# Copyright 2021 NVIDIA Corporation. All Rights Reserved.
#
# Licensed under the Apache License, Version 2.0 (the "License");
# you may not use this file except in compliance with the License.
# You may obtain a copy of the License at
#
# http://www.apache.org/licenses/LICENSE-2.0
#
# Unless required by applicable law or agreed to in writing, software
# distributed under the License is distributed on an "AS IS" BASIS,
# WITHOUT WARRANTIES OR CONDITIONS OF ANY KIND, either express or implied.
# See the License for the specific language governing permissions and
# limitations under the License.
# ================================
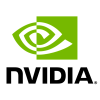
Exporting Ranking Models
In this example notebook we demonstrate how to export (save) NVTabular workflow
and a ranking model
for model deployment with Merlin Systems library.
Learning Objectives:
Export NVTabular workflow for model deployment
Export TensorFlow DLRM model for model deployment
We will follow the steps below:
Prepare the data with NVTabular and export NVTabular workflow
Train a DLRM model with Merlin Models and export the trained model
Importing Libraries
Let’s start with importing the libraries that we’ll use in this notebook.
import os
import nvtabular as nvt
from nvtabular.ops import *
from merlin.models.utils.example_utils import workflow_fit_transform
from merlin.schema.tags import Tags
import merlin.models.tf as mm
from merlin.io.dataset import Dataset
import tensorflow as tf
2022-04-21 09:36:11.187627: I tensorflow/core/platform/cpu_feature_guard.cc:152] This TensorFlow binary is optimized with oneAPI Deep Neural Network Library (oneDNN) to use the following CPU instructions in performance-critical operations: SSE3 SSE4.1 SSE4.2 AVX
To enable them in other operations, rebuild TensorFlow with the appropriate compiler flags.
2022-04-21 09:36:13.394590: I tensorflow/core/common_runtime/gpu/gpu_device.cc:1525] Created device /job:localhost/replica:0/task:0/device:GPU:0 with 16255 MB memory: -> device: 0, name: Tesla V100-SXM2-32GB-LS, pci bus id: 0000:8a:00.0, compute capability: 7.0
Feature Engineering with NVTabular
We use the synthetic train and test datasets generated by mimicking the real Ali-CCP: Alibaba Click and Conversion Prediction dataset to build our recommender system ranking models.
If you would like to use real Ali-CCP dataset instead, you can download the training and test datasets on tianchi.aliyun.com. You can then use get_aliccp() function to curate the raw csv files and save them as the parquet.
from merlin.datasets.synthetic import generate_data
DATA_FOLDER = os.environ.get("DATA_FOLDER", "/workspace/data/")
NUM_ROWS = os.environ.get("NUM_ROWS", 1000000)
train, valid = generate_data("aliccp-raw", int(NUM_ROWS), set_sizes=(0.7, 0.3))
# save the datasets as parquet files
train.to_ddf().to_parquet(os.path.join(DATA_FOLDER, "train"))
valid.to_ddf().to_parquet(os.path.join(DATA_FOLDER, "valid"))
(None,)
Let’s define our input and output paths.
train_path = os.path.join(DATA_FOLDER, "train", "part.0.parquet")
valid_path = os.path.join(DATA_FOLDER, "valid", "part.0.parquet")
output_path = os.path.join(DATA_FOLDER, "processed")
After we execute fit()
and transform()
functions on the raw dataset applying the operators defined in the NVTabular workflow pipeline below, the processed parquet files are saved to output_path
.
%%time
user_id = ["user_id"] >> Categorify() >> TagAsUserID()
item_id = ["item_id"] >> Categorify() >> TagAsItemID()
targets = ["click"] >> AddMetadata(tags=[Tags.BINARY_CLASSIFICATION, "target"])
item_features = ["item_category", "item_shop", "item_brand"] >> Categorify() >> TagAsItemFeatures()
user_features = (
[
"user_shops",
"user_profile",
"user_group",
"user_gender",
"user_age",
"user_consumption_2",
"user_is_occupied",
"user_geography",
"user_intentions",
"user_brands",
"user_categories",
]
>> Categorify()
>> TagAsUserFeatures()
)
outputs = user_id + item_id + item_features + user_features + targets
workflow = nvt.Workflow(outputs)
train_dataset = nvt.Dataset(train_path)
valid_dataset = nvt.Dataset(valid_path)
workflow.fit(train_dataset)
workflow.transform(train_dataset).to_parquet(output_path=output_path + "/train/")
workflow.transform(valid_dataset).to_parquet(output_path=output_path + "/valid/")
/usr/local/lib/python3.8/dist-packages/cudf/core/dataframe.py:1292: UserWarning: The deep parameter is ignored and is only included for pandas compatibility.
