# Copyright 2021 NVIDIA Corporation. All Rights Reserved.
#
# Licensed under the Apache License, Version 2.0 (the "License");
# you may not use this file except in compliance with the License.
# You may obtain a copy of the License at
#
# http://www.apache.org/licenses/LICENSE-2.0
#
# Unless required by applicable law or agreed to in writing, software
# distributed under the License is distributed on an "AS IS" BASIS,
# WITHOUT WARRANTIES OR CONDITIONS OF ANY KIND, either express or implied.
# See the License for the specific language governing permissions and
# limitations under the License.
# ==============================================================================
# Each user is responsible for checking the content of datasets and the
# applicable licenses and determining if suitable for the intended use.
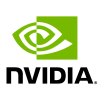
HugeCTR Training and Inference with Remote File System Example
Overview
HugeCTR supports reading Parquet data, loading and saving models from/to remote file systems like HDFS, AWS S3, and GCS. Users can read their data stored in these remote file systems and train with it. And after training, users can choose to dump the trained parameters and optimizer states into these file systems. And during inference, users can read data and load sparse models from remote filesystem. In this example notebook, we are going to demonstrate the end to end procedure of training with HDFS and training plus inference with Amazon AWS S3.
Setup HugeCTR
To setup the environment, refer to HugeCTR Example Notebooks and follow the instructions there before running the following.
Training with HDFS Example
Hadoop is not pre-installe din the Merlin Training Container. To help you build and install HDFS, we provide a script here. Please build and install Hadoop using these two scripts. Make sure you have hadoop installed in your Container by running the following:
!hadoop version
Hadoop 3.3.2
Source code repository https://github.com/apache/hadoop.git -r 0bcb014209e219273cb6fd4152df7df713cbac61
Compiled by root on 2022-07-25T09:53Z
Compiled with protoc 3.7.1
From source with checksum 4b40fff8bb27201ba07b6fa5651217fb
This command was run using /opt/hadoop/share/hadoop/common/hadoop-common-3.3.2.jar
Data Preparation
Users can use the DataSourceParams to setup file system configurations. Currently, we support Local
, HDFS
, S3
, and GCS
.
Firstly, we want to make sure that we have train and validation datasets ready:
!hdfs dfs -ls hdfs://10.19.172.76:9000/dlrm_parquet/train
Found 8 items
-rw-r--r-- 1 root supergroup 112247365 2022-07-27 06:19 hdfs://10.19.172.76:9000/dlrm_parquet/train/gen_0.parquet
-rw-r--r-- 1 root supergroup 112243637 2022-07-27 06:19 hdfs://10.19.172.76:9000/dlrm_parquet/train/gen_1.parquet
-rw-r--r-- 1 root supergroup 112251207 2022-07-27 06:19 hdfs://10.19.172.76:9000/dlrm_parquet/train/gen_2.parquet
-rw-r--r-- 1 root supergroup 112241764 2022-07-27 06:19 hdfs://10.19.172.76:9000/dlrm_parquet/train/gen_3.parquet
-rw-r--r-- 1 root supergroup 112247838 2022-07-27 06:19 hdfs://10.19.172.76:9000/dlrm_parquet/train/gen_4.parquet
-rw-r--r-- 1 root supergroup 112244076 2022-07-27 06:19 hdfs://10.19.172.76:9000/dlrm_parquet/train/gen_5.parquet
-rw-r--r-- 1 root supergroup 112253553 2022-07-27 06:19 hdfs://10.19.172.76:9000/dlrm_parquet/train/gen_6.parquet
-rw-r--r-- 1 root supergroup 112249557 2022-07-27 06:19 hdfs://10.19.172.76:9000/dlrm_parquet/train/gen_7.parquet
!hdfs dfs -ls hdfs://10.19.172.76:9000/dlrm_parquet/val
Found 2 items
-rw-r--r-- 1 root supergroup 112239093 2022-07-27 06:19 hdfs://10.19.172.76:9000/dlrm_parquet/val/gen_0.parquet
-rw-r--r-- 1 root supergroup 112249156 2022-07-27 06:19 hdfs://10.19.172.76:9000/dlrm_parquet/val/gen_1.parquet
Secondly, create file_list.txt and file_list_test.txt
:
!mkdir /dlrm_parquet
!mkdir /dlrm_parquet/train
!mkdir /dlrm_parquet/val
%%writefile /dlrm_parquet/file_list.txt
8
hdfs://10.19.172.76:9000/dlrm_parquet/train/gen_0.parquet
hdfs://10.19.172.76:9000/dlrm_parquet/train/gen_1.parquet
hdfs://10.19.172.76:9000/dlrm_parquet/train/gen_2.parquet
hdfs://10.19.172.76:9000/dlrm_parquet/train/gen_3.parquet
hdfs://10.19.172.76:9000/dlrm_parquet/train/gen_4.parquet
hdfs://10.19.172.76:9000/dlrm_parquet/train/gen_5.parquet
hdfs://10.19.172.76:9000/dlrm_parquet/train/gen_6.parquet
hdfs://10.19.172.76:9000/dlrm_parquet/train/gen_7.parquet
Overwriting /dlrm_parquet/file_list.txt
%%writefile /dlrm_parquet/file_list_test.txt
2
hdfs://10.19.172.76:9000/dlrm_parquet/val/gen_0.parquet
hdfs://10.19.172.76:9000/dlrm_parquet/val/gen_1.parquet
Overwriting /dlrm_parquet/file_list_test.txt
Lastly, create _metadata.json
for both train and validation dataset to specify the feature information of your dataset:
%%writefile /dlrm_parquet/train/_metadata.json
{ "file_stats": [{"file_name": "./dlrm_parquet/train/gen_0.parquet", "num_rows":1000000}, {"file_name": "./dlrm_parquet/train/gen_1.parquet", "num_rows":1000000},
{"file_name": "./dlrm_parquet/train/gen_2.parquet", "num_rows":1000000}, {"file_name": "./dlrm_parquet/train/gen_3.parquet", "num_rows":1000000},
{"file_name": "./dlrm_parquet/train/gen_4.parquet", "num_rows":1000000}, {"file_name": "./dlrm_parquet/train/gen_5.parquet", "num_rows":1000000},
{"file_name": "./dlrm_parquet/train/gen_6.parquet", "num_rows":1000000}, {"file_name": "./dlrm_parquet/train/gen_7.parquet", "num_rows":1000000} ],
"labels": [{"col_name": "label0", "index":0} ],
"conts": [{"col_name": "C1", "index":1}, {"col_name": "C2", "index":2}, {"col_name": "C3", "index":3},
{"col_name": "C4", "index":4}, {"col_name": "C5", "index":5}, {"col_name": "C6", "index":6},
{"col_name": "C7", "index":7}, {"col_name": "C8", "index":8}, {"col_name": "C9", "index":9},
{"col_name": "C10", "index":10}, {"col_name": "C11", "index":11}, {"col_name": "C12", "index":12},
{"col_name": "C13", "index":13} ],
"cats": [{"col_name": "C14", "index":14}, {"col_name": "C15", "index":15}, {"col_name": "C16", "index":16},
{"col_name": "C17", "index":17}, {"col_name": "C18", "index":18}, {"col_name": "C19", "index":19},
{"col_name": "C20", "index":20}, {"col_name": "C21", "index":21}, {"col_name": "C22", "index":22},
{"col_name": "C23", "index":23}, {"col_name": "C24", "index":24}, {"col_name": "C25", "index":25},
{"col_name": "C26", "index":26}, {"col_name": "C27", "index":27}, {"col_name": "C28", "index":28},
{"col_name": "C29", "index":29}, {"col_name": "C30", "index":30}, {"col_name": "C31", "index":31},
{"col_name": "C32", "index":32}, {"col_name": "C33", "index":33}, {"col_name": "C34", "index":34},
{"col_name": "C35", "index":35}, {"col_name": "C36", "index":36}, {"col_name": "C37", "index":37},
{"col_name": "C38", "index":38}, {"col_name": "C39", "index":39} ] }
Writing /dlrm_parquet/train/_metadata.json
%%writefile /dlrm_parquet/val/_metadata.json
{ "file_stats": [{"file_name": "./dlrm_parquet/val/gen_0.parquet", "num_rows":1000000},
{"file_name": "./dlrm_parquet/val/gen_1.parquet", "num_rows":1000000} ],
"labels": [{"col_name": "label0", "index":0} ],
"conts": [{"col_name": "C1", "index":1}, {"col_name": "C2", "index":2}, {"col_name": "C3", "index":3},
{"col_name": "C4", "index":4}, {"col_name": "C5", "index":5}, {"col_name": "C6", "index":6},
{"col_name": "C7", "index":7}, {"col_name": "C8", "index":8}, {"col_name": "C9", "index":9},
{"col_name": "C10", "index":10}, {"col_name": "C11", "index":11}, {"col_name": "C12", "index":12},
{"col_name": "C13", "index":13} ],
"cats": [{"col_name": "C14", "index":14}, {"col_name": "C15", "index":15}, {"col_name": "C16", "index":16},
{"col_name": "C17", "index":17}, {"col_name": "C18", "index":18}, {"col_name": "C19", "index":19},
{"col_name": "C20", "index":20}, {"col_name": "C21", "index":21}, {"col_name": "C22", "index":22},
{"col_name": "C23", "index":23}, {"col_name": "C24", "index":24}, {"col_name": "C25", "index":25},
{"col_name": "C26", "index":26}, {"col_name": "C27", "index":27}, {"col_name": "C28", "index":28},
{"col_name": "C29", "index":29}, {"col_name": "C30", "index":30}, {"col_name": "C31", "index":31},
{"col_name": "C32", "index":32}, {"col_name": "C33", "index":33}, {"col_name": "C34", "index":34},
{"col_name": "C35", "index":35}, {"col_name": "C36", "index":36}, {"col_name": "C37", "index":37},
{"col_name": "C38", "index":38}, {"col_name": "C39", "index":39} ] }
Writing /dlrm_parquet/val/_metadata.json
Training a DLRM model
Important APIs used in the following script:
We use the DataSourceParams to define the remote file system to read data from
In DataReaderParams, we specify the
DataSourceParams
.In fit() method, we specify HDFS path in the
snapshot_prefix
parameters to dump trained models to HDFS.
