,
bottom_names = ["fc1"],
top_names = ["relu1"]))
model.add(hugectr.DenseLayer(layer_type = hugectr.Layer_t.Dropout,
bottom_names = ["relu1"],
top_names = ["dropout1"],
dropout_rate=0.5))
model.add(hugectr.DenseLayer(layer_type = hugectr.Layer_t.InnerProduct,
bottom_names = ["dropout1"],
top_names = ["fc2"],
num_output=1024))
model.add(hugectr.DenseLayer(layer_type = hugectr.Layer_t.ReLU,
bottom_names = ["fc2"],
top_names = ["relu2"]))
model.add(hugectr.DenseLayer(layer_type = hugectr.Layer_t.Dropout,
bottom_names = ["relu2"],
top_names = ["dropout2"],
dropout_rate=0.5))
model.add(hugectr.DenseLayer(layer_type = hugectr.Layer_t.InnerProduct,
bottom_names = ["dropout2"],
top_names = ["fc3"],
num_output=1))
model.add(hugectr.DenseLayer(layer_type = hugectr.Layer_t.Add,
bottom_names = ["fc3", "wide_redn"],
top_names = ["add1"]))
model.add(hugectr.DenseLayer(layer_type = hugectr.Layer_t.BinaryCrossEntropyLoss,
bottom_names = ["add1", "label"],
top_names = ["loss"]))
model.compile()
model.summary()
model.fit(max_iter = 21000, display = 1000, eval_interval = 4000, snapshot = 20000, snapshot_prefix = os.path.join(args.model_path, "wdl/"))
model.graph_to_json(graph_config_file = os.path.join(args.model_path, "wdl.json"))
Writing ./train.py
!python train.py --data_path $DATA_DIR --model_path $MODEL_DIR
MpiInitService: MPI was already initialized by another (non-HugeCTR) mechanism.
HugeCTR Version: 23.4
====================================================Model Init=====================================================
[HCTR][03:10:28.412][WARNING][RK0][main]: The model name is not specified when creating the solver.
[HCTR][03:10:28.413][INFO][RK0][main]: Global seed is 4031005480
[HCTR][03:10:29.069][INFO][RK0][main]: Device to NUMA mapping:
GPU 0 -> node 0
[HCTR][03:10:32.353][WARNING][RK0][main]: Peer-to-peer access cannot be fully enabled.
[HCTR][03:10:32.353][DEBUG][RK0][main]: [device 0] allocating 0.0000 GB, available 29.9792
[HCTR][03:10:32.353][INFO][RK0][main]: Start all2all warmup
[HCTR][03:10:32.353][INFO][RK0][main]: End all2all warmup
[HCTR][03:10:32.355][INFO][RK0][main]: Using All-reduce algorithm: NCCL
[HCTR][03:10:32.361][INFO][RK0][main]: Device 0: Tesla V100-SXM2-32GB
[HCTR][03:10:32.362][INFO][RK0][main]: eval source /hugectr_e2e/criteo/val/_file_list.txt max_row_group_size 475000
[HCTR][03:10:32.364][INFO][RK0][main]: train source /hugectr_e2e/criteo/train/_file_list.txt max_row_group_size 475000
[HCTR][03:10:32.364][INFO][RK0][main]: num of DataReader workers for train: 1
[HCTR][03:10:32.364][INFO][RK0][main]: num of DataReader workers for eval: 1
[HCTR][03:10:32.365][DEBUG][RK0][main]: [device 0] allocating 0.0018 GB, available 29.7234
[HCTR][03:10:32.366][DEBUG][RK0][main]: [device 0] allocating 0.0018 GB, available 29.7175
[HCTR][03:10:32.379][INFO][RK0][main]: Vocabulary size: 7946054
[HCTR][03:10:32.380][INFO][RK0][main]: max_vocabulary_size_per_gpu_=6990506
[HCTR][03:10:32.391][DEBUG][RK0][main]: [device 0] allocating 0.0788 GB, available 28.3132
[HCTR][03:10:32.392][INFO][RK0][main]: max_vocabulary_size_per_gpu_=7372800
[HCTR][03:10:32.396][DEBUG][RK0][main]: [device 0] allocating 1.3516 GB, available 26.5847
[HCTR][03:10:32.397][INFO][RK0][main]: Graph analysis to resolve tensor dependency
===================================================Model Compile===================================================
[HCTR][03:10:32.408][DEBUG][RK0][main]: [device 0] allocating 0.2162 GB, available 26.3523