warnings.warn(
CPU times: user 2.14 s, sys: 1.66 s, total: 3.8 s
Wall time: 6.01 s
We save NVTabular workflow
model in the current working directory.
workflow.save("workflow")
Let’s check out our saved workflow model folder.
!apt-get update
!apt-get install tree
Get:1 http://archive.ubuntu.com/ubuntu focal InRelease [265 kB]
Get:2 http://security.ubuntu.com/ubuntu focal-security InRelease [114 kB]
Get:3 http://archive.ubuntu.com/ubuntu focal-updates InRelease [114 kB]
Get:4 http://archive.ubuntu.com/ubuntu focal-backports InRelease [108 kB]
Get:5 http://archive.ubuntu.com/ubuntu focal/restricted amd64 Packages [33.4 kB]
Get:6 http://archive.ubuntu.com/ubuntu focal/universe amd64 Packages [11.3 MB]
Get:7 http://archive.ubuntu.com/ubuntu focal/multiverse amd64 Packages [177 kB]
Get:8 http://archive.ubuntu.com/ubuntu focal/main amd64 Packages [1275 kB]
Get:9 http://archive.ubuntu.com/ubuntu focal-updates/multiverse amd64 Packages [30.3 kB]
Get:10 http://archive.ubuntu.com/ubuntu focal-updates/restricted amd64 Packages [1214 kB]
Get:11 http://security.ubuntu.com/ubuntu focal-security/main amd64 Packages [1771 kB]
Get:12 http://archive.ubuntu.com/ubuntu focal-updates/universe amd64 Packages [1153 kB]
Get:13 http://archive.ubuntu.com/ubuntu focal-updates/main amd64 Packages [2186 kB]
Get:14 http://archive.ubuntu.com/ubuntu focal-backports/main amd64 Packages [51.2 kB]
Get:15 http://archive.ubuntu.com/ubuntu focal-backports/universe amd64 Packages [26.0 kB]
Get:16 http://security.ubuntu.com/ubuntu focal-security/restricted amd64 Packages [1139 kB]
Get:17 http://security.ubuntu.com/ubuntu focal-security/multiverse amd64 Packages [25.8 kB]
Get:18 http://security.ubuntu.com/ubuntu focal-security/universe amd64 Packages [870 kB]
Fetched 21.9 MB in 3s (6363 kB/s)
Reading package lists... Done
Reading package lists... Done
Building dependency tree
Reading state information... Done
The following NEW packages will be installed:
tree
0 upgraded, 1 newly installed, 0 to remove and 34 not upgraded.
Need to get 43.0 kB of archives.
After this operation, 115 kB of additional disk space will be used.
Get:1 http://archive.ubuntu.com/ubuntu focal/universe amd64 tree amd64 1.8.0-1 [43.0 kB]
Fetched 43.0 kB in 0s (139 kB/s)
Selecting previously unselected package tree.
(Reading database ... 44765 files and directories currently installed.)
Preparing to unpack .../tree_1.8.0-1_amd64.deb ...
Unpacking tree (1.8.0-1) ...
Setting up tree (1.8.0-1) ...
!tree ./workflow
./workflow
├── categories
│ ├── unique.item_brand.parquet
│ ├── unique.item_category.parquet
│ ├── unique.item_id.parquet
│ ├── unique.item_shop.parquet
│ ├── unique.user_age.parquet
│ ├── unique.user_brands.parquet
│ ├── unique.user_categories.parquet
│ ├── unique.user_consumption_2.parquet
│ ├── unique.user_gender.parquet
│ ├── unique.user_geography.parquet
│ ├── unique.user_group.parquet
│ ├── unique.user_id.parquet
│ ├── unique.user_intentions.parquet
│ ├── unique.user_is_occupied.parquet
│ ├── unique.user_profile.parquet
│ └── unique.user_shops.parquet
├── metadata.json
└── workflow.pkl
1 directory, 18 files
Build and Train a DLRM model
In this example, we build, train, and export a Deep Learning Recommendation Model (DLRM) architecture. To learn more about how to train different deep learning models, how easily transition from one model to another and the seamless integration between data preparation and model training visit 03-Exploring-different-models.ipynb notebook.