%%writefile train_with_hdfs.py
import hugectr
from mpi4py import MPI
from hugectr.data import DataSourceParams
# Create a file system configuration
data_source_params = DataSourceParams(
source = hugectr.DataSourceType_t.HDFS, #use HDFS
server = '10.19.172.76', #your HDFS namenode IP
port = 9000, #your HDFS namenode port
)
# DLRM train
solver = hugectr.CreateSolver(max_eval_batches = 1280,
batchsize_eval = 1024,
batchsize = 1024,
lr = 0.01,
vvgpu = [[1]],
i64_input_key = True,
use_mixed_precision = False,
repeat_dataset = True,
use_cuda_graph = False)
reader = hugectr.DataReaderParams(data_reader_type = hugectr.DataReaderType_t.Parquet,
source = ["/dlrm_parquet/file_list.txt"],
eval_source = "/dlrm_parquet/file_list_test.txt",
slot_size_array = [405274, 72550, 55008, 222734, 316071, 156265, 220243, 200179, 234566, 335625, 278726, 263070, 312542, 203773, 145859, 117421, 78140, 3648, 156308, 94562, 357703, 386976, 238046, 230917, 292, 156382],
data_source_params = data_source_params, #file system config for data reading
check_type = hugectr.Check_t.Non)
optimizer = hugectr.CreateOptimizer(optimizer_type = hugectr.Optimizer_t.SGD,
update_type = hugectr.Update_t.Local,
atomic_update = True)
model = hugectr.Model(solver, reader, optimizer)
model.add(hugectr.Input(label_dim = 1, label_name = "label",
dense_dim = 13, dense_name = "dense",
data_reader_sparse_param_array =
[hugectr.DataReaderSparseParam("data1", 1, True, 26)]))
model.add(hugectr.SparseEmbedding(embedding_type = hugectr.Embedding_t.DistributedSlotSparseEmbeddingHash,
workspace_size_per_gpu_in_mb = 10720,
embedding_vec_size = 128,
combiner = "sum",
sparse_embedding_name = "sparse_embedding1",
bottom_name = "data1",
optimizer = optimizer))
model.add(hugectr.DenseLayer(layer_type = hugectr.Layer_t.InnerProduct,
bottom_names = ["dense"],
top_names = ["fc1"],
num_output=512))
model.add(hugectr.DenseLayer(layer_type = hugectr.Layer_t.ReLU,
bottom_names = ["fc1"],
top_names = ["relu1"]))
model.add(hugectr.DenseLayer(layer_type = hugectr.Layer_t.InnerProduct,
bottom_names = ["relu1"],
top_names = ["fc2"],
num_output=256))
model.add(hugectr.DenseLayer(layer_type = hugectr.Layer_t.ReLU,
bottom_names = ["fc2"],
top_names = ["relu2"]))
model.add(hugectr.DenseLayer(layer_type = hugectr.Layer_t.InnerProduct,
bottom_names = ["relu2"],
top_names = ["fc3"],
num_output=128))
model.add(hugectr.DenseLayer(layer_type = hugectr.Layer_t.ReLU,
bottom_names = ["fc3"],
top_names = ["relu3"]))
model.add(hugectr.DenseLayer(layer_type = hugectr.Layer_t.Interaction,
bottom_names = ["relu3","sparse_embedding1"],
top_names = ["interaction1"]))
model.add(hugectr.DenseLayer(layer_type = hugectr.Layer_t.InnerProduct,
bottom_names = ["interaction1"],
top_names = ["fc4"],
num_output=1024))
model.add(hugectr.DenseLayer(layer_type = hugectr.Layer_t.ReLU,
bottom_names = ["fc4"],
top_names = ["relu4"]))
model.add(hugectr.DenseLayer(layer_type = hugectr.Layer_t.InnerProduct,
bottom_names = ["relu4"],
top_names = ["fc5"],
num_output=1024))
model.add(hugectr.DenseLayer(layer_type = hugectr.Layer_t.ReLU,
bottom_names = ["fc5"],
top_names = ["relu5"]))
model.add(hugectr.DenseLayer(layer_type = hugectr.Layer_t.InnerProduct,
bottom_names = ["relu5"],
top_names = ["fc6"],
num_output=512))
model.add(hugectr.DenseLayer(layer_type = hugectr.Layer_t.ReLU,
bottom_names = ["fc6"],
top_names = ["relu6"]))
model.add(hugectr.DenseLayer(layer_type = hugectr.Layer_t.InnerProduct,
bottom_names = ["relu6"],
top_names = ["fc7"],
num_output=256))
model.add(hugectr.DenseLayer(layer_type = hugectr.Layer_t.ReLU,
bottom_names = ["fc7"],
top_names = ["relu7"]))
model.add(hugectr.DenseLayer(layer_type = hugectr.Layer_t.InnerProduct,
bottom_names = ["relu7"],
top_names = ["fc8"],
num_output=1))
model.add(hugectr.DenseLayer(layer_type = hugectr.Layer_t.BinaryCrossEntropyLoss,
bottom_names = ["fc8", "label"],
top_names = ["loss"]))
model.compile()
model.summary()
model.fit(max_iter = 2020, display = 200, eval_interval = 1000, snapshot = 2000, snapshot_prefix = "hdfs://10.19.172.76:9000/model/dlrm/")
Overwriting train_with_hdfs.py
!python train_with_hdfs.py
HugeCTR Version: 3.8
====================================================Model Init=====================================================
[HCTR][07:51:52.502][WARNING][RK0][main]: The model name is not specified when creating the solver.
[HCTR][07:51:52.502][INFO][RK0][main]: Global seed is 3218787045
[HCTR][07:51:52.505][INFO][RK0][main]: Device to NUMA mapping:
GPU 1 -> node 0
[HCTR][07:51:55.607][WARNING][RK0][main]: Peer-to-peer access cannot be fully enabled.
[HCTR][07:51:55.607][INFO][RK0][main]: Start all2all warmup
[HCTR][07:51:55.609][INFO][RK0][main]: End all2all warmup
[HCTR][07:51:56.529][INFO][RK0][main]: Using All-reduce algorithm: NCCL
[HCTR][07:51:56.530][INFO][RK0][main]: Device 1: NVIDIA A10
[HCTR][07:51:56.531][INFO][RK0][main]: num of DataReader workers for train: 1
[HCTR][07:51:56.531][INFO][RK0][main]: num of DataReader workers for eval: 1
[HCTR][07:51:57.695][INFO][RK0][main]: Using Hadoop Cluster 10.19.172.76:9000
[HCTR][07:51:57.740][INFO][RK0][main]: Using Hadoop Cluster 10.19.172.76:9000
[HCTR][07:51:57.740][INFO][RK0][main]: Vocabulary size: 5242880
[HCTR][07:51:57.741][INFO][RK0][main]: max_vocabulary_size_per_gpu_=21954560
[HCTR][07:51:57.755][INFO][RK0][main]: Graph analysis to resolve tensor dependency
===================================================Model Compile===================================================
[HCTR][07:52:04.336][INFO][RK0][main]: gpu0 start to init embedding
[HCTR][07:52:04.411][INFO][RK0][main]: gpu0 init embedding done
[HCTR][07:52:04.413][INFO][RK0][main]: Starting AUC NCCL warm-up
[HCTR][07:52:04.415][INFO][RK0][main]: Warm-up done
===================================================Model Summary===================================================
[HCTR][07:52:04.415][INFO][RK0][main]: label Dense Sparse
label dense data1
(None, 1) (None, 13)
——————————————————————————————————————————————————————————————————————————————————————————————————————————————————
Layer Type Input Name Output Name Output Shape
——————————————————————————————————————————————————————————————————————————————————————————————————————————————————
DistributedSlotSparseEmbeddingHash data1 sparse_embedding1 (None, 26, 128)
------------------------------------------------------------------------------------------------------------------
InnerProduct dense fc1 (None, 512)
------------------------------------------------------------------------------------------------------------------
ReLU fc1 relu1 (None, 512)
------------------------------------------------------------------------------------------------------------------
InnerProduct relu1 fc2 (None, 256)
------------------------------------------------------------------------------------------------------------------
ReLU fc2 relu2 (None, 256)
------------------------------------------------------------------------------------------------------------------
InnerProduct relu2 fc3 (None, 128)
------------------------------------------------------------------------------------------------------------------
ReLU fc3 relu3 (None, 128)
------------------------------------------------------------------------------------------------------------------
Interaction relu3 interaction1 (None, 480)
sparse_embedding1
------------------------------------------------------------------------------------------------------------------
InnerProduct interaction1 fc4 (None, 1024)
------------------------------------------------------------------------------------------------------------------
ReLU fc4 relu4 (None, 1024)
------------------------------------------------------------------------------------------------------------------
InnerProduct relu4 fc5 (None, 1024)
------------------------------------------------------------------------------------------------------------------
ReLU fc5 relu5 (None, 1024)
------------------------------------------------------------------------------------------------------------------
InnerProduct relu5 fc6 (None, 512)
------------------------------------------------------------------------------------------------------------------
ReLU fc6 relu6 (None, 512)
------------------------------------------------------------------------------------------------------------------
InnerProduct relu6 fc7 (None, 256)
------------------------------------------------------------------------------------------------------------------
ReLU fc7 relu7 (None, 256)
------------------------------------------------------------------------------------------------------------------
InnerProduct relu7 fc8 (None, 1)
------------------------------------------------------------------------------------------------------------------
BinaryCrossEntropyLoss fc8 loss
label
------------------------------------------------------------------------------------------------------------------
=====================================================Model Fit=====================================================
[HCTR][07:52:04.415][INFO][RK0][main]: Use non-epoch mode with number of iterations: 2020
[HCTR][07:52:04.415][INFO][RK0][main]: Training batchsize: 1024, evaluation batchsize: 1024
[HCTR][07:52:04.415][INFO][RK0][main]: Evaluation interval: 1000, snapshot interval: 2000
[HCTR][07:52:04.415][INFO][RK0][main]: Dense network trainable: True
[HCTR][07:52:04.415][INFO][RK0][main]: Sparse embedding sparse_embedding1 trainable: True
[HCTR][07:52:04.415][INFO][RK0][main]: Use mixed precision: False, scaler: 1.000000, use cuda graph: False
[HCTR][07:52:04.415][INFO][RK0][main]: lr: 0.010000, warmup_steps: 1, end_lr: 0.000000
[HCTR][07:52:04.415][INFO][RK0][main]: decay_start: 0, decay_steps: 1, decay_power: 2.000000
[HCTR][07:52:04.415][INFO][RK0][main]: Training source file: /dlrm_parquet/file_list.txt
[HCTR][07:52:04.415][INFO][RK0][main]: Evaluation source file: /dlrm_parquet/file_list_test.txt
[HCTR][07:52:05.134][INFO][RK0][main]: Iter: 200 Time(200 iters): 0.716815s Loss: 0.69327 lr:0.01
[HCTR][07:52:05.856][INFO][RK0][main]: Iter: 400 Time(200 iters): 0.719486s Loss: 0.693207 lr:0.01
[HCTR][07:52:06.608][INFO][RK0][main]: Iter: 600 Time(200 iters): 0.750294s Loss: 0.693568 lr:0.01
[HCTR][07:52:07.331][INFO][RK0][main]: Iter: 800 Time(200 iters): 0.721128s Loss: 0.693352 lr:0.01
[HCTR][07:52:09.118][INFO][RK0][main]: Iter: 1000 Time(200 iters): 1.78435s Loss: 0.693352 lr:0.01
[HCTR][07:52:11.667][INFO][RK0][main]: Evaluation, AUC: 0.499891
[HCTR][07:52:11.668][INFO][RK0][main]: Eval Time for 1280 iters: 2.5486s
[HCTR][07:52:12.393][INFO][RK0][main]: Iter: 1200 Time(200 iters): 3.2728s Loss: 0.693178 lr:0.01
[HCTR][07:52:13.116][INFO][RK0][main]: Iter: 1400 Time(200 iters): 0.720984s Loss: 0.693292 lr:0.01
[HCTR][07:52:13.875][INFO][RK0][main]: Iter: 1600 Time(200 iters): 0.756448s Loss: 0.693053 lr:0.01
[HCTR][07:52:14.603][INFO][RK0][main]: Iter: 1800 Time(200 iters): 0.725832s Loss: 0.693433 lr:0.01
[HCTR][07:52:16.382][INFO][RK0][main]: Iter: 2000 Time(200 iters): 1.77763s Loss: 0.693193 lr:0.01
[HCTR][07:52:18.959][INFO][RK0][main]: Evaluation, AUC: 0.500092
[HCTR][07:52:18.959][INFO][RK0][main]: Eval Time for 1280 iters: 2.57548s
[HCTR][07:52:19.575][INFO][RK0][main]: Rank0: Write hash table to file
[HDFS][INFO]: Write to HDFS /model/dlrm/0_sparse_2000.model/key successfully!