[HCTR][03:10:32.409][DEBUG][RK0][main]: [device 0] allocating 0.0056 GB, available 26.3464
[HCTR][03:10:40.869][INFO][RK0][main]: gpu0 start to init embedding
[HCTR][03:10:40.869][INFO][RK0][main]: gpu0 init embedding done
[HCTR][03:10:40.869][INFO][RK0][main]: gpu0 start to init embedding
[HCTR][03:10:40.873][INFO][RK0][main]: gpu0 init embedding done
[HCTR][03:10:40.873][DEBUG][RK0][main]: [device 0] allocating 0.0001 GB, available 26.3464
[HCTR][03:10:40.874][INFO][RK0][main]: Starting AUC NCCL warm-up
[HCTR][03:10:40.879][INFO][RK0][main]: Warm-up done
===================================================Model Summary===================================================
[HCTR][03:10:40.879][INFO][RK0][main]: Model structure on each GPU
Label Dense Sparse
label dense wide_data,deep_data
(2720,1) (2720,13)
——————————————————————————————————————————————————————————————————————————————————————————————————————————————————
Layer Type Input Name Output Name Output Shape
——————————————————————————————————————————————————————————————————————————————————————————————————————————————————
DistributedSlotSparseEmbeddingHash wide_data sparse_embedding2 (2720,2,1)
------------------------------------------------------------------------------------------------------------------
DistributedSlotSparseEmbeddingHash deep_data sparse_embedding1 (2720,26,16)
------------------------------------------------------------------------------------------------------------------
Reshape sparse_embedding1 reshape1 (2720,416)
------------------------------------------------------------------------------------------------------------------
Reshape sparse_embedding2 reshape2 (2720,2)
------------------------------------------------------------------------------------------------------------------
ReduceSum reshape2 wide_redn (2720,1)
------------------------------------------------------------------------------------------------------------------
Concat reshape1 concat1 (2720,429)
dense
------------------------------------------------------------------------------------------------------------------
InnerProduct concat1 fc1 (2720,1024)
------------------------------------------------------------------------------------------------------------------
ReLU fc1 relu1 (2720,1024)
------------------------------------------------------------------------------------------------------------------
Dropout relu1 dropout1 (2720,1024)
------------------------------------------------------------------------------------------------------------------
InnerProduct dropout1 fc2 (2720,1024)
------------------------------------------------------------------------------------------------------------------
ReLU fc2 relu2 (2720,1024)
------------------------------------------------------------------------------------------------------------------
Dropout relu2 dropout2 (2720,1024)
------------------------------------------------------------------------------------------------------------------
InnerProduct dropout2 fc3 (2720,1)
------------------------------------------------------------------------------------------------------------------
Add fc3 add1 (2720,1)
wide_redn
------------------------------------------------------------------------------------------------------------------
BinaryCrossEntropyLoss add1 loss
label
------------------------------------------------------------------------------------------------------------------