NVTabular workflow above exports a schema file, schema.pbtxt, of our processed dataset. To learn more about the schema object, schema file and tags
, you can explore 02-Merlin-Models-and-NVTabular-integration.ipynb.
# define train and valid dataset objects
train = Dataset(os.path.join(output_path, "train", "*.parquet"))
valid = Dataset(os.path.join(output_path, "valid", "*.parquet"))
# define schema object
schema = train.schema
target_column = schema.select_by_tag(Tags.TARGET).column_names[0]
target_column
'click'
model = mm.DLRMModel(
schema,
embedding_dim=64,
bottom_block=mm.MLPBlock([128, 64]),
top_block=mm.MLPBlock([128, 64, 32]),
prediction_tasks=mm.BinaryClassificationTask(target_column, metrics=[tf.keras.metrics.AUC()]),
)
%%time
model.compile("adam", run_eagerly=False)
model.fit(train, validation_data=valid, batch_size=512)
2022-04-21 09:36:37.360581: W tensorflow/python/util/util.cc:368] Sets are not currently considered sequences, but this may change in the future, so consider avoiding using them.
1368/1368 [==============================] - ETA: 0s - auc: 0.5014 - loss: 0.6932 - regularization_loss: 0.0000e+00 - total_loss: 0.6932
2022-04-21 09:37:08.686596: W tensorflow/core/grappler/optimizers/loop_optimizer.cc:907] Skipping loop optimization for Merge node with control input: cond/branch_executed/_13
1368/1368 [==============================] - 33s 17ms/step - auc: 0.5014 - loss: 0.6932 - regularization_loss: 0.0000e+00 - total_loss: 0.6932 - val_auc: 0.4997 - val_loss: 0.6939 - val_regularization_loss: 0.0000e+00 - val_total_loss: 0.6939
CPU times: user 56.3 s, sys: 9.24 s, total: 1min 5s
Wall time: 36.1 s
<keras.callbacks.History at 0x7f19a12fc730>
Save model
The last step of machine learning (ML)/deep learning (DL) pipeline is to deploy the ETL workflow and saved model to production. In the production setting, we want to transform the input data as done during training (ETL). We need to apply the same mean/std for continuous features and use the same categorical mapping to convert the categories to continuous integer before we use the DL model for a prediction. Therefore, we deploy the NVTabular workflow with the Tensorflow model as an ensemble model to Triton Inference using Merlin Systems library very easily. The ensemble model guarantees that the same transformation is applied to the raw inputs.
Let’s save our DLRM model.
model.save("dlrm")
WARNING:absl:Found untraced functions such as sequential_block_8_layer_call_fn, sequential_block_8_layer_call_and_return_conditional_losses, binary_classification_task_layer_call_fn, binary_classification_task_layer_call_and_return_conditional_losses, click/binary_classification_task/output_layer_layer_call_fn while saving (showing 5 of 48). These functions will not be directly callable after loading.
INFO:tensorflow:Assets written to: dlrm/assets
INFO:tensorflow:Assets written to: dlrm/assets
We have NVTabular wokflow and DLRM model exported, now it is time to move on to the next step: model deployment with Merlin Systems.
Deploying the model with Merlin Systems
We trained and exported our ranking model and NVTabular workflow. In the next step, we will learn how to deploy our trained DLRM model into Triton Inference Server with Merlin Systems library. NVIDIA Triton Inference Server (TIS) simplifies the deployment of AI models at scale in production. TIS provides a cloud and edge inferencing solution optimized for both CPUs and GPUs. It supports a number of different machine learning frameworks such as TensorFlow and PyTorch.
For the next step, visit Merlin Systems library and execute Serving-Ranking-Models-With-Merlin-Systems notebook to deploy our saved DLRM and NVTabular workflow models as an ensemble to TIS and obtain prediction results for a qiven request. In doing so, you need to mount the saved DLRM and NVTabular workflow to the inference container following the instructions in the README.md.