[HDFS][INFO]: Write to HDFS /model/dlrm/0_sparse_2000.model/emb_vector successfully!
[HCTR][07:52:31.132][INFO][RK0][main]: Dumping sparse weights to files, successful
[HCTR][07:52:31.132][INFO][RK0][main]: Dumping sparse optimzer states to files, successful
[HDFS][INFO]: Write to HDFS /model/dlrm/_dense_2000.model successfully!
[HCTR][07:52:31.307][INFO][RK0][main]: Dumping dense weights to HDFS, successful
[HDFS][INFO]: Write to HDFS /model/dlrm/_opt_dense_2000.model successfully!
[HCTR][07:52:31.365][INFO][RK0][main]: Dumping dense optimizer states to HDFS, successful
[HCTR][07:52:31.430][INFO][RK0][main]: Finish 2020 iterations with batchsize: 1024 in 27.02s.
Check that our model files are saved in HDFS:
!hdfs dfs -ls hdfs://10.19.172.76:9000/model/dlrm
Found 3 items
drwxr-xr-x - root supergroup 0 2022-07-27 07:52 hdfs://10.19.172.76:9000/model/dlrm/0_sparse_2000.model
-rw-r--r-- 3 root supergroup 9479684 2022-07-27 07:52 hdfs://10.19.172.76:9000/model/dlrm/_dense_2000.model
-rw-r--r-- 3 root supergroup 0 2022-07-27 07:52 hdfs://10.19.172.76:9000/model/dlrm/_opt_dense_2000.model
Training a DCN model with AWS S3
Before you start: Please note that AWS S3 SDKs are NOT preinstalled in the NGC docker. To use S3 related functionalites, please do the following steps to customize the building of HugeCTR:
git clone https://github.com/NVIDIA/HugeCTR.git
cd HugeCTR
git submodule update –init –recursive
mkdir -p build && cd build
cmake -DCMAKE_BUILD_TYPE=Release -DSM=70 -DENABLE_S3=ON … #ENABLE_S3 option will install AWS S3 SDKs for you.
make -j && make install
Data preparation
Create file_list.txt and file_list_test.txt
:
!mkdir -p /hugectr-io-test/data/dcn_parquet/train
!mkdir -p /hugectr-io-test/data/dcn_parquet/val
%%writefile /hugectr-io-test/data/dcn_parquet/file_list.txt
16
s3://hugectr-io-test/data/dcn_parquet/train/gen_0.parquet
s3://hugectr-io-test/data/dcn_parquet/train/gen_1.parquet
s3://hugectr-io-test/data/dcn_parquet/train/gen_2.parquet
s3://hugectr-io-test/data/dcn_parquet/train/gen_3.parquet
s3://hugectr-io-test/data/dcn_parquet/train/gen_4.parquet
s3://hugectr-io-test/data/dcn_parquet/train/gen_5.parquet
s3://hugectr-io-test/data/dcn_parquet/train/gen_6.parquet
s3://hugectr-io-test/data/dcn_parquet/train/gen_7.parquet
s3://hugectr-io-test/data/dcn_parquet/train/gen_8.parquet
s3://hugectr-io-test/data/dcn_parquet/train/gen_9.parquet
s3://hugectr-io-test/data/dcn_parquet/train/gen_10.parquet
s3://hugectr-io-test/data/dcn_parquet/train/gen_11.parquet
s3://hugectr-io-test/data/dcn_parquet/train/gen_12.parquet
s3://hugectr-io-test/data/dcn_parquet/train/gen_13.parquet
s3://hugectr-io-test/data/dcn_parquet/train/gen_14.parquet
s3://hugectr-io-test/data/dcn_parquet/train/gen_15.parquet
Writing /hugectr-io-test/data/dcn_parquet/file_list.txt
%%writefile /hugectr-io-test/data/dcn_parquet/file_list_test.txt
4
s3://hugectr-io-test/data/dcn_parquet/val/gen_0.parquet
s3://hugectr-io-test/data/dcn_parquet/val/gen_1.parquet
s3://hugectr-io-test/data/dcn_parquet/val/gen_2.parquet
s3://hugectr-io-test/data/dcn_parquet/val/gen_3.parquet
Writing /hugectr-io-test/data/dcn_parquet/file_list_test.txt
%%writefile /hugectr-io-test/data/dcn_parquet/train/_metadata.json
{ "file_stats": [{"file_name": "s3://hugectr-io-test/data/dcn_parquet/train/gen_0.parquet", "num_rows":40960}, {"file_name": "s3://hugectr-io-test/data/dcn_parquet/train/gen_1.parquet", "num_rows":40960},
{"file_name": "s3://hugectr-io-test/data/dcn_parquet/train/gen_2.parquet", "num_rows":40960}, {"file_name": "s3://hugectr-io-test/data/dcn_parquet/train/gen_3.parquet", "num_rows":40960},
{"file_name": "s3://hugectr-io-test/data/dcn_parquet/train/gen_4.parquet", "num_rows":40960}, {"file_name": "s3://hugectr-io-test/data/dcn_parquet/train/gen_5.parquet", "num_rows":40960},
{"file_name": "s3://hugectr-io-test/data/dcn_parquet/train/gen_6.parquet", "num_rows":40960}, {"file_name": "s3://hugectr-io-test/data/dcn_parquet/train/gen_7.parquet", "num_rows":40960},
{"file_name": "s3://hugectr-io-test/data/dcn_parquet/train/gen_8.parquet", "num_rows":40960}, {"file_name": "s3://hugectr-io-test/data/dcn_parquet/train/gen_9.parquet", "num_rows":40960},
{"file_name": "s3://hugectr-io-test/data/dcn_parquet/train/gen_10.parquet", "num_rows":40960}, {"file_name": "s3://hugectr-io-test/data/dcn_parquet/train/gen_11.parquet", "num_rows":40960},
{"file_name": "s3://hugectr-io-test/data/dcn_parquet/train/gen_12.parquet", "num_rows":40960}, {"file_name": "s3://hugectr-io-test/data/dcn_parquet/train/gen_13.parquet", "num_rows":40960},
{"file_name": "s3://hugectr-io-test/data/dcn_parquet/train/gen_14.parquet", "num_rows":40960}, {"file_name": "s3://hugectr-io-test/data/dcn_parquet/train/gen_15.parquet", "num_rows":40960}],
"labels": [{"col_name": "label0", "index":0} ],
"conts": [{"col_name": "C1", "index":1}, {"col_name": "C2", "index":2}, {"col_name": "C3", "index":3}, {"col_name": "C4", "index":4}, {"col_name": "C5", "index":5}, {"col_name": "C6", "index":6},
{"col_name": "C7", "index":7}, {"col_name": "C8", "index":8}, {"col_name": "C9", "index":9}, {"col_name": "C10", "index":10}, {"col_name": "C11", "index":11}, {"col_name": "C12", "index":12},
{"col_name": "C13", "index":13} ],
"cats": [{"col_name": "C14", "index":14}, {"col_name": "C15", "index":15}, {"col_name": "C16", "index":16}, {"col_name": "C17", "index":17}, {"col_name": "C18", "index":18},
{"col_name": "C19", "index":19}, {"col_name": "C20", "index":20}, {"col_name": "C21", "index":21}, {"col_name": "C22", "index":22}, {"col_name": "C23", "index":23},
{"col_name": "C24", "index":24}, {"col_name": "C25", "index":25}, {"col_name": "C26", "index":26}, {"col_name": "C27", "index":27}, {"col_name": "C28", "index":28},
{"col_name": "C29", "index":29}, {"col_name": "C30", "index":30}, {"col_name": "C31", "index":31}, {"col_name": "C32", "index":32}, {"col_name": "C33", "index":33},
{"col_name": "C34", "index":34}, {"col_name": "C35", "index":35}, {"col_name": "C36", "index":36}, {"col_name": "C37", "index":37}, {"col_name": "C38", "index":38}, {"col_name": "C39", "index":39} ] }
Writing /hugectr-io-test/data/dcn_parquet/train/_metadata.json
%%writefile /hugectr-io-test/data/dcn_parquet/val/_metadata.json
{ "file_stats": [{"file_name": "s3://hugectr-io-test/data/dcn_parquet/val/gen_0.parquet", "num_rows":40960},
{"file_name": "s3://hugectr-io-test/data/dcn_parquet/val/gen_1.parquet", "num_rows":40960},
{"file_name": "s3://hugectr-io-test/data/dcn_parquet/val/gen_2.parquet", "num_rows":40960},
{"file_name": "s3://hugectr-io-test/data/dcn_parquet/val/gen_3.parquet", "num_rows":40960}],
"labels": [{"col_name": "label0", "index":0} ],
"conts": [{"col_name": "C1", "index":1}, {"col_name": "C2", "index":2}, {"col_name": "C3", "index":3}, {"col_name": "C4", "index":4}, {"col_name": "C5", "index":5}, {"col_name": "C6", "index":6},
{"col_name": "C7", "index":7}, {"col_name": "C8", "index":8}, {"col_name": "C9", "index":9}, {"col_name": "C10", "index":10}, {"col_name": "C11", "index":11}, {"col_name": "C12", "index":12},
{"col_name": "C13", "index":13} ],
"cats": [{"col_name": "C14", "index":14}, {"col_name": "C15", "index":15}, {"col_name": "C16", "index":16}, {"col_name": "C17", "index":17}, {"col_name": "C18", "index":18},
{"col_name": "C19", "index":19}, {"col_name": "C20", "index":20}, {"col_name": "C21", "index":21}, {"col_name": "C22", "index":22}, {"col_name": "C23", "index":23},
{"col_name": "C24", "index":24}, {"col_name": "C25", "index":25}, {"col_name": "C26", "index":26}, {"col_name": "C27", "index":27}, {"col_name": "C28", "index":28},
{"col_name": "C29", "index":29}, {"col_name": "C30", "index":30}, {"col_name": "C31", "index":31}, {"col_name": "C32", "index":32}, {"col_name": "C33", "index":33},
{"col_name": "C34", "index":34}, {"col_name": "C35", "index":35}, {"col_name": "C36", "index":36}, {"col_name": "C37", "index":37}, {"col_name": "C38", "index":38}, {"col_name": "C39", "index":39} ] }
Writing /hugectr-io-test/data/dcn_parquet/val/_metadata.json
Training
Important APIs used in the following script:
We use the DataSourceParams to define the remote file system to read data from, in this case, S3.