=====================================================Model Fit=====================================================
[HCTR][03:10:40.879][INFO][RK0][main]: Use non-epoch mode with number of iterations: 21000
[HCTR][03:10:40.879][INFO][RK0][main]: Training batchsize: 2720, evaluation batchsize: 2720
[HCTR][03:10:40.879][INFO][RK0][main]: Evaluation interval: 4000, snapshot interval: 20000
[HCTR][03:10:40.879][INFO][RK0][main]: Dense network trainable: True
[HCTR][03:10:40.879][INFO][RK0][main]: Sparse embedding sparse_embedding1 trainable: True
[HCTR][03:10:40.879][INFO][RK0][main]: Sparse embedding sparse_embedding2 trainable: True
[HCTR][03:10:40.879][INFO][RK0][main]: Use mixed precision: False, scaler: 1.000000, use cuda graph: True
[HCTR][03:10:40.879][INFO][RK0][main]: lr: 0.001000, warmup_steps: 1, end_lr: 0.000000
[HCTR][03:10:40.879][INFO][RK0][main]: decay_start: 0, decay_steps: 1, decay_power: 2.000000
[HCTR][03:10:40.879][INFO][RK0][main]: Training source file: /hugectr_e2e/criteo/train/_file_list.txt
[HCTR][03:10:40.879][INFO][RK0][main]: Evaluation source file: /hugectr_e2e/criteo/val/_file_list.txt
[HCTR][03:10:49.588][INFO][RK0][main]: Iter: 1000 Time(1000 iters): 8.70458s Loss: 0.124098 lr:0.001
[HCTR][03:10:58.211][INFO][RK0][main]: Iter: 2000 Time(1000 iters): 8.6176s Loss: 0.130088 lr:0.001
[HCTR][03:11:06.835][INFO][RK0][main]: Iter: 3000 Time(1000 iters): 8.61959s Loss: 0.101731 lr:0.001
[HCTR][03:11:15.449][INFO][RK0][main]: Iter: 4000 Time(1000 iters): 8.61009s Loss: 0.110557 lr:0.001
[HCTR][03:11:19.929][INFO][RK0][main]: Evaluation, AUC: 0.738497
[HCTR][03:11:19.929][INFO][RK0][main]: Eval Time for 4000 iters: 4.47924s
[HCTR][03:11:28.559][INFO][RK0][main]: Iter: 5000 Time(1000 iters): 13.1046s Loss: 0.10236 lr:0.001
[HCTR][03:11:37.182][INFO][RK0][main]: Iter: 6000 Time(1000 iters): 8.61852s Loss: 0.102157 lr:0.001
[HCTR][03:11:45.771][INFO][RK0][main]: Iter: 7000 Time(1000 iters): 8.58452s Loss: 0.123451 lr:0.001
[HCTR][03:11:54.385][INFO][RK0][main]: Iter: 8000 Time(1000 iters): 8.61023s Loss: 0.122763 lr:0.001
[HCTR][03:11:58.867][INFO][RK0][main]: Evaluation, AUC: 0.698276
[HCTR][03:11:58.867][INFO][RK0][main]: Eval Time for 4000 iters: 4.48087s
[HCTR][03:12:07.487][INFO][RK0][main]: Iter: 9000 Time(1000 iters): 13.097s Loss: 0.0999177 lr:0.001
[HCTR][03:12:16.103][INFO][RK0][main]: Iter: 10000 Time(1000 iters): 8.61106s Loss: 0.0999892 lr:0.001
[HCTR][03:12:24.722][INFO][RK0][main]: Iter: 11000 Time(1000 iters): 8.61545s Loss: 0.0883301 lr:0.001
[HCTR][03:12:33.348][INFO][RK0][main]: Iter: 12000 Time(1000 iters): 8.62134s Loss: 0.0828304 lr:0.001
[HCTR][03:12:37.823][INFO][RK0][main]: Evaluation, AUC: 0.688598
[HCTR][03:12:37.823][INFO][RK0][main]: Eval Time for 4000 iters: 4.4733s
[HCTR][03:12:46.425][INFO][RK0][main]: Iter: 13000 Time(1000 iters): 13.0717s Loss: 0.108287 lr:0.001
[HCTR][03:12:55.059][INFO][RK0][main]: Iter: 14000 Time(1000 iters): 8.62997s Loss: 0.0745141 lr:0.001
[HCTR][03:13:03.671][INFO][RK0][main]: Iter: 15000 Time(1000 iters): 8.60764s Loss: 0.0720452 lr:0.001
[HCTR][03:13:12.287][INFO][RK0][main]: Iter: 16000 Time(1000 iters): 8.61101s Loss: 0.0851126 lr:0.001
[HCTR][03:13:16.758][INFO][RK0][main]: Evaluation, AUC: 0.685426
[HCTR][03:13:16.758][INFO][RK0][main]: Eval Time for 4000 iters: 4.47088s
[HCTR][03:13:25.378][INFO][RK0][main]: Iter: 17000 Time(1000 iters): 13.0865s Loss: 0.0632745 lr:0.001
[HCTR][03:13:34.011][INFO][RK0][main]: Iter: 18000 Time(1000 iters): 8.62825s Loss: 0.0742994 lr:0.001
[HCTR][03:13:42.626][INFO][RK0][main]: Iter: 19000 Time(1000 iters): 8.61035s Loss: 0.0679226 lr:0.001
[HCTR][03:13:51.230][INFO][RK0][main]: Iter: 20000 Time(1000 iters): 8.59954s Loss: 0.0779185 lr:0.001
[HCTR][03:13:55.704][INFO][RK0][main]: Evaluation, AUC: 0.684045
[HCTR][03:13:55.704][INFO][RK0][main]: Eval Time for 4000 iters: 4.4736s
[HCTR][03:13:55.733][INFO][RK0][main]: Rank0: Write hash table to file
[HCTR][03:13:55.902][INFO][RK0][main]: Rank0: Write hash table to file
[HCTR][03:13:56.075][INFO][RK0][main]: Dumping sparse weights to files, successful
[HCTR][03:13:56.091][INFO][RK0][main]: Rank0: Write optimzer state to file
[HCTR][03:13:56.104][INFO][RK0][main]: Done
[HCTR][03:13:56.119][INFO][RK0][main]: Rank0: Write optimzer state to file
[HCTR][03:13:56.133][INFO][RK0][main]: Done
[HCTR][03:13:56.398][INFO][RK0][main]: Rank0: Write optimzer state to file
[HCTR][03:13:56.611][INFO][RK0][main]: Done
[HCTR][03:13:56.903][INFO][RK0][main]: Rank0: Write optimzer state to file
[HCTR][03:13:57.152][INFO][RK0][main]: Done
[HCTR][03:13:57.169][INFO][RK0][main]: Dumping sparse optimzer states to files, successful
[HCTR][03:13:57.176][INFO][RK0][main]: Dumping dense weights to file, successful
[HCTR][03:13:57.188][INFO][RK0][main]: Dumping dense optimizer states to file, successful
[HCTR][03:14:05.788][INFO][RK0][main]: Iter: 21000 Time(1000 iters): 14.5538s Loss: 0.0770708 lr:0.001
[HCTR][03:14:05.788][INFO][RK0][main]: Finish 21000 iterations with batchsize: 2720 in 204.91s.
[HCTR][03:14:05.788][INFO][RK0][main]: Save the model graph to /hugectr_e2e/model/wdl.json successfully
Load model to HPS and inference with HugeCTR
from hugectr.inference import InferenceModel, InferenceParams
import hugectr
import os
model_config = os.path.join(MODEL_DIR, "wdl.json")
inference_params = InferenceParams(
model_name = "wdl",
max_batchsize = 1024,
hit_rate_threshold = 1.0,
dense_model_file = os.path.join(MODEL_DIR, "wdl/_dense_20000.model"),
sparse_model_files = [os.path.join(MODEL_DIR, "wdl/0_sparse_20000.model"), os.path.join(MODEL_DIR, "wdl/1_sparse_20000.model")],
deployed_devices = [0],
use_gpu_embedding_cache = True,
cache_size_percentage = 1.0,
i64_input_key = True
)
inference_model = InferenceModel(model_config, inference_params)
pred = inference_model.predict(
10,
"/hugectr_e2e/criteo/val/_file_list.txt",
hugectr.DataReaderType_t.Parquet,
hugectr.Check_t.Non,
SLOT_SIZE_ARRAY
)
print(pred.shape)
print(pred)
[HCTR][03:14:16.183][WARNING][RK0][main]: default_value_for_each_table.size() is not equal to the number of embedding tables
[HCTR][03:14:16.185][INFO][RK0][main]: Global seed is 1256414940
[HCTR][03:14:16.306][INFO][RK0][main]: Device to NUMA mapping:
GPU 0 -> node 0
[HCTR][03:14:18.054][WARNING][RK0][main]: Peer-to-peer access cannot be fully enabled.
[HCTR][03:14:18.054][DEBUG][RK0][main]: [device 0] allocating 0.0000 GB, available 30.4832
[HCTR][03:14:18.054][INFO][RK0][main]: Start all2all warmup
[HCTR][03:14:18.054][INFO][RK0][main]: End all2all warmup
[HCTR][03:14:18.055][INFO][RK0][main]: default_emb_vec_value is not specified using default: 0
[HCTR][03:14:18.055][INFO][RK0][main]: default_emb_vec_value is not specified using default: 0
====================================================HPS Create====================================================
[HCTR][03:14:18.055][INFO][RK0][main]: Creating HashMap CPU database backend...
[HCTR][03:14:18.055][DEBUG][RK0][main]: Created blank database backend in local memory!