In DataReaderParams, we specify the
DataSourceParams
.In fit() method, we specify S3 path in the
snapshot_prefix
parameters to dump trained models to S3.
%%writefile train_with_s3.py
import hugectr
from mpi4py import MPI
from hugectr.data import DataSourceParams
# Create a file system configuration for data reading
data_source_params = DataSourceParams(
source = hugectr.FileSystemType_t.S3, #use AWS S3
server = 'us-east-1', #your AWS region
port = 9000, #with be ignored
)
solver = hugectr.CreateSolver(
max_eval_batches=1280,
batchsize_eval=1024,
batchsize=1024,
lr=0.001,
vvgpu=[[0]],
i64_input_key=True,
repeat_dataset=True,
)
reader = hugectr.DataReaderParams(
data_reader_type=hugectr.DataReaderType_t.Parquet,
source=["/hugectr-io-test/data/dcn_parquet/file_list.txt"],
eval_source="/hugectr-io-test/data/dcn_parquet/file_list_test.txt",
slot_size_array=[39884,39043,17289,7420,20263,3,7120,1543,39884,39043,17289,7420,20263,3,7120,1543,63,63,39884,39043,17289,7420,20263,3,7120,1543],
data_source_params=data_source_params, # Using the S3 configurations
check_type=hugectr.Check_t.Non,
)
optimizer = hugectr.CreateOptimizer(optimizer_type=hugectr.Optimizer_t.SGD)
model = hugectr.Model(solver, reader, optimizer)
model.add(
hugectr.Input(
label_dim=1,
label_name="label",
dense_dim=13,
dense_name="dense",
data_reader_sparse_param_array=[
hugectr.DataReaderSparseParam("data1", 1, True, 26)
],
)
)
model.add(
hugectr.SparseEmbedding(
embedding_type=hugectr.Embedding_t.DistributedSlotSparseEmbeddingHash,
workspace_size_per_gpu_in_mb=150,
embedding_vec_size=16,
combiner="sum",
sparse_embedding_name="sparse_embedding1",
bottom_name="data1",
optimizer=optimizer,
)
)
model.add(
hugectr.DenseLayer(
layer_type=hugectr.Layer_t.Reshape,
bottom_names=["sparse_embedding1"],
top_names=["reshape1"],
leading_dim=416,
)
)
model.add(
hugectr.DenseLayer(
layer_type=hugectr.Layer_t.Concat, bottom_names=["reshape1", "dense"], top_names=["concat1"]
)
)
model.add(
hugectr.DenseLayer(
layer_type=hugectr.Layer_t.Slice,
bottom_names=["concat1"],
top_names=["slice11", "slice12"],
ranges=[(0, 429), (0, 429)],
)
)
model.add(
hugectr.DenseLayer(
layer_type=hugectr.Layer_t.MultiCross,
bottom_names=["slice11"],
top_names=["multicross1"],
num_layers=6,
)
)
model.add(
hugectr.DenseLayer(
layer_type=hugectr.Layer_t.InnerProduct,
bottom_names=["slice12"],
top_names=["fc1"],
num_output=1024,
)
)
model.add(
hugectr.DenseLayer(layer_type=hugectr.Layer_t.ReLU, bottom_names=["fc1"], top_names=["relu1"])
)
model.add(
hugectr.DenseLayer(
layer_type=hugectr.Layer_t.Dropout,
bottom_names=["relu1"],
top_names=["dropout1"],
dropout_rate=0.5,
)
)
model.add(
hugectr.DenseLayer(
layer_type=hugectr.Layer_t.Concat,
bottom_names=["dropout1", "multicross1"],
top_names=["concat2"],
)
)
model.add(
hugectr.DenseLayer(
layer_type=hugectr.Layer_t.InnerProduct,
bottom_names=["concat2"],
top_names=["fc2"],
num_output=1,
)
)
model.add(
hugectr.DenseLayer(
layer_type=hugectr.Layer_t.BinaryCrossEntropyLoss,
bottom_names=["fc2", "label"],
top_names=["loss"],
)
)
model.compile()
model.summary()
model.fit(max_iter = 1100, display = 100, eval_interval = 500, snapshot = 1000, snapshot_prefix = "https://s3.us-east-1.amazonaws.com/hugectr-io-test/pipeline_test/dcn_model/")
model.graph_to_json(graph_config_file = "dcn.json")
Overwriting train_with_s3.py
!python train_with_s3.py
HugeCTR Version: 4.1
====================================================Model Init=====================================================
[HCTR][06:54:55.819][WARNING][RK0][main]: The model name is not specified when creating the solver.
[HCTR][06:54:55.819][INFO][RK0][main]: Global seed is 569406237
[HCTR][06:54:55.822][INFO][RK0][main]: Device to NUMA mapping:
GPU 0 -> node 0
[HCTR][06:54:57.710][WARNING][RK0][main]: Peer-to-peer access cannot be fully enabled.
[HCTR][06:54:57.710][INFO][RK0][main]: Start all2all warmup
[HCTR][06:54:57.710][INFO][RK0][main]: End all2all warmup
[HCTR][06:54:57.711][INFO][RK0][main]: Using All-reduce algorithm: NCCL
[HCTR][06:54:57.712][INFO][RK0][main]: Device 0: Tesla V100-SXM2-32GB
[HCTR][06:54:57.713][INFO][RK0][main]: num of DataReader workers for train: 1
[HCTR][06:54:57.713][INFO][RK0][main]: num of DataReader workers for eval: 1
[HCTR][06:54:57.714][INFO][RK0][main]: Using S3 file system backend.
[HCTR][06:54:59.762][INFO][RK0][main]: Using S3 file system backend.
[HCTR][06:55:01.777][INFO][RK0][main]: Vocabulary size: 397821
[HCTR][06:55:01.777][INFO][RK0][main]: max_vocabulary_size_per_gpu_=2457600
[HCTR][06:55:01.780][INFO][RK0][main]: Graph analysis to resolve tensor dependency
===================================================Model Compile===================================================
[HCTR][06:55:03.407][INFO][RK0][main]: gpu0 start to init embedding
[HCTR][06:55:03.408][INFO][RK0][main]: gpu0 init embedding done
[HCTR][06:55:03.409][INFO][RK0][main]: Starting AUC NCCL warm-up
[HCTR][06:55:03.411][INFO][RK0][main]: Warm-up done
===================================================Model Summary===================================================
[HCTR][06:55:03.412][INFO][RK0][main]: Model structure on each GPU
Label Dense Sparse
label dense data1
(1024,1) (1024,13)
——————————————————————————————————————————————————————————————————————————————————————————————————————————————————
Layer Type Input Name Output Name Output Shape
——————————————————————————————————————————————————————————————————————————————————————————————————————————————————
DistributedSlotSparseEmbeddingHash data1 sparse_embedding1 (1024,26,16)
------------------------------------------------------------------------------------------------------------------
Reshape sparse_embedding1 reshape1 (1024,416)
------------------------------------------------------------------------------------------------------------------
Concat reshape1 concat1 (1024,429)
dense
------------------------------------------------------------------------------------------------------------------
Slice concat1 slice11 (1024,429)
slice12 (1024,429)
------------------------------------------------------------------------------------------------------------------
MultiCross slice11 multicross1 (1024,429)
------------------------------------------------------------------------------------------------------------------
InnerProduct slice12 fc1 (1024,1024)
------------------------------------------------------------------------------------------------------------------
ReLU fc1 relu1 (1024,1024)
------------------------------------------------------------------------------------------------------------------
Dropout relu1 dropout1 (1024,1024)
------------------------------------------------------------------------------------------------------------------
Concat dropout1 concat2 (1024,1453)
multicross1
------------------------------------------------------------------------------------------------------------------
InnerProduct concat2 fc2 (1024,1)
------------------------------------------------------------------------------------------------------------------
BinaryCrossEntropyLoss fc2 loss
label
------------------------------------------------------------------------------------------------------------------
=====================================================Model Fit=====================================================
[HCTR][06:55:03.412][INFO][RK0][main]: Use non-epoch mode with number of iterations: 1100
[HCTR][06:55:03.412][INFO][RK0][main]: Training batchsize: 1024, evaluation batchsize: 1024
[HCTR][06:55:03.412][INFO][RK0][main]: Evaluation interval: 500, snapshot interval: 1000
[HCTR][06:55:03.412][INFO][RK0][main]: Dense network trainable: True
[HCTR][06:55:03.412][INFO][RK0][main]: Sparse embedding sparse_embedding1 trainable: True
[HCTR][06:55:03.412][INFO][RK0][main]: Use mixed precision: False, scaler: 1.000000, use cuda graph: True
[HCTR][06:55:03.412][INFO][RK0][main]: lr: 0.001000, warmup_steps: 1, end_lr: 0.000000
[HCTR][06:55:03.412][INFO][RK0][main]: decay_start: 0, decay_steps: 1, decay_power: 2.000000
[HCTR][06:55:03.412][INFO][RK0][main]: Training source file: /hugectr-io-test/data/dcn_parquet/file_list.txt
[HCTR][06:55:03.412][INFO][RK0][main]: Evaluation source file: /hugectr-io-test/data/dcn_parquet/file_list_test.txt
[HCTR][06:55:04.668][INFO][RK0][main]: Iter: 100 Time(100 iters): 1.25574s Loss: 0.712926 lr:0.001
[HCTR][06:55:06.839][INFO][RK0][main]: Iter: 200 Time(100 iters): 2.16987s Loss: 0.701584 lr:0.001
[HCTR][06:55:08.066][INFO][RK0][main]: Iter: 300 Time(100 iters): 1.22653s Loss: 0.696012 lr:0.001
[HCTR][06:55:10.229][INFO][RK0][main]: Iter: 400 Time(100 iters): 2.16121s Loss: 0.698167 lr:0.001
[HCTR][06:55:11.653][INFO][RK0][main]: Iter: 500 Time(100 iters): 1.42367s Loss: 0.695641 lr:0.001
[HCTR][06:55:29.727][INFO][RK0][main]: Evaluation, AUC: 0.500979
[HCTR][06:55:29.727][INFO][RK0][main]: Eval Time for 1280 iters: 18.0735s
[HCTR][06:55:32.311][INFO][RK0][main]: Iter: 600 Time(100 iters): 20.6575s Loss: 0.696028 lr:0.001
[HCTR][06:55:33.349][INFO][RK0][main]: Iter: 700 Time(100 iters): 1.03696s Loss: 0.693602 lr:0.001
[HCTR][06:55:35.089][INFO][RK0][main]: Iter: 800 Time(100 iters): 1.73903s Loss: 0.693618 lr:0.001
[HCTR][06:55:36.191][INFO][RK0][main]: Iter: 900 Time(100 iters): 1.10101s Loss: 0.696232 lr:0.001
[HCTR][06:55:37.789][INFO][RK0][main]: Iter: 1000 Time(100 iters): 1.59704s Loss: 0.693168 lr:0.001
[HCTR][06:55:53.378][INFO][RK0][main]: Evaluation, AUC: 0.50103
[HCTR][06:55:53.378][INFO][RK0][main]: Eval Time for 1280 iters: 15.5882s
[HCTR][06:55:53.378][INFO][RK0][main]: Using S3 file system backend.
[HCTR][06:55:55.410][INFO][RK0][main]: Rank0: Write hash table to file
[HCTR][06:55:56.473][DEBUG][RK0][main]: Successfully write to AWS S3 location: https://s3.us-east-1.amazonaws.com/hugectr-io-test/pipeline_test/dcn_model/0_sparse_1000.model/key
[HCTR][06:55:57.348][DEBUG][RK0][main]: Successfully write to AWS S3 location: https://s3.us-east-1.amazonaws.com/hugectr-io-test/pipeline_test/dcn_model/0_sparse_1000.model/emb_vector
[HCTR][06:55:57.360][INFO][RK0][main]: Dumping sparse weights to files, successful
[HCTR][06:55:57.360][INFO][RK0][main]: Dumping sparse optimzer states to files, successful
[HCTR][06:55:57.361][INFO][RK0][main]: Using S3 file system backend.
[HCTR][06:56:00.462][DEBUG][RK0][main]: Successfully write to AWS S3 location: https://s3.us-east-1.amazonaws.com/hugectr-io-test/pipeline_test/dcn_model/_dense_1000.model
[HCTR][06:56:00.467][INFO][RK0][main]: Dumping dense weights to file, successful
[HCTR][06:56:00.467][INFO][RK0][main]: Using S3 file system backend.
[HCTR][06:56:02.839][DEBUG][RK0][main]: Successfully write to AWS S3 location: https://s3.us-east-1.amazonaws.com/hugectr-io-test/pipeline_test/dcn_model/_opt_dense_1000.model
[HCTR][06:56:02.843][INFO][RK0][main]: Dumping dense optimizer states to file, successful
[HCTR][06:56:06.987][INFO][RK0][main]: Finish 1100 iterations with batchsize: 1024 in 63.58s.
[HCTR][06:56:06.988][INFO][RK0][main]: Save the model graph to dcn.json successfully
Inference
Important API used in the following script:
In InferenceParams(), we specify S3 path in the
sparse_model_files
parameter to load trained models from S3.In predict(), we specify the DataSourceParams to read data from S3.
Please note that we are Not supporting reading model graphs from S3 yet. Only models can be read from remote.
%%writefile inference_with_s3.py
import hugectr
from hugectr.inference import InferenceModel, InferenceParams
from hugectr.data import DataSourceParams
import numpy as np
from mpi4py import MPI
# Create a file system configuration for data reading
data_source_params = DataSourceParams(
source = hugectr.FileSystemType_t.S3, # use AWS S3
server = 'us-east-1', # your AWS region
port = 9000, # with be ignored
)
model_config = "dcn.json" # should be in local
inference_params = InferenceParams(
model_name = "dcn",
max_batchsize = 1024,
hit_rate_threshold = 1.0,
dense_model_file = "https://s3.us-east-1.amazonaws.com/hugectr-io-test/pipeline_test/dcn_model/_dense_1000.model", # S3 URL
sparse_model_files = ["https://s3.us-east-1.amazonaws.com/hugectr-io-test/pipeline_test/dcn_model/0_sparse_1000.model"], # S3 URL
deployed_devices = [0],
use_gpu_embedding_cache = True,
cache_size_percentage = 1.0,
i64_input_key = True
)
inference_model = InferenceModel(model_config, inference_params)
pred = inference_model.predict(
10,
"/hugectr-io-test/data/dcn_parquet/file_list_test.txt",
hugectr.DataReaderType_t.Parquet,
hugectr.Check_t.Non,
[39884,39043,17289,7420,20263,3,7120,1543,39884,39043,17289,7420,20263,3,7120,1543,63,63,39884,39043,17289,7420,20263,3,7120,1543],
data_source_params
)
print(pred.shape)
print(pred)
Overwriting inference_with_s3.py
!python inference_with_s3.py
[HCTR][02:48:08.494][INFO][RK0][main]: Global seed is 2188274617
[HCTR][02:48:08.496][INFO][RK0][main]: Device to NUMA mapping:
GPU 0 -> node 0
[HCTR][02:48:10.297][WARNING][RK0][main]: Peer-to-peer access cannot be fully enabled.
[HCTR][02:48:10.297][DEBUG][RK0][main]: [device 0] allocating 0.0000 GB, available 30.7791
[HCTR][02:48:10.297][INFO][RK0][main]: Start all2all warmup
[HCTR][02:48:10.297][INFO][RK0][main]: End all2all warmup
[HCTR][02:48:10.298][INFO][RK0][main]: default_emb_vec_value is not specified using default: 0
====================================================HPS Create====================================================
[HCTR][02:48:10.298][INFO][RK0][main]: Creating HashMap CPU database backend...
[HCTR][02:48:10.298][DEBUG][RK0][main]: Created blank database backend in local memory!
[HCTR][02:48:10.298][INFO][RK0][main]: Volatile DB: initial cache rate = 1
[HCTR][02:48:10.298][INFO][RK0][main]: Volatile DB: cache missed embeddings = 0
[HCTR][02:48:10.298][DEBUG][RK0][main]: Created raw model loader in local memory!
[HCTR][02:48:10.298][INFO][RK0][main]: Using S3 file system backend.
[HCTR][02:48:21.335][INFO][RK0][main]: Table: hps_et.dcn.sparse_embedding1; cached 252900 / 252900 embeddings in volatile database (HashMapBackend); load: 252900 / 18446744073709551615 (0.00%).
[HCTR][02:48:21.335][DEBUG][RK0][main]: Real-time subscribers created!
[HCTR][02:48:21.335][INFO][RK0][main]: Creating embedding cache in device 0.
[HCTR][02:48:21.340][INFO][RK0][main]: Model name: dcn
[HCTR][02:48:21.340][INFO][RK0][main]: Max batch size: 1024
[HCTR][02:48:21.340][INFO][RK0][main]: Number of embedding tables: 1
[HCTR][02:48:21.340][INFO][RK0][main]: Use GPU embedding cache: True, cache size percentage: 1.000000
[HCTR][02:48:21.340][INFO][RK0][main]: Use static table: False
[HCTR][02:48:21.340][INFO][RK0][main]: Use I64 input key: True
[HCTR][02:48:21.340][INFO][RK0][main]: Configured cache hit rate threshold: 1.000000
[HCTR][02:48:21.340][INFO][RK0][main]: The size of thread pool: 80
[HCTR][02:48:21.340][INFO][RK0][main]: The size of worker memory pool: 2
[HCTR][02:48:21.340][INFO][RK0][main]: The size of refresh memory pool: 1
[HCTR][02:48:21.340][INFO][RK0][main]: The refresh percentage : 0.000000
[HCTR][02:48:21.351][INFO][RK0][main]: Model name: dcn
[HCTR][02:48:21.351][INFO][RK0][main]: Use mixed precision: False
[HCTR][02:48:21.351][INFO][RK0][main]: Use cuda graph: True
[HCTR][02:48:21.351][INFO][RK0][main]: Max batchsize: 1024
[HCTR][02:48:21.351][INFO][RK0][main]: Use I64 input key: True
[HCTR][02:48:21.351][INFO][RK0][main]: start create embedding for inference
[HCTR][02:48:21.351][INFO][RK0][main]: sparse_input name data1
[HCTR][02:48:21.351][INFO][RK0][main]: create embedding for inference success
[HCTR][02:48:21.352][DEBUG][RK0][main]: [device 0] allocating 0.0033 GB, available 30.4958
[HCTR][02:48:21.352][INFO][RK0][main]: No projection_dim given, degrade to DCNv1
[HCTR][02:48:21.352][WARNING][RK0][main]: using multi-cross v1
[HCTR][02:48:21.352][INFO][RK0][main]: Inference stage skip BinaryCrossEntropyLoss layer, replaced by Sigmoid layer
[HCTR][02:48:21.353][DEBUG][RK0][main]: [device 0] allocating 0.0423 GB, available 30.4490
[HCTR][02:48:22.133][INFO][RK0][main]: Using S3 file system backend.
[HCTR][02:48:29.008][DEBUG][RK0][main]: [device 0] allocating 0.0001 GB, available 30.4470
[HCTR][02:48:29.009][INFO][RK0][main]: Create inference data reader on 1 GPU(s)
[HCTR][02:48:29.009][INFO][RK0][main]: num of DataReader workers: 1
[HCTR][02:48:29.009][DEBUG][RK0][main]: [device 0] allocating 0.0014 GB, available 30.4451
[HCTR][02:48:29.010][DEBUG][RK0][main]: [device 0] allocating 0.0000 GB, available 30.4451
[HCTR][02:48:29.010][INFO][RK0][main]: Using S3 file system backend.
[HCTR][02:48:31.017][INFO][RK0][main]: Vocabulary size: 397821
████████████████████████████████████████▏ 100.0% [ 10/ 10 | 7.5 Hz | 1s<0s] 0m
[HCTR][02:48:32.354][INFO][RK0][main]: Inference time for 10 batches: 1.33394
(10240, 1)
[[0.47839856]
[0.4756918 ]
[0.47329405]
...
[0.46896443]
[0.49150574]
[0.45769793]]
Training a DCN model with Google Cloud Storage
Before you start: Please note that GCS SDK are NOT preinstalled in the NGC docker. To use GCS related functionalites, please do the following steps to customize the building of HugeCTR:
git clone https://github.com/NVIDIA/HugeCTR.git
cd HugeCTR
git submodule update –init –recursive
mkdir -p build && cd build
cmake -DCMAKE_BUILD_TYPE=Release -DSM=70 -DENABLE_GCS=ON … #ENABLE_GCS option will install GCS SDKs for you.
make -j && make install
Data preparation
Create file_list.txt and file_list_test.txt
:
!mkdir -p /hugectr-io-test/data/dcn_parquet/train
!mkdir -p /hugectr-io-test/data/dcn_parquet/val
%%writefile /hugectr-io-test/data/dcn_parquet/file_list.txt
16
gs://hugectr-io-test/data/dcn_parquet/train/gen_0.parquet
gs://hugectr-io-test/data/dcn_parquet/train/gen_1.parquet
gs://hugectr-io-test/data/dcn_parquet/train/gen_2.parquet
gs://hugectr-io-test/data/dcn_parquet/train/gen_3.parquet
gs://hugectr-io-test/data/dcn_parquet/train/gen_4.parquet
gs://hugectr-io-test/data/dcn_parquet/train/gen_5.parquet
gs://hugectr-io-test/data/dcn_parquet/train/gen_6.parquet
gs://hugectr-io-test/data/dcn_parquet/train/gen_7.parquet
gs://hugectr-io-test/data/dcn_parquet/train/gen_8.parquet
gs://hugectr-io-test/data/dcn_parquet/train/gen_9.parquet
gs://hugectr-io-test/data/dcn_parquet/train/gen_10.parquet
gs://hugectr-io-test/data/dcn_parquet/train/gen_11.parquet
gs://hugectr-io-test/data/dcn_parquet/train/gen_12.parquet
gs://hugectr-io-test/data/dcn_parquet/train/gen_13.parquet
gs://hugectr-io-test/data/dcn_parquet/train/gen_14.parquet
gs://hugectr-io-test/data/dcn_parquet/train/gen_15.parquet
Overwriting /hugectr-io-test/data/dcn_parquet/file_list.txt
%%writefile /hugectr-io-test/data/dcn_parquet/file_list_test.txt
4
gs://hugectr-io-test/data/dcn_parquet/val/gen_0.parquet
gs://hugectr-io-test/data/dcn_parquet/val/gen_1.parquet
gs://hugectr-io-test/data/dcn_parquet/val/gen_2.parquet
gs://hugectr-io-test/data/dcn_parquet/val/gen_3.parquet
Overwriting /hugectr-io-test/data/dcn_parquet/file_list_test.txt
%%writefile /hugectr-io-test/data/dcn_parquet/train/_metadata.json
{ "file_stats": [{"file_name": "gs://hugectr-io-test/data/dcn_parquet/train/gen_0.parquet", "num_rows":40960}, {"file_name": "gs://hugectr-io-test/data/dcn_parquet/train/gen_1.parquet", "num_rows":40960},
{"file_name": "gs://hugectr-io-test/data/dcn_parquet/train/gen_2.parquet", "num_rows":40960}, {"file_name": "gs://hugectr-io-test/data/dcn_parquet/train/gen_3.parquet", "num_rows":40960},
{"file_name": "gs://hugectr-io-test/data/dcn_parquet/train/gen_4.parquet", "num_rows":40960}, {"file_name": "gs://hugectr-io-test/data/dcn_parquet/train/gen_5.parquet", "num_rows":40960},
{"file_name": "gs://hugectr-io-test/data/dcn_parquet/train/gen_6.parquet", "num_rows":40960}, {"file_name": "gs://hugectr-io-test/data/dcn_parquet/train/gen_7.parquet", "num_rows":40960},
{"file_name": "gs://hugectr-io-test/data/dcn_parquet/train/gen_8.parquet", "num_rows":40960}, {"file_name": "gs://hugectr-io-test/data/dcn_parquet/train/gen_9.parquet", "num_rows":40960},
{"file_name": "gs://hugectr-io-test/data/dcn_parquet/train/gen_10.parquet", "num_rows":40960}, {"file_name": "gs://hugectr-io-test/data/dcn_parquet/train/gen_11.parquet", "num_rows":40960},
{"file_name": "gs://hugectr-io-test/data/dcn_parquet/train/gen_12.parquet", "num_rows":40960}, {"file_name": "gs://hugectr-io-test/data/dcn_parquet/train/gen_13.parquet", "num_rows":40960},
{"file_name": "gs://hugectr-io-test/data/dcn_parquet/train/gen_14.parquet", "num_rows":40960}, {"file_name": "gs://hugectr-io-test/data/dcn_parquet/train/gen_15.parquet", "num_rows":40960}],
"labels": [{"col_name": "label0", "index":0} ],
"conts": [{"col_name": "C1", "index":1}, {"col_name": "C2", "index":2}, {"col_name": "C3", "index":3}, {"col_name": "C4", "index":4}, {"col_name": "C5", "index":5}, {"col_name": "C6", "index":6},
{"col_name": "C7", "index":7}, {"col_name": "C8", "index":8}, {"col_name": "C9", "index":9}, {"col_name": "C10", "index":10}, {"col_name": "C11", "index":11}, {"col_name": "C12", "index":12},
{"col_name": "C13", "index":13} ],
"cats": [{"col_name": "C14", "index":14}, {"col_name": "C15", "index":15}, {"col_name": "C16", "index":16}, {"col_name": "C17", "index":17}, {"col_name": "C18", "index":18},
{"col_name": "C19", "index":19}, {"col_name": "C20", "index":20}, {"col_name": "C21", "index":21}, {"col_name": "C22", "index":22}, {"col_name": "C23", "index":23},
{"col_name": "C24", "index":24}, {"col_name": "C25", "index":25}, {"col_name": "C26", "index":26}, {"col_name": "C27", "index":27}, {"col_name": "C28", "index":28},
{"col_name": "C29", "index":29}, {"col_name": "C30", "index":30}, {"col_name": "C31", "index":31}, {"col_name": "C32", "index":32}, {"col_name": "C33", "index":33},
{"col_name": "C34", "index":34}, {"col_name": "C35", "index":35}, {"col_name": "C36", "index":36}, {"col_name": "C37", "index":37}, {"col_name": "C38", "index":38}, {"col_name": "C39", "index":39} ] }
Overwriting /hugectr-io-test/data/dcn_parquet/train/_metadata.json
%%writefile /hugectr-io-test/data/dcn_parquet/val/_metadata.json
{ "file_stats": [{"file_name": "gs://hugectr-io-test/data/dcn_parquet/val/gen_0.parquet", "num_rows":40960},
{"file_name": "gs://hugectr-io-test/data/dcn_parquet/val/gen_1.parquet", "num_rows":40960},
{"file_name": "gs://hugectr-io-test/data/dcn_parquet/val/gen_2.parquet", "num_rows":40960},
{"file_name": "gs://hugectr-io-test/data/dcn_parquet/val/gen_3.parquet", "num_rows":40960}],
"labels": [{"col_name": "label0", "index":0} ],
"conts": [{"col_name": "C1", "index":1}, {"col_name": "C2", "index":2}, {"col_name": "C3", "index":3}, {"col_name": "C4", "index":4}, {"col_name": "C5", "index":5}, {"col_name": "C6", "index":6},
{"col_name": "C7", "index":7}, {"col_name": "C8", "index":8}, {"col_name": "C9", "index":9}, {"col_name": "C10", "index":10}, {"col_name": "C11", "index":11}, {"col_name": "C12", "index":12},
{"col_name": "C13", "index":13} ],
"cats": [{"col_name": "C14", "index":14}, {"col_name": "C15", "index":15}, {"col_name": "C16", "index":16}, {"col_name": "C17", "index":17}, {"col_name": "C18", "index":18},
{"col_name": "C19", "index":19}, {"col_name": "C20", "index":20}, {"col_name": "C21", "index":21}, {"col_name": "C22", "index":22}, {"col_name": "C23", "index":23},
{"col_name": "C24", "index":24}, {"col_name": "C25", "index":25}, {"col_name": "C26", "index":26}, {"col_name": "C27", "index":27}, {"col_name": "C28", "index":28},
{"col_name": "C29", "index":29}, {"col_name": "C30", "index":30}, {"col_name": "C31", "index":31}, {"col_name": "C32", "index":32}, {"col_name": "C33", "index":33},
{"col_name": "C34", "index":34}, {"col_name": "C35", "index":35}, {"col_name": "C36", "index":36}, {"col_name": "C37", "index":37}, {"col_name": "C38", "index":38}, {"col_name": "C39", "index":39} ] }
Overwriting /hugectr-io-test/data/dcn_parquet/val/_metadata.json
Training
Important APIs used in the following script:
We use the DataSourceParams to define the remote file system to read data from, in this case, GCS.
In DataReaderParams, we specify the
DataSourceParams
.In fit() method, we specify GCS path in the
snapshot_prefix
parameters to dump trained models to GCS.
#You need to set the GCP credentials environmental variable to access the GCS.
%env GOOGLE_APPLICATION_CREDENTIALS=/path/to/your/gcs_key.json
env: GOOGLE_APPLICATION_CREDENTIALS=/path/to/your/gcs_key.json
%%writefile train_with_gcs.py
import hugectr
from mpi4py import MPI
from hugectr.data import DataSourceParams
# Create a file system configuration for data reading
data_source_params = DataSourceParams(
source = hugectr.FileSystemType_t.GCS, #use Google Cloud Storage
server = 'storage.googleapis.com', #your endpoint override, usually storage.googleapis.com or storage.google.cloud.com
port = 9000, #with be ignored
)
solver = hugectr.CreateSolver(
max_eval_batches=1280,
batchsize_eval=1024,
batchsize=1024,
lr=0.001,
vvgpu=[[0]],
i64_input_key=True,
repeat_dataset=True,
)
reader = hugectr.DataReaderParams(
data_reader_type=hugectr.DataReaderType_t.Parquet,
source=["/hugectr-io-test/data/dcn_parquet/file_list.txt"],
eval_source="/hugectr-io-test/data/dcn_parquet/file_list_test.txt",
slot_size_array=[39884,39043,17289,7420,20263,3,7120,1543,39884,39043,17289,7420,20263,3,7120,1543,63,63,39884,39043,17289,7420,20263,3,7120,1543],
data_source_params=data_source_params, # Using the GCS configurations
check_type=hugectr.Check_t.Non,
)
optimizer = hugectr.CreateOptimizer(optimizer_type=hugectr.Optimizer_t.SGD)
model = hugectr.Model(solver, reader, optimizer)
model.add(
hugectr.Input(
label_dim=1,
label_name="label",
dense_dim=13,
dense_name="dense",
data_reader_sparse_param_array=[
hugectr.DataReaderSparseParam("data1", 1, True, 26)
],
)
)
model.add(
hugectr.SparseEmbedding(
embedding_type=hugectr.Embedding_t.DistributedSlotSparseEmbeddingHash,
workspace_size_per_gpu_in_mb=150,
embedding_vec_size=16,
combiner="sum",
sparse_embedding_name="sparse_embedding1",
bottom_name="data1",
optimizer=optimizer,
)
)
model.add(
hugectr.DenseLayer(
layer_type=hugectr.Layer_t.Reshape,
bottom_names=["sparse_embedding1"],
top_names=["reshape1"],
leading_dim=416,
)
)
model.add(
hugectr.DenseLayer(
layer_type=hugectr.Layer_t.Concat, bottom_names=["reshape1", "dense"], top_names=["concat1"]
)
)
model.add(
hugectr.DenseLayer(
layer_type=hugectr.Layer_t.Slice,
bottom_names=["concat1"],
top_names=["slice11", "slice12"],
ranges=[(0, 429), (0, 429)],
)
)
model.add(
hugectr.DenseLayer(
layer_type=hugectr.Layer_t.MultiCross,
bottom_names=["slice11"],
top_names=["multicross1"],
num_layers=6,
)
)
model.add(
hugectr.DenseLayer(
layer_type=hugectr.Layer_t.InnerProduct,
bottom_names=["slice12"],
top_names=["fc1"],
num_output=1024,
)
)
model.add(
hugectr.DenseLayer(layer_type=hugectr.Layer_t.ReLU, bottom_names=["fc1"], top_names=["relu1"])
)
model.add(
hugectr.DenseLayer(
layer_type=hugectr.Layer_t.Dropout,
bottom_names=["relu1"],
top_names=["dropout1"],
dropout_rate=0.5,
)
)
model.add(
hugectr.DenseLayer(
layer_type=hugectr.Layer_t.Concat,
bottom_names=["dropout1", "multicross1"],
top_names=["concat2"],
)
)
model.add(
hugectr.DenseLayer(
layer_type=hugectr.Layer_t.InnerProduct,
bottom_names=["concat2"],
top_names=["fc2"],
num_output=1,
)
)
model.add(
hugectr.DenseLayer(
layer_type=hugectr.Layer_t.BinaryCrossEntropyLoss,
bottom_names=["fc2", "label"],
top_names=["loss"],
)
)
model.compile()
model.summary()
model.fit(max_iter = 1100, display = 100, eval_interval = 500, snapshot = 1000, snapshot_prefix = "https://storage.googleapis.com/hugectr-io-test/pipeline_test/")
model.graph_to_json(graph_config_file = "dcn.json")
Overwriting train_with_gcs.py
!python train_with_gcs.py
HugeCTR Version: 4.1
====================================================Model Init=====================================================
[HCTR][03:15:35.248][WARNING][RK0][main]: The model name is not specified when creating the solver.
[HCTR][03:15:35.248][INFO][RK0][main]: Global seed is 1008636636
[HCTR][03:15:35.251][INFO][RK0][main]: Device to NUMA mapping:
GPU 0 -> node 0
[HCTR][03:15:37.306][WARNING][RK0][main]: Peer-to-peer access cannot be fully enabled.
[HCTR][03:15:37.306][INFO][RK0][main]: Start all2all warmup
[HCTR][03:15:37.306][INFO][RK0][main]: End all2all warmup
[HCTR][03:15:37.307][INFO][RK0][main]: Using All-reduce algorithm: NCCL
[HCTR][03:15:37.308][INFO][RK0][main]: Device 0: Tesla V100-SXM2-32GB
[HCTR][03:15:37.308][INFO][RK0][main]: num of DataReader workers for train: 1
[HCTR][03:15:37.308][INFO][RK0][main]: num of DataReader workers for eval: 1
[HCTR][03:15:37.309][INFO][RK0][main]: Using GCS file system backend.
[HCTR][03:15:37.323][INFO][RK0][main]: Using GCS file system backend.
[HCTR][03:15:37.328][INFO][RK0][main]: Vocabulary size: 397821
[HCTR][03:15:37.329][INFO][RK0][main]: max_vocabulary_size_per_gpu_=2457600
[HCTR][03:15:37.331][INFO][RK0][main]: Graph analysis to resolve tensor dependency
[HCTR][03:15:37.331][WARNING][RK0][main]: using multi-cross v1
[HCTR][03:15:37.331][WARNING][RK0][main]: using multi-cross v1
===================================================Model Compile===================================================
[HCTR][03:15:39.005][INFO][RK0][main]: gpu0 start to init embedding
[HCTR][03:15:39.006][INFO][RK0][main]: gpu0 init embedding done
[HCTR][03:15:39.008][INFO][RK0][main]: Starting AUC NCCL warm-up
[HCTR][03:15:39.010][INFO][RK0][main]: Warm-up done
===================================================Model Summary===================================================
[HCTR][03:15:39.010][INFO][RK0][main]: Model structure on each GPU
Label Dense Sparse
label dense data1
(1024,1) (1024,13)
——————————————————————————————————————————————————————————————————————————————————————————————————————————————————
Layer Type Input Name Output Name Output Shape
——————————————————————————————————————————————————————————————————————————————————————————————————————————————————
DistributedSlotSparseEmbeddingHash data1 sparse_embedding1 (1024,26,16)
------------------------------------------------------------------------------------------------------------------
Reshape sparse_embedding1 reshape1 (1024,416)
------------------------------------------------------------------------------------------------------------------
Concat reshape1 concat1 (1024,429)
dense
------------------------------------------------------------------------------------------------------------------
Slice concat1 slice11 (1024,429)
slice12 (1024,429)
------------------------------------------------------------------------------------------------------------------
MultiCross slice11 multicross1 (1024,429)
------------------------------------------------------------------------------------------------------------------
InnerProduct slice12 fc1 (1024,1024)
------------------------------------------------------------------------------------------------------------------
ReLU fc1 relu1 (1024,1024)
------------------------------------------------------------------------------------------------------------------
Dropout relu1 dropout1 (1024,1024)
------------------------------------------------------------------------------------------------------------------
Concat dropout1 concat2 (1024,1453)
multicross1
------------------------------------------------------------------------------------------------------------------
InnerProduct concat2 fc2 (1024,1)
------------------------------------------------------------------------------------------------------------------
BinaryCrossEntropyLoss fc2 loss
label
------------------------------------------------------------------------------------------------------------------
=====================================================Model Fit=====================================================
[HCTR][03:15:39.011][INFO][RK0][main]: Use non-epoch mode with number of iterations: 1100
[HCTR][03:15:39.011][INFO][RK0][main]: Training batchsize: 1024, evaluation batchsize: 1024
[HCTR][03:15:39.011][INFO][RK0][main]: Evaluation interval: 500, snapshot interval: 1000
[HCTR][03:15:39.011][INFO][RK0][main]: Dense network trainable: True
[HCTR][03:15:39.011][INFO][RK0][main]: Sparse embedding sparse_embedding1 trainable: True
[HCTR][03:15:39.011][INFO][RK0][main]: Use mixed precision: False, scaler: 1.000000, use cuda graph: True
[HCTR][03:15:39.011][INFO][RK0][main]: lr: 0.001000, warmup_steps: 1, end_lr: 0.000000
[HCTR][03:15:39.011][INFO][RK0][main]: decay_start: 0, decay_steps: 1, decay_power: 2.000000
[HCTR][03:15:39.011][INFO][RK0][main]: Training source file: /hugectr-io-test/data/dcn_parquet/file_list.txt
[HCTR][03:15:39.011][INFO][RK0][main]: Evaluation source file: /hugectr-io-test/data/dcn_parquet/file_list_test.txt
[HCTR][03:15:40.236][INFO][RK0][main]: Iter: 100 Time(100 iters): 1.22452s Loss: 0.786299 lr:0.001
[HCTR][03:15:41.872][INFO][RK0][main]: Iter: 200 Time(100 iters): 1.6347s Loss: 0.738846 lr:0.001
[HCTR][03:15:43.102][INFO][RK0][main]: Iter: 300 Time(100 iters): 1.22938s Loss: 0.711017 lr:0.001
[HCTR][03:15:44.736][INFO][RK0][main]: Iter: 400 Time(100 iters): 1.63355s Loss: 0.708317 lr:0.001
[HCTR][03:15:45.850][INFO][RK0][main]: Iter: 500 Time(100 iters): 1.11226s Loss: 0.697101 lr:0.001
[HCTR][03:15:59.880][INFO][RK0][main]: Evaluation, AUC: 0.501301
[HCTR][03:15:59.880][INFO][RK0][main]: Eval Time for 1280 iters: 14.0298s
[HCTR][03:16:01.456][INFO][RK0][main]: Iter: 600 Time(100 iters): 15.6054s Loss: 0.698077 lr:0.001
[HCTR][03:16:02.201][INFO][RK0][main]: Iter: 700 Time(100 iters): 0.744573s Loss: 0.697804 lr:0.001
[HCTR][03:16:03.244][INFO][RK0][main]: Iter: 800 Time(100 iters): 1.04207s Loss: 0.695543 lr:0.001
[HCTR][03:16:04.007][INFO][RK0][main]: Iter: 900 Time(100 iters): 0.761465s Loss: 0.695323 lr:0.001
[HCTR][03:16:05.289][INFO][RK0][main]: Iter: 1000 Time(100 iters): 1.28151s Loss: 0.695319 lr:0.001
[HCTR][03:16:17.647][INFO][RK0][main]: Evaluation, AUC: 0.501347
[HCTR][03:16:17.647][INFO][RK0][main]: Eval Time for 1280 iters: 12.3576s
[HCTR][03:16:17.647][INFO][RK0][main]: Using GCS file system backend.
[HCTR][03:16:17.664][INFO][RK0][main]: Rank0: Write hash table to file
[HCTR][03:16:18.623][DEBUG][RK0][main]: Successfully write to GCS location: https://storage.googleapis.com/hugectr-io-test/pipeline_test/0_sparse_1000.model/key
[HCTR][03:16:20.289][DEBUG][RK0][main]: Successfully write to GCS location: https://storage.googleapis.com/hugectr-io-test/pipeline_test/0_sparse_1000.model/emb_vector
[HCTR][03:16:20.294][INFO][RK0][main]: Dumping sparse weights to files, successful
[HCTR][03:16:20.294][INFO][RK0][main]: Dumping sparse optimzer states to files, successful
[HCTR][03:16:20.294][INFO][RK0][main]: Using GCS file system backend.
[HCTR][03:16:21.254][DEBUG][RK0][main]: Successfully write to GCS location: https://storage.googleapis.com/hugectr-io-test/pipeline_test/_dense_1000.model
[HCTR][03:16:21.255][INFO][RK0][main]: Dumping dense weights to file, successful
[HCTR][03:16:21.255][INFO][RK0][main]: Using GCS file system backend.
[HCTR][03:16:21.803][DEBUG][RK0][main]: Successfully write to GCS location: https://storage.googleapis.com/hugectr-io-test/pipeline_test/_opt_dense_1000.model
[HCTR][03:16:21.804][INFO][RK0][main]: Dumping dense optimizer states to file, successful
[HCTR][03:16:22.606][INFO][RK0][main]: Finish 1100 iterations with batchsize: 1024 in 43.60s.
[HCTR][03:16:22.607][INFO][RK0][main]: Save the model graph to dcn.json successfully
Inference
Data preparation**
Please note that we are Not supporting reading model graphs and dense models from GCS yet. Only Sparse models can be read from remote.**
%%writefile inference_with_gcs.py
import hugectr
from hugectr.inference import InferenceModel, InferenceParams
from hugectr.data import DataSourceParams
import numpy as np
from mpi4py import MPI
# Create a file system configuration for data reading
data_source_params = DataSourceParams(
source = hugectr.FileSystemType_t.GCS, # use GCS
server = 'storage.googleapis.com', # your GCS endpoint override
port = 9000, # with be ignored
)
model_config = "dcn.json" # should be in local
inference_params = InferenceParams(
model_name = "dcn",
max_batchsize = 1024,
hit_rate_threshold = 1.0,
dense_model_file = "./_dense_10000.model", # should be in local
sparse_model_files = ["https://storage.googleapis.com/hugectr-io-test/pipeline_test/0_sparse_1000.model"], # GCS URL
deployed_devices = [0],
use_gpu_embedding_cache = True,
cache_size_percentage = 1.0,
i64_input_key = True
)
inference_model = InferenceModel(model_config, inference_params)
pred = inference_model.predict(
10,
"/hugectr-io-test/data/dcn_parquet/file_list_test.txt",
hugectr.DataReaderType_t.Parquet,
hugectr.Check_t.Non,
[39884,39043,17289,7420,20263,3,7120,1543,39884,39043,17289,7420,20263,3,7120,1543,63,63,39884,39043,17289,7420,20263,3,7120,1543],
data_source_params
)
print(pred.shape)
print(pred)
Overwriting inference_with_gcs.py
!python inference_with_gcs.py
[HCTR][09:30:37.214][INFO][RK0][main]: Global seed is 1015829727
[HCTR][09:30:37.217][INFO][RK0][main]: Device to NUMA mapping:
GPU 0 -> node 0
[HCTR][09:30:39.061][WARNING][RK0][main]: Peer-to-peer access cannot be fully enabled.
[HCTR][09:30:39.061][DEBUG][RK0][main]: [device 0] allocating 0.0000 GB, available 30.7830
[HCTR][09:30:39.061][INFO][RK0][main]: Start all2all warmup
[HCTR][09:30:39.061][INFO][RK0][main]: End all2all warmup
[HCTR][09:30:39.062][INFO][RK0][main]: default_emb_vec_value is not specified using default: 0
====================================================HPS Create====================================================
[HCTR][09:30:39.062][INFO][RK0][main]: Creating HashMap CPU database backend...
[HCTR][09:30:39.062][DEBUG][RK0][main]: Created blank database backend in local memory!
[HCTR][09:30:39.062][INFO][RK0][main]: Volatile DB: initial cache rate = 1
[HCTR][09:30:39.062][INFO][RK0][main]: Volatile DB: cache missed embeddings = 0
[HCTR][09:30:39.062][DEBUG][RK0][main]: Created raw model loader in local memory!
[HCTR][09:30:39.063][INFO][RK0][main]: Using GCS file system backend.
[HCTR][09:30:40.357][INFO][RK0][main]: Table: hps_et.dcn.sparse_embedding1; cached 252900 / 252900 embeddings in volatile database (HashMapBackend); load: 252900 / 18446744073709551615 (0.00%).
[HCTR][09:30:40.357][DEBUG][RK0][main]: Real-time subscribers created!
[HCTR][09:30:40.357][INFO][RK0][main]: Creating embedding cache in device 0.
[HCTR][09:30:40.362][INFO][RK0][main]: Model name: dcn
[HCTR][09:30:40.362][INFO][RK0][main]: Max batch size: 1024
[HCTR][09:30:40.362][INFO][RK0][main]: Number of embedding tables: 1
[HCTR][09:30:40.362][INFO][RK0][main]: Use GPU embedding cache: True, cache size percentage: 1.000000
[HCTR][09:30:40.362][INFO][RK0][main]: Use static table: False
[HCTR][09:30:40.362][INFO][RK0][main]: Use I64 input key: True
[HCTR][09:30:40.362][INFO][RK0][main]: Configured cache hit rate threshold: 1.000000
[HCTR][09:30:40.362][INFO][RK0][main]: The size of thread pool: 80
[HCTR][09:30:40.362][INFO][RK0][main]: The size of worker memory pool: 2
[HCTR][09:30:40.362][INFO][RK0][main]: The size of refresh memory pool: 1
[HCTR][09:30:40.362][INFO][RK0][main]: The refresh percentage : 0.000000
[HCTR][09:30:40.373][INFO][RK0][main]: Model name: dcn
[HCTR][09:30:40.373][INFO][RK0][main]: Use mixed precision: False
[HCTR][09:30:40.373][INFO][RK0][main]: Use cuda graph: True
[HCTR][09:30:40.373][INFO][RK0][main]: Max batchsize: 1024
[HCTR][09:30:40.373][INFO][RK0][main]: Use I64 input key: True
[HCTR][09:30:40.373][INFO][RK0][main]: start create embedding for inference
[HCTR][09:30:40.373][INFO][RK0][main]: sparse_input name data1
[HCTR][09:30:40.373][INFO][RK0][main]: create embedding for inference success
[HCTR][09:30:40.373][DEBUG][RK0][main]: [device 0] allocating 0.0033 GB, available 30.4978
[HCTR][09:30:40.373][INFO][RK0][main]: No projection_dim given, degrade to DCNv1
[HCTR][09:30:40.373][WARNING][RK0][main]: using multi-cross v1
[HCTR][09:30:40.374][INFO][RK0][main]: Inference stage skip BinaryCrossEntropyLoss layer, replaced by Sigmoid layer
[HCTR][09:30:40.374][DEBUG][RK0][main]: [device 0] allocating 0.0423 GB, available 30.4509
[HCTR][09:30:41.157][DEBUG][RK0][main]: [device 0] allocating 0.0001 GB, available 30.4470
[HCTR][09:30:41.157][INFO][RK0][main]: Create inference data reader on 1 GPU(s)
[HCTR][09:30:41.157][INFO][RK0][main]: num of DataReader workers: 1
[HCTR][09:30:41.157][DEBUG][RK0][main]: [device 0] allocating 0.0014 GB, available 30.4451
[HCTR][09:30:41.158][DEBUG][RK0][main]: [device 0] allocating 0.0000 GB, available 30.4451
[HCTR][09:30:41.158][INFO][RK0][main]: Using GCS file system backend.
[HCTR][09:30:41.162][INFO][RK0][main]: Vocabulary size: 397821
████████████████████████████████████████▏ 100.0% [ 10/ 10 | 19.0 Hz | 1s<0s] m
[HCTR][09:30:41.687][INFO][RK0][main]: Inference time for 10 batches: 0.50521
(10240, 1)
[[0.5404203 ]
[0.53341234]
[0.54492587]
...
[0.55712426]
[0.5270296 ]
[0.5275917 ]]