[HCTR][03:14:18.055][INFO][RK0][main]: Volatile DB: initial cache rate = 1
[HCTR][03:14:18.055][INFO][RK0][main]: Volatile DB: cache missed embeddings = 0
[HCTR][03:14:18.055][DEBUG][RK0][main]: Created raw model loader in local memory!
[HCTR][03:14:18.410][INFO][RK0][main]: Table: hps_et.wdl.sparse_embedding2; cached 2327937 / 2327937 embeddings in volatile database (HashMapBackend); load: 2327937 / 18446744073709551615 (0.00%).
[HCTR][03:14:18.836][INFO][RK0][main]: Table: hps_et.wdl.sparse_embedding1; cached 4169063 / 4169063 embeddings in volatile database (HashMapBackend); load: 4169063 / 18446744073709551615 (0.00%).
[HCTR][03:14:18.837][DEBUG][RK0][main]: Real-time subscribers created!
[HCTR][03:14:18.837][INFO][RK0][main]: Creating embedding cache in device 0.
[HCTR][03:14:18.842][INFO][RK0][main]: Model name: wdl
[HCTR][03:14:18.842][INFO][RK0][main]: Max batch size: 1024
[HCTR][03:14:18.842][INFO][RK0][main]: Fuse embedding tables: False
[HCTR][03:14:18.842][INFO][RK0][main]: Number of embedding tables: 2
[HCTR][03:14:18.842][INFO][RK0][main]: Use GPU embed(10240, 1)
[[4.7124020e-04]
[8.2945243e-02]
[7.2710402e-03]
...
[2.4967238e-02]
[1.2772739e-05]
[4.1527884e-12]]
ding cache: True, cache size percentage: 1.000000
[HCTR][03:14:18.842][INFO][RK0][main]: Embedding cache type: dynamic
[HCTR][03:14:18.842][INFO][RK0][main]: Use I64 input key: True
[HCTR][03:14:18.842][INFO][RK0][main]: Configured cache hit rate threshold: 1.000000
[HCTR][03:14:18.842][INFO][RK0][main]: The size of thread pool: 80
[HCTR][03:14:18.842][INFO][RK0][main]: The size of worker memory pool: 2
[HCTR][03:14:18.842][INFO][RK0][main]: The size of refresh memory pool: 1
[HCTR][03:14:18.842][INFO][RK0][main]: The refresh percentage : 0.000000
[HCTR][03:14:18.856][INFO][RK0][main]: Model name: wdl
[HCTR][03:14:18.856][INFO][RK0][main]: Use mixed precision: False
[HCTR][03:14:18.856][INFO][RK0][main]: Use cuda graph: True
[HCTR][03:14:18.856][INFO][RK0][main]: Max batchsize: 1024
[HCTR][03:14:18.856][INFO][RK0][main]: Use I64 input key: True
[HCTR][03:14:18.856][INFO][RK0][main]: start create embedding for inference
[HCTR][03:14:18.856][INFO][RK0][main]: sparse_input name wide_data
MpiInitService: Initialized!
[HCTR][03:14:18.856][INFO][RK0][main]: sparse_input name deep_data
[HCTR][03:14:18.856][INFO][RK0][main]: create embedding for inference success
[HCTR][03:14:18.857][DEBUG][RK0][main]: [device 0] allocating 0.0016 GB, available 29.8503
[HCTR][03:14:18.857][INFO][RK0][main]: Inference stage skip BinaryCrossEntropyLoss layer, replaced by Sigmoid layer
[HCTR][03:14:18.858][DEBUG][RK0][main]: [device 0] allocating 0.0388 GB, available 29.8035
[HCTR][03:14:20.599][INFO][RK0][main]: Create inference data reader on 1 GPU(s)
[HCTR][03:14:20.599][INFO][RK0][main]: num of DataReader workers: 1
[HCTR][03:14:20.600][DEBUG][RK0][main]: [device 0] allocating 0.0007 GB, available 29.7976
[HCTR][03:14:20.601][INFO][RK0][main]: parquet_eval_max_row_group_size 475000
[HCTR][03:14:20.603][INFO][RK0][main]: Vocabulary size: 7942094
[HCTR][03:14:20.642][INFO][RK0][main]: Inference time for 10 batches: 0.